کد مقاله | کد نشریه | سال انتشار | مقاله انگلیسی | نسخه تمام متن |
---|---|---|---|---|
727521 | 892763 | 2013 | 7 صفحه PDF | دانلود رایگان |
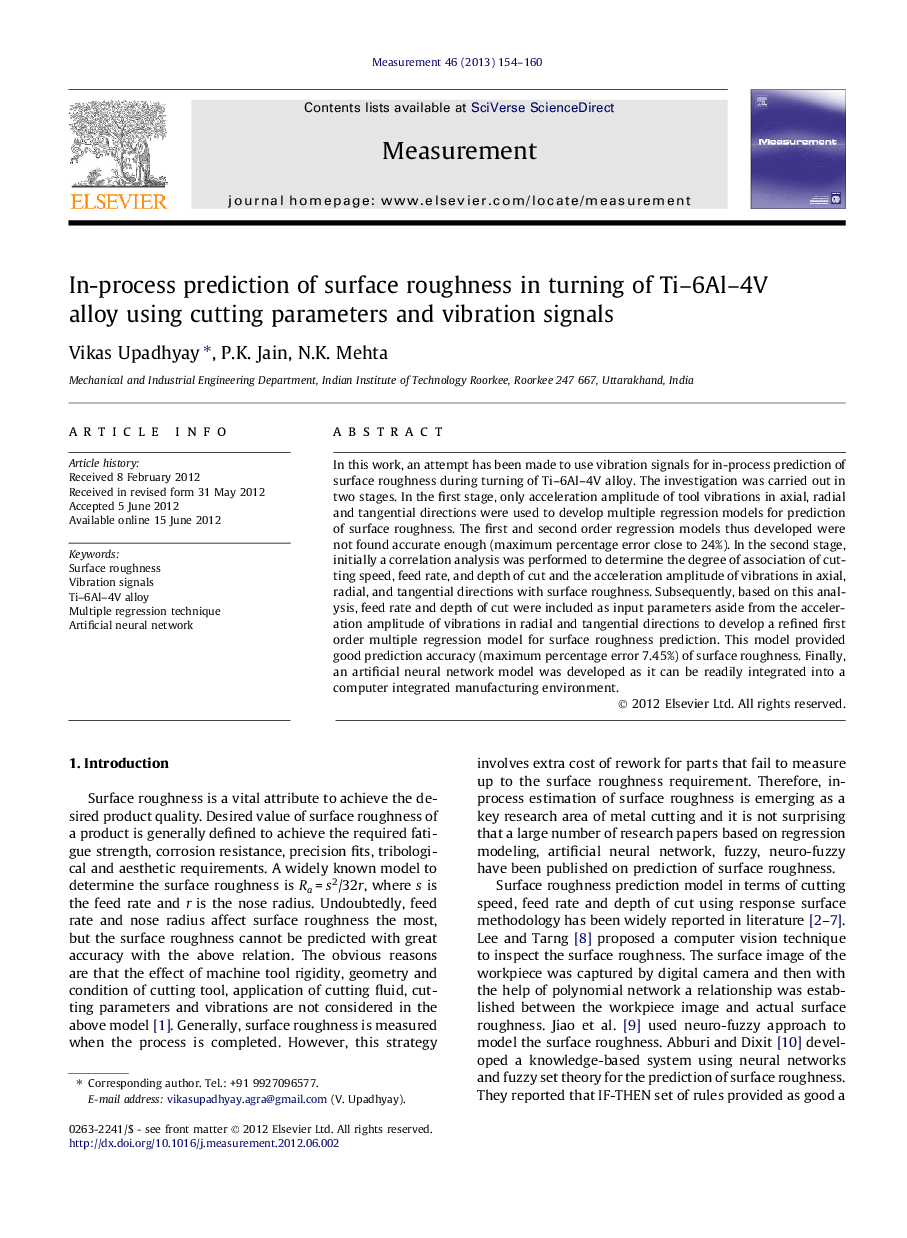
In this work, an attempt has been made to use vibration signals for in-process prediction of surface roughness during turning of Ti–6Al–4V alloy. The investigation was carried out in two stages. In the first stage, only acceleration amplitude of tool vibrations in axial, radial and tangential directions were used to develop multiple regression models for prediction of surface roughness. The first and second order regression models thus developed were not found accurate enough (maximum percentage error close to 24%). In the second stage, initially a correlation analysis was performed to determine the degree of association of cutting speed, feed rate, and depth of cut and the acceleration amplitude of vibrations in axial, radial, and tangential directions with surface roughness. Subsequently, based on this analysis, feed rate and depth of cut were included as input parameters aside from the acceleration amplitude of vibrations in radial and tangential directions to develop a refined first order multiple regression model for surface roughness prediction. This model provided good prediction accuracy (maximum percentage error 7.45%) of surface roughness. Finally, an artificial neural network model was developed as it can be readily integrated into a computer integrated manufacturing environment.
► Vibration signals and cutting parameters can be used for in-process prediction.
► Radial and tangential vibrations, feed rate and depth of cut are used in model.
► Average percentage error in prediction was 4.27% for regression model.
► Average percentage error for ANN model was 4.11%.
Journal: Measurement - Volume 46, Issue 1, January 2013, Pages 154–160