کد مقاله | کد نشریه | سال انتشار | مقاله انگلیسی | نسخه تمام متن |
---|---|---|---|---|
731470 | 893065 | 2012 | 15 صفحه PDF | دانلود رایگان |
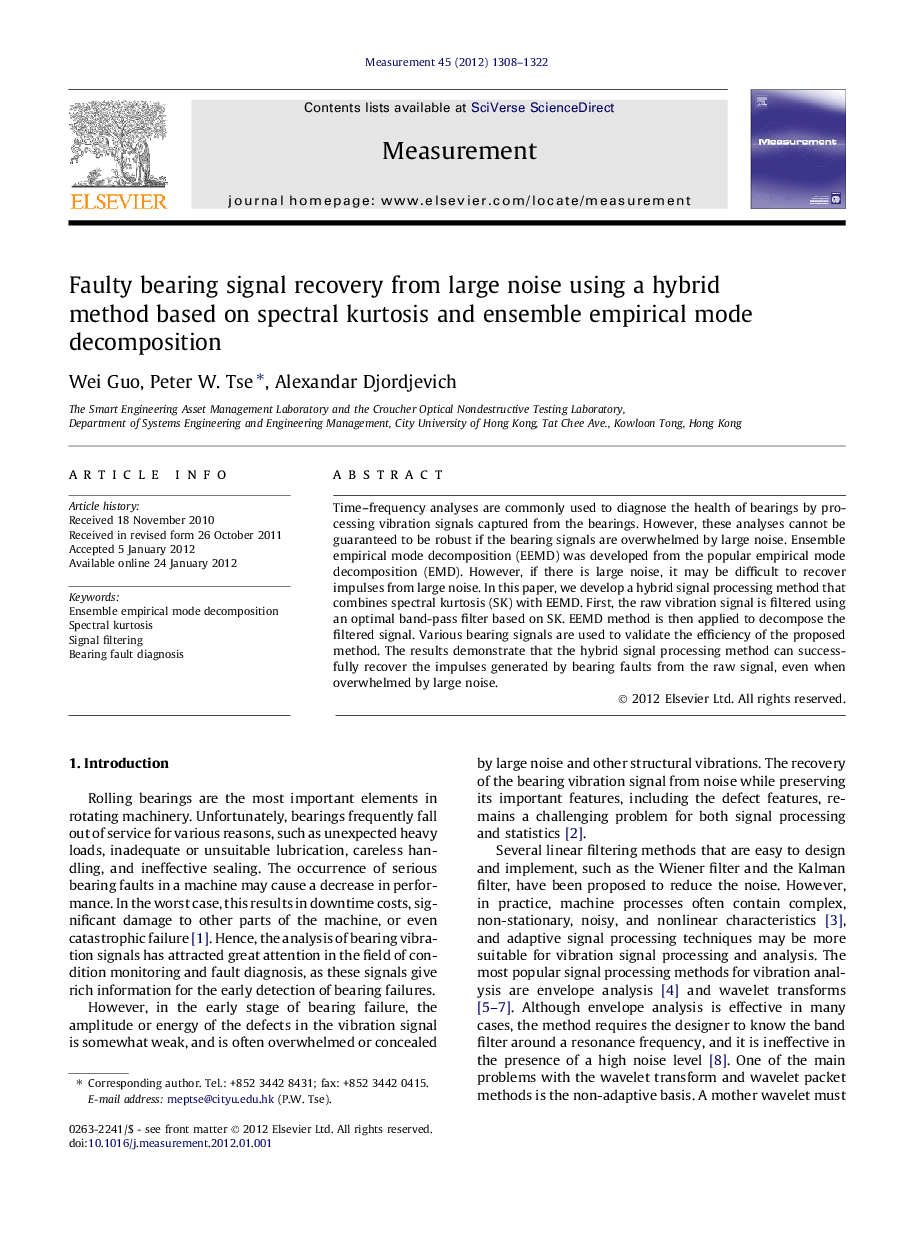
Time–frequency analyses are commonly used to diagnose the health of bearings by processing vibration signals captured from the bearings. However, these analyses cannot be guaranteed to be robust if the bearing signals are overwhelmed by large noise. Ensemble empirical mode decomposition (EEMD) was developed from the popular empirical mode decomposition (EMD). However, if there is large noise, it may be difficult to recover impulses from large noise. In this paper, we develop a hybrid signal processing method that combines spectral kurtosis (SK) with EEMD. First, the raw vibration signal is filtered using an optimal band-pass filter based on SK. EEMD method is then applied to decompose the filtered signal. Various bearing signals are used to validate the efficiency of the proposed method. The results demonstrate that the hybrid signal processing method can successfully recover the impulses generated by bearing faults from the raw signal, even when overwhelmed by large noise.
► We proposed a hybrid signal processing method for faulty bearing signals.
► This method combines spectral kurtosis and ensemble empirical mode decomposition.
► It can recover the bearing signal from the raw vibration signal with large noise.
► The extracted impulsive signal relates to the fault of the inspected bearing.
► The recovered bearing signal can be used for accurate fault diagnosis.
Journal: Measurement - Volume 45, Issue 5, June 2012, Pages 1308–1322