کد مقاله | کد نشریه | سال انتشار | مقاله انگلیسی | نسخه تمام متن |
---|---|---|---|---|
8918075 | 1642816 | 2018 | 25 صفحه PDF | دانلود رایگان |
عنوان انگلیسی مقاله ISI
Manifold learning-based methods for analyzing single-cell RNA-sequencing data
دانلود مقاله + سفارش ترجمه
دانلود مقاله ISI انگلیسی
رایگان برای ایرانیان
کلمات کلیدی
موضوعات مرتبط
مهندسی و علوم پایه
مهندسی کامپیوتر
علوم کامپیوتر (عمومی)
پیش نمایش صفحه اول مقاله
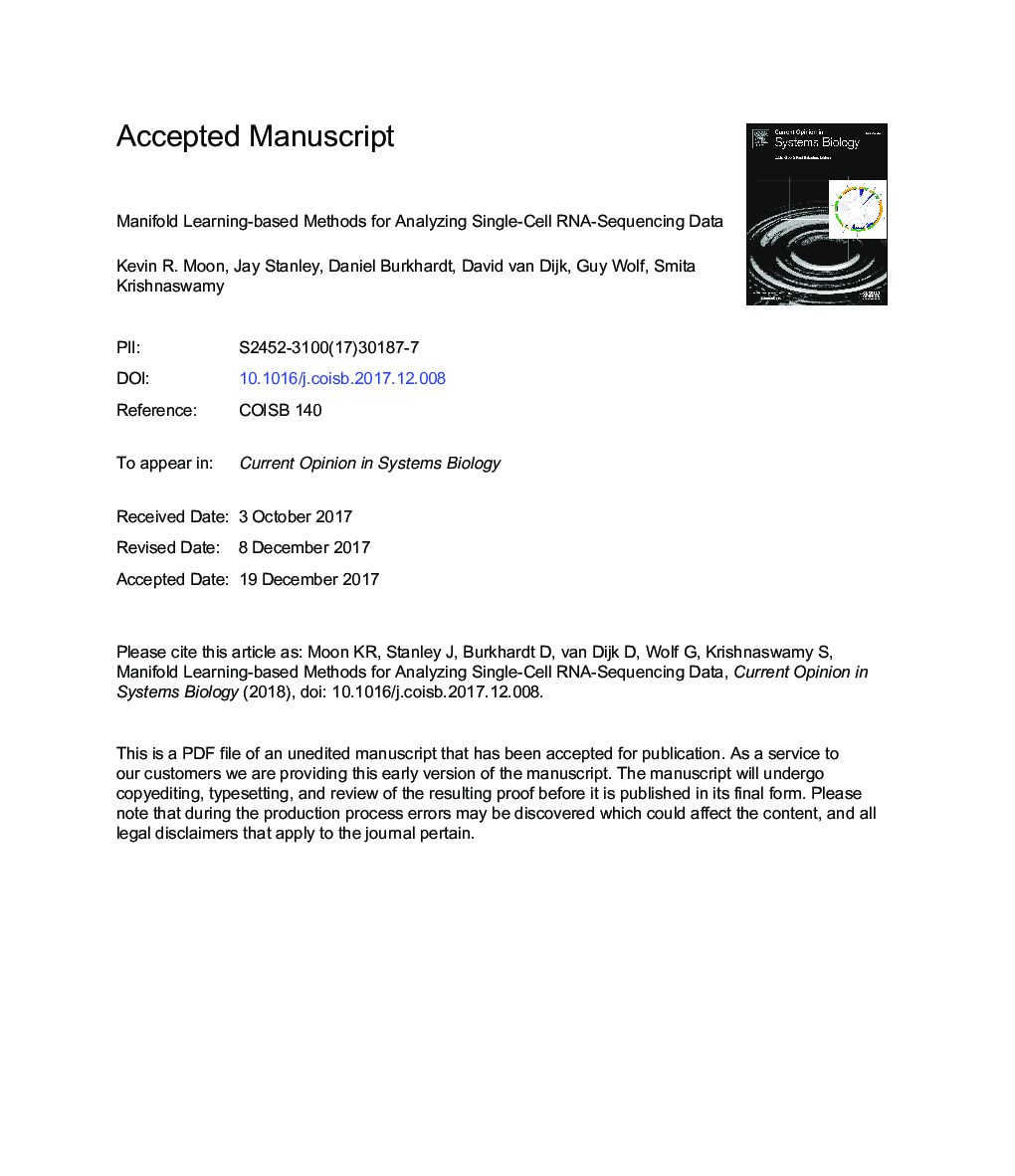
چکیده انگلیسی
Recent advances in single-cell RNA sequencing technologies enable deep insights into cellular development, gene regulation, and phenotypic diversity by measuring gene expression for thousands of cells in a single experiment. While these technologies hold great potential for improving our understanding of cellular states and progression, they also pose new challenges and require advanced mathematical and algorithmic tools to extract underlying biological signals. In this review, we cover one of the most promising avenues of research into unlocking the potential of scRNA-seq data: the field of manifold learning, and the related manifold assumption in data analysis. Manifold learning provides a powerful structure for algorithmic approaches to process the data, extract its dynamics, and infer patterns in it. In particular, we cover manifold learning-based methods for denoising the data, revealing gene interactions, extracting pseudotime progressions with model fitting, visualizing the cellular state space via dimensionality reduction, and clustering the data.
ناشر
Database: Elsevier - ScienceDirect (ساینس دایرکت)
Journal: Current Opinion in Systems Biology - Volume 7, February 2018, Pages 36-46
Journal: Current Opinion in Systems Biology - Volume 7, February 2018, Pages 36-46
نویسندگان
Kevin R. Moon, Jay S. III, Daniel Burkhardt, David van Dijk, Guy Wolf, Smita Krishnaswamy,