کد مقاله | کد نشریه | سال انتشار | مقاله انگلیسی | نسخه تمام متن |
---|---|---|---|---|
7547343 | 1489730 | 2018 | 19 صفحه PDF | دانلود رایگان |
عنوان انگلیسی مقاله ISI
Nonparametric density estimation for nonnegative data, using symmetrical-based inverse and reciprocal inverse Gaussian kernels through dual transformation
دانلود مقاله + سفارش ترجمه
دانلود مقاله ISI انگلیسی
رایگان برای ایرانیان
کلمات کلیدی
موضوعات مرتبط
مهندسی و علوم پایه
ریاضیات
ریاضیات کاربردی
پیش نمایش صفحه اول مقاله
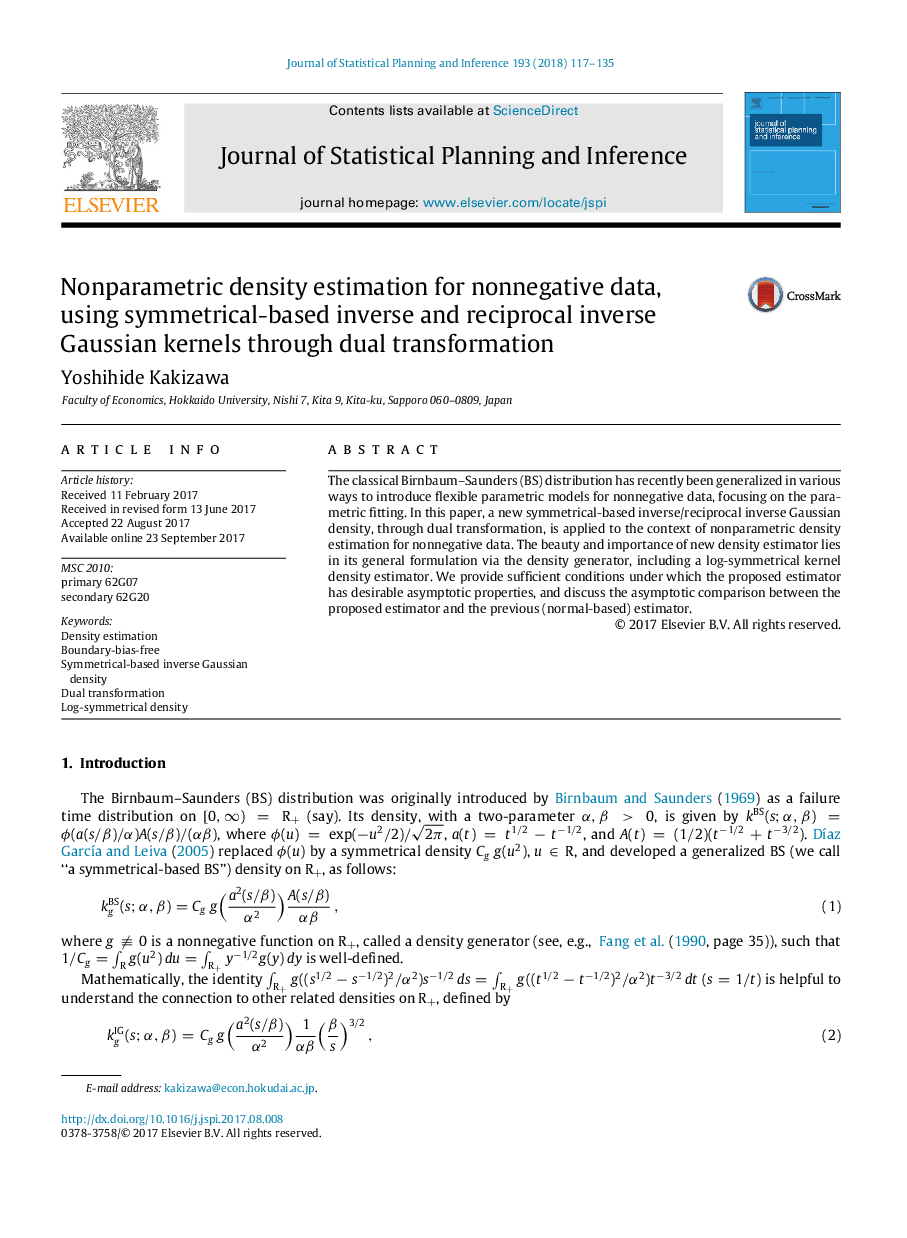
چکیده انگلیسی
The classical Birnbaum-Saunders (BS) distribution has recently been generalized in various ways to introduce flexible parametric models for nonnegative data, focusing on the parametric fitting. In this paper, a new symmetrical-based inverse/reciprocal inverse Gaussian density, through dual transformation, is applied to the context of nonparametric density estimation for nonnegative data. The beauty and importance of new density estimator lies in its general formulation via the density generator, including a log-symmetrical kernel density estimator. We provide sufficient conditions under which the proposed estimator has desirable asymptotic properties, and discuss the asymptotic comparison between the proposed estimator and the previous (normal-based) estimator.
ناشر
Database: Elsevier - ScienceDirect (ساینس دایرکت)
Journal: Journal of Statistical Planning and Inference - Volume 193, February 2018, Pages 117-135
Journal: Journal of Statistical Planning and Inference - Volume 193, February 2018, Pages 117-135
نویسندگان
Yoshihide Kakizawa,