کد مقاله | کد نشریه | سال انتشار | مقاله انگلیسی | نسخه تمام متن |
---|---|---|---|---|
8070996 | 1521390 | 2018 | 12 صفحه PDF | دانلود رایگان |
عنوان انگلیسی مقاله ISI
Production prediction and energy-saving model based on Extreme Learning Machine integrated ISM-AHP: Application in complex chemical processes
دانلود مقاله + سفارش ترجمه
دانلود مقاله ISI انگلیسی
رایگان برای ایرانیان
کلمات کلیدی
موضوعات مرتبط
مهندسی و علوم پایه
مهندسی انرژی
انرژی (عمومی)
پیش نمایش صفحه اول مقاله
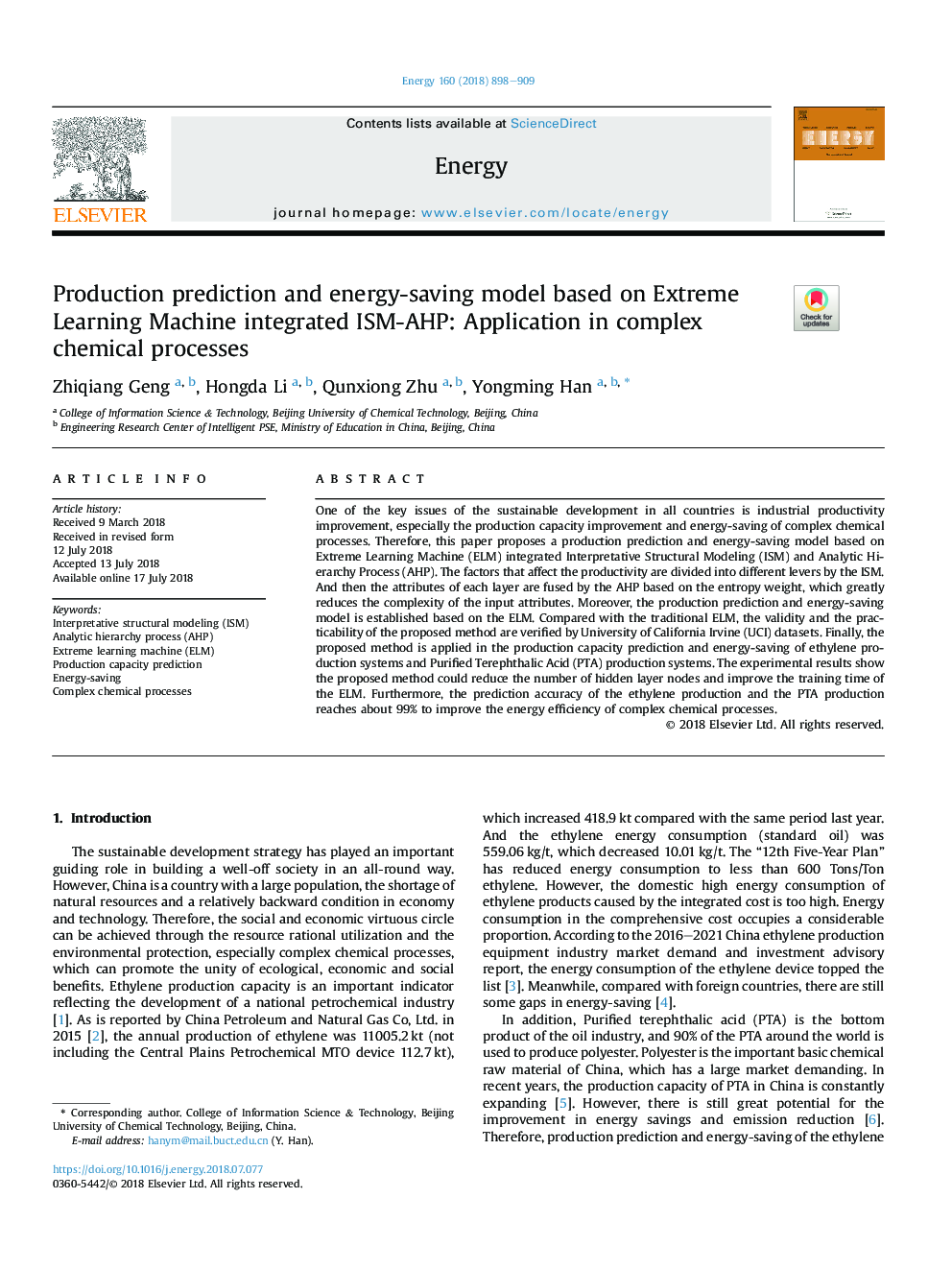
چکیده انگلیسی
One of the key issues of the sustainable development in all countries is industrial productivity improvement, especially the production capacity improvement and energy-saving of complex chemical processes. Therefore, this paper proposes a production prediction and energy-saving model based on Extreme Learning Machine (ELM) integrated Interpretative Structural Modeling (ISM) and Analytic Hierarchy Process (AHP). The factors that affect the productivity are divided into different levers by the ISM. And then the attributes of each layer are fused by the AHP based on the entropy weight, which greatly reduces the complexity of the input attributes. Moreover, the production prediction and energy-saving model is established based on the ELM. Compared with the traditional ELM, the validity and the practicability of the proposed method are verified by University of California Irvine (UCI) datasets. Finally, the proposed method is applied in the production capacity prediction and energy-saving of ethylene production systems and Purified Terephthalic Acid (PTA) production systems. The experimental results show the proposed method could reduce the number of hidden layer nodes and improve the training time of the ELM. Furthermore, the prediction accuracy of the ethylene production and the PTA production reaches about 99% to improve the energy efficiency of complex chemical processes.
ناشر
Database: Elsevier - ScienceDirect (ساینس دایرکت)
Journal: Energy - Volume 160, 1 October 2018, Pages 898-909
Journal: Energy - Volume 160, 1 October 2018, Pages 898-909
نویسندگان
Zhiqiang Geng, Hongda Li, Qunxiong Zhu, Yongming Han,