کد مقاله | کد نشریه | سال انتشار | مقاله انگلیسی | نسخه تمام متن |
---|---|---|---|---|
8686995 | 1580837 | 2018 | 40 صفحه PDF | دانلود رایگان |
عنوان انگلیسی مقاله ISI
A low-rank multivariate general linear model for multi-subject fMRI data and a non-convex optimization algorithm for brain response comparison
دانلود مقاله + سفارش ترجمه
دانلود مقاله ISI انگلیسی
رایگان برای ایرانیان
کلمات کلیدی
موضوعات مرتبط
علوم زیستی و بیوفناوری
علم عصب شناسی
علوم اعصاب شناختی
پیش نمایش صفحه اول مقاله
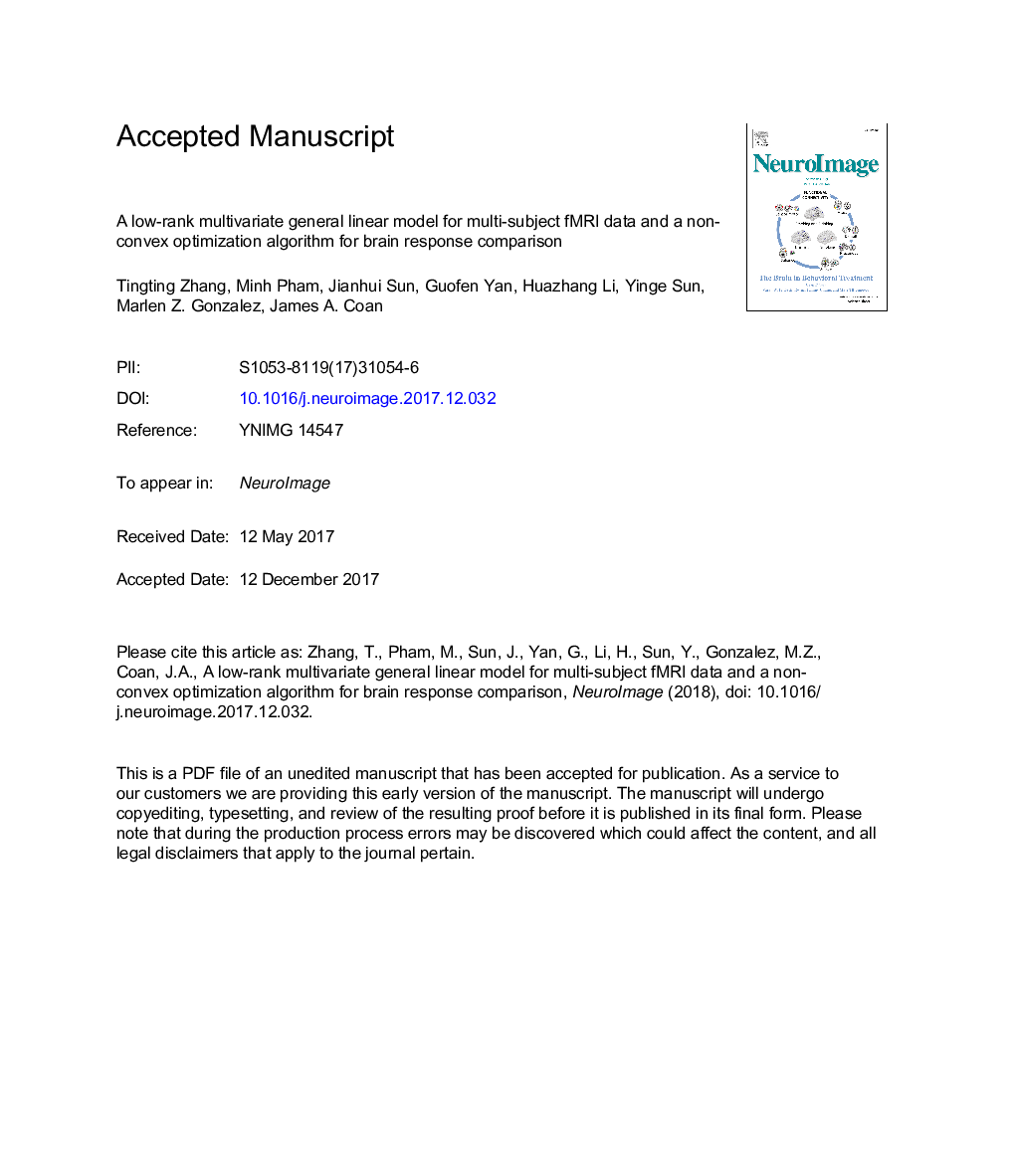
چکیده انگلیسی
The focus of this paper is on evaluating brain responses to different stimuli and identifying brain regions with different responses using multi-subject, stimulus-evoked functional magnetic resonance imaging (fMRI) data. To jointly model many brain voxels' responses to designed stimuli, we present a new low-rank multivariate general linear model (LRMGLM) for stimulus-evoked fMRI data. The new model not only is flexible to characterize variation in hemodynamic response functions (HRFs) across different regions and stimulus types, but also enables information “borrowing” across voxels and uses much fewer parameters than typical nonparametric models for HRFs. To estimate the proposed LRMGLM, we introduce a new penalized optimization function, which leads to temporally and spatially smooth HRF estimates. We develop an efficient optimization algorithm to minimize the optimization function and identify the voxels with different responses to stimuli. We show that the proposed method can outperform several existing voxel-wise methods by achieving both high sensitivity and specificity. We apply the proposed method to the fMRI data collected in an emotion study, and identify anterior dACC to have different responses to a designed threat and control stimuli.
ناشر
Database: Elsevier - ScienceDirect (ساینس دایرکت)
Journal: NeuroImage - Volume 173, June 2018, Pages 580-591
Journal: NeuroImage - Volume 173, June 2018, Pages 580-591
نویسندگان
Tingting Zhang, Minh Pham, Jianhui Sun, Guofen Yan, Huazhang Li, Yinge Sun, Marlen Z. Gonzalez, James A. Coan,