کد مقاله | کد نشریه | سال انتشار | مقاله انگلیسی | نسخه تمام متن |
---|---|---|---|---|
84750 | 158901 | 2011 | 8 صفحه PDF | دانلود رایگان |
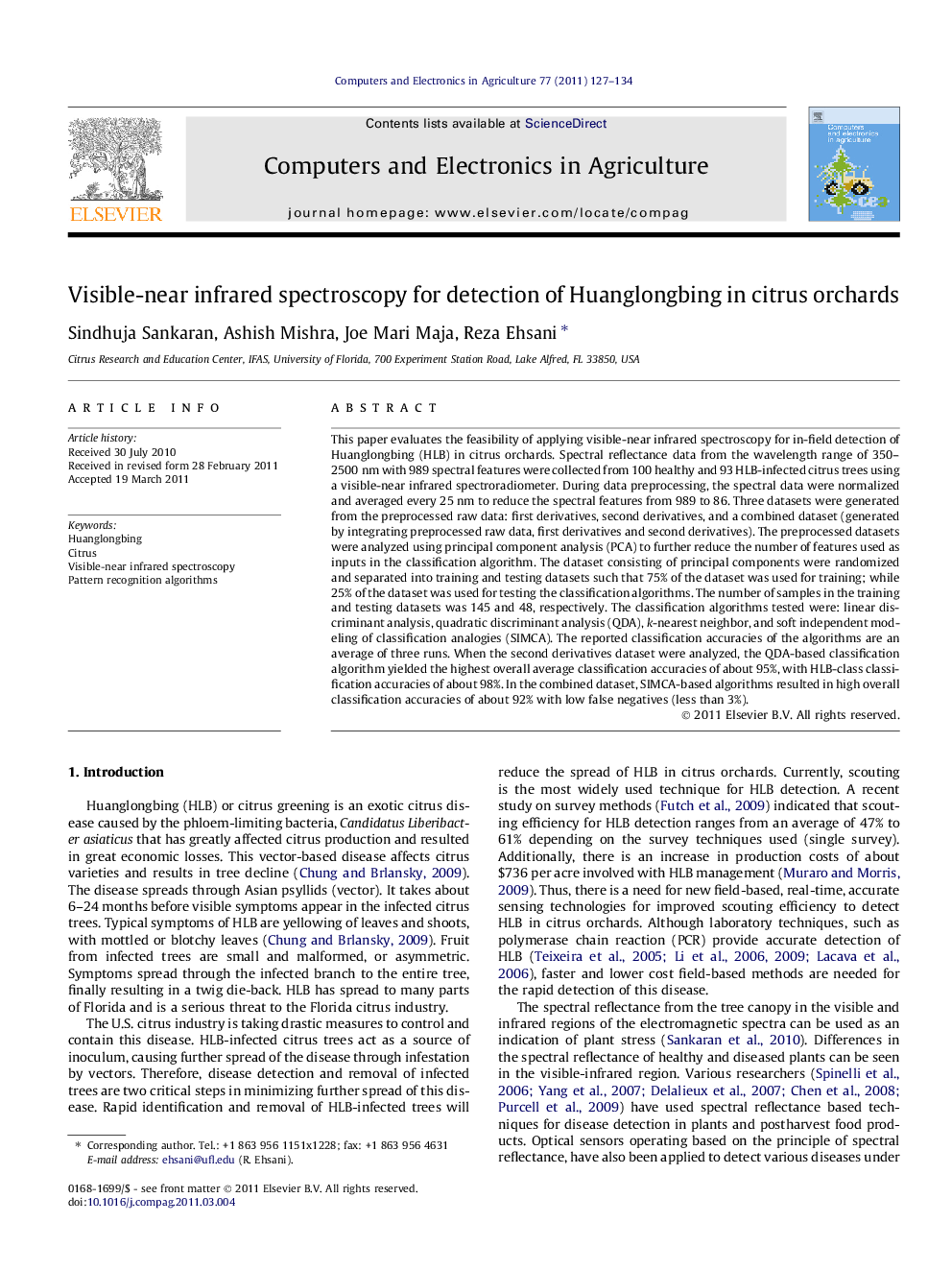
This paper evaluates the feasibility of applying visible-near infrared spectroscopy for in-field detection of Huanglongbing (HLB) in citrus orchards. Spectral reflectance data from the wavelength range of 350–2500 nm with 989 spectral features were collected from 100 healthy and 93 HLB-infected citrus trees using a visible-near infrared spectroradiometer. During data preprocessing, the spectral data were normalized and averaged every 25 nm to reduce the spectral features from 989 to 86. Three datasets were generated from the preprocessed raw data: first derivatives, second derivatives, and a combined dataset (generated by integrating preprocessed raw data, first derivatives and second derivatives). The preprocessed datasets were analyzed using principal component analysis (PCA) to further reduce the number of features used as inputs in the classification algorithm. The dataset consisting of principal components were randomized and separated into training and testing datasets such that 75% of the dataset was used for training; while 25% of the dataset was used for testing the classification algorithms. The number of samples in the training and testing datasets was 145 and 48, respectively. The classification algorithms tested were: linear discriminant analysis, quadratic discriminant analysis (QDA), k-nearest neighbor, and soft independent modeling of classification analogies (SIMCA). The reported classification accuracies of the algorithms are an average of three runs. When the second derivatives dataset were analyzed, the QDA-based classification algorithm yielded the highest overall average classification accuracies of about 95%, with HLB-class classification accuracies of about 98%. In the combined dataset, SIMCA-based algorithms resulted in high overall classification accuracies of about 92% with low false negatives (less than 3%).
► Visible-near infrared spectroscopy as sensing tool for Huanglongbing (HLB) detection.
► Quadratic discriminant analysis results in an overall classification accuracy of 90% and higher.
► Soft independent modeling of classification analogies yielded low false negative values.
► Sensor shows some capability to detect HLB-infected leaves without symptoms.
Journal: Computers and Electronics in Agriculture - Volume 77, Issue 2, July 2011, Pages 127–134