کد مقاله | کد نشریه | سال انتشار | مقاله انگلیسی | نسخه تمام متن |
---|---|---|---|---|
84388 | 158879 | 2013 | 15 صفحه PDF | دانلود رایگان |
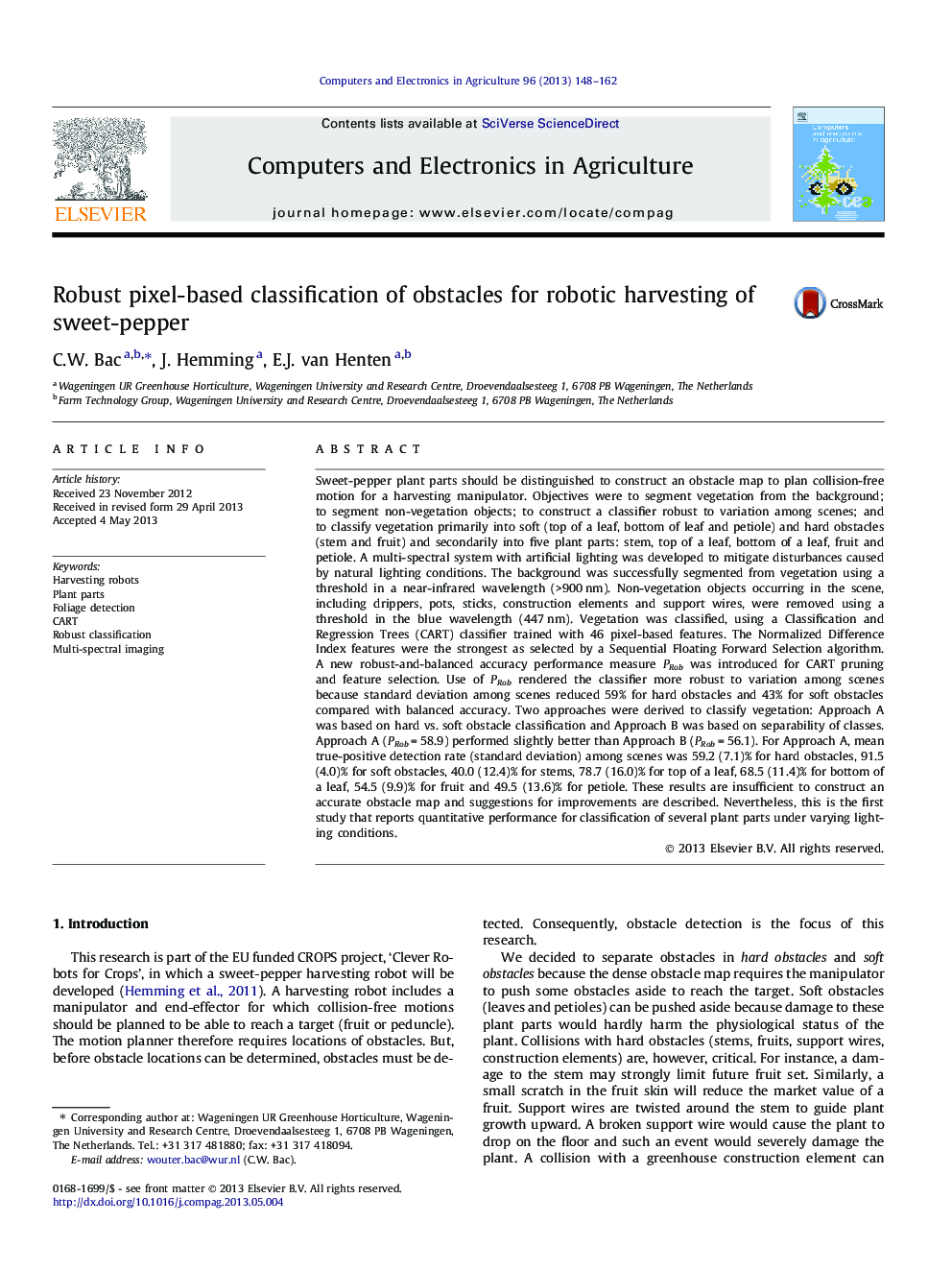
• First study with quantified performance of obstacle classification for harvesting.
• Classification by two approaches, each comprising four classification problems.
• Pixel classification of plant parts under varying light, in a greenhouse, is limited.
• True- and false-positive detection rate of hard obstacles limited to 59.2% and 8.5%.
• A new robust-and-balanced accuracy performance measure PRob successfully introduced.
Sweet-pepper plant parts should be distinguished to construct an obstacle map to plan collision-free motion for a harvesting manipulator. Objectives were to segment vegetation from the background; to segment non-vegetation objects; to construct a classifier robust to variation among scenes; and to classify vegetation primarily into soft (top of a leaf, bottom of leaf and petiole) and hard obstacles (stem and fruit) and secondarily into five plant parts: stem, top of a leaf, bottom of a leaf, fruit and petiole. A multi-spectral system with artificial lighting was developed to mitigate disturbances caused by natural lighting conditions. The background was successfully segmented from vegetation using a threshold in a near-infrared wavelength (>900 nm). Non-vegetation objects occurring in the scene, including drippers, pots, sticks, construction elements and support wires, were removed using a threshold in the blue wavelength (447 nm). Vegetation was classified, using a Classification and Regression Trees (CART) classifier trained with 46 pixel-based features. The Normalized Difference Index features were the strongest as selected by a Sequential Floating Forward Selection algorithm. A new robust-and-balanced accuracy performance measure PRob was introduced for CART pruning and feature selection. Use of PRob rendered the classifier more robust to variation among scenes because standard deviation among scenes reduced 59% for hard obstacles and 43% for soft obstacles compared with balanced accuracy. Two approaches were derived to classify vegetation: Approach A was based on hard vs. soft obstacle classification and Approach B was based on separability of classes. Approach A (PRob = 58.9) performed slightly better than Approach B (PRob = 56.1). For Approach A, mean true-positive detection rate (standard deviation) among scenes was 59.2 (7.1)% for hard obstacles, 91.5 (4.0)% for soft obstacles, 40.0 (12.4)% for stems, 78.7 (16.0)% for top of a leaf, 68.5 (11.4)% for bottom of a leaf, 54.5 (9.9)% for fruit and 49.5 (13.6)% for petiole. These results are insufficient to construct an accurate obstacle map and suggestions for improvements are described. Nevertheless, this is the first study that reports quantitative performance for classification of several plant parts under varying lighting conditions.
Journal: Computers and Electronics in Agriculture - Volume 96, August 2013, Pages 148–162