کد مقاله | کد نشریه | سال انتشار | مقاله انگلیسی | نسخه تمام متن |
---|---|---|---|---|
85446 | 158949 | 2006 | 16 صفحه PDF | دانلود رایگان |
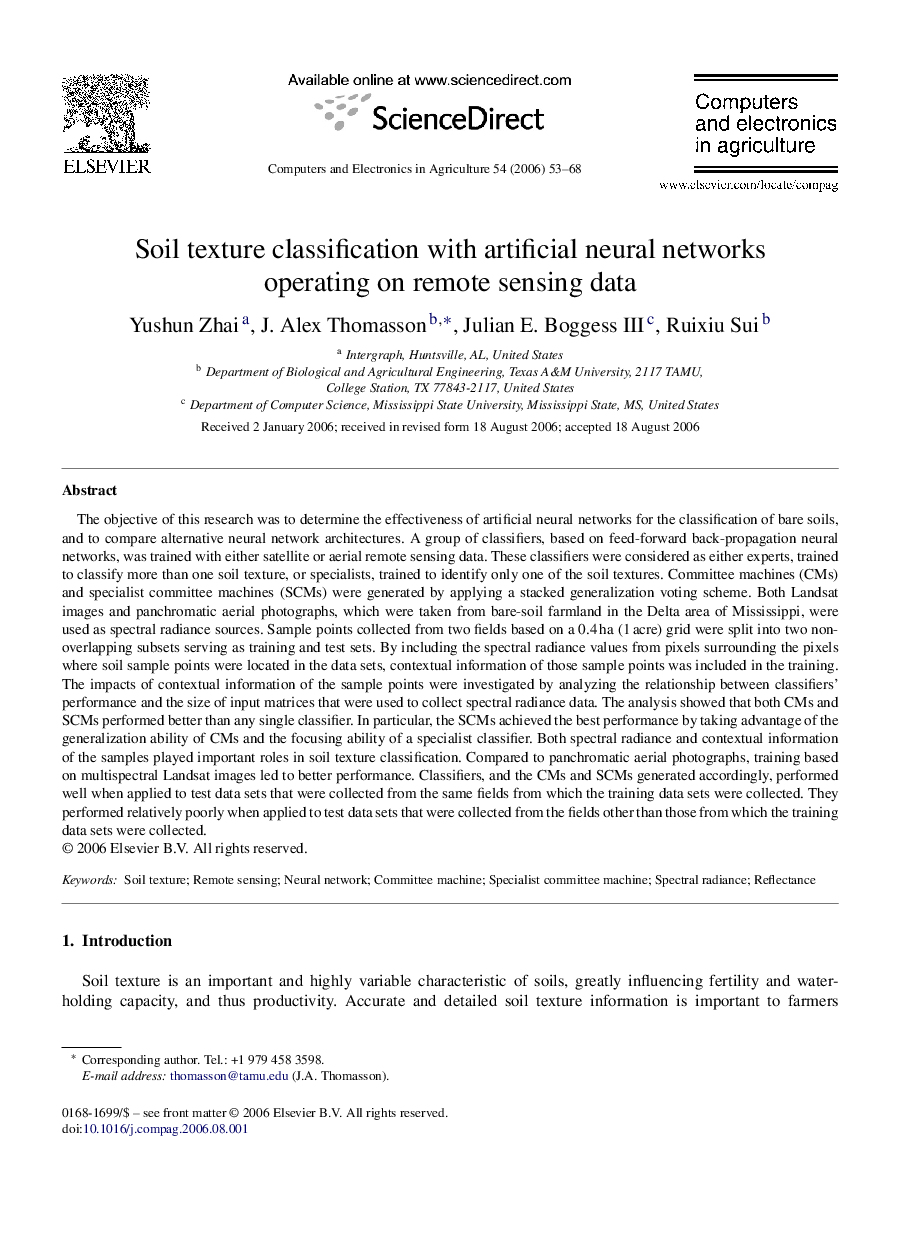
The objective of this research was to determine the effectiveness of artificial neural networks for the classification of bare soils, and to compare alternative neural network architectures. A group of classifiers, based on feed-forward back-propagation neural networks, was trained with either satellite or aerial remote sensing data. These classifiers were considered as either experts, trained to classify more than one soil texture, or specialists, trained to identify only one of the soil textures. Committee machines (CMs) and specialist committee machines (SCMs) were generated by applying a stacked generalization voting scheme. Both Landsat images and panchromatic aerial photographs, which were taken from bare-soil farmland in the Delta area of Mississippi, were used as spectral radiance sources. Sample points collected from two fields based on a 0.4 ha (1 acre) grid were split into two non-overlapping subsets serving as training and test sets. By including the spectral radiance values from pixels surrounding the pixels where soil sample points were located in the data sets, contextual information of those sample points was included in the training. The impacts of contextual information of the sample points were investigated by analyzing the relationship between classifiers’ performance and the size of input matrices that were used to collect spectral radiance data. The analysis showed that both CMs and SCMs performed better than any single classifier. In particular, the SCMs achieved the best performance by taking advantage of the generalization ability of CMs and the focusing ability of a specialist classifier. Both spectral radiance and contextual information of the samples played important roles in soil texture classification. Compared to panchromatic aerial photographs, training based on multispectral Landsat images led to better performance. Classifiers, and the CMs and SCMs generated accordingly, performed well when applied to test data sets that were collected from the same fields from which the training data sets were collected. They performed relatively poorly when applied to test data sets that were collected from the fields other than those from which the training data sets were collected.
Journal: Computers and Electronics in Agriculture - Volume 54, Issue 2, December 2006, Pages 53–68