کد مقاله | کد نشریه | سال انتشار | مقاله انگلیسی | نسخه تمام متن |
---|---|---|---|---|
979228 | 933329 | 2010 | 6 صفحه PDF | دانلود رایگان |
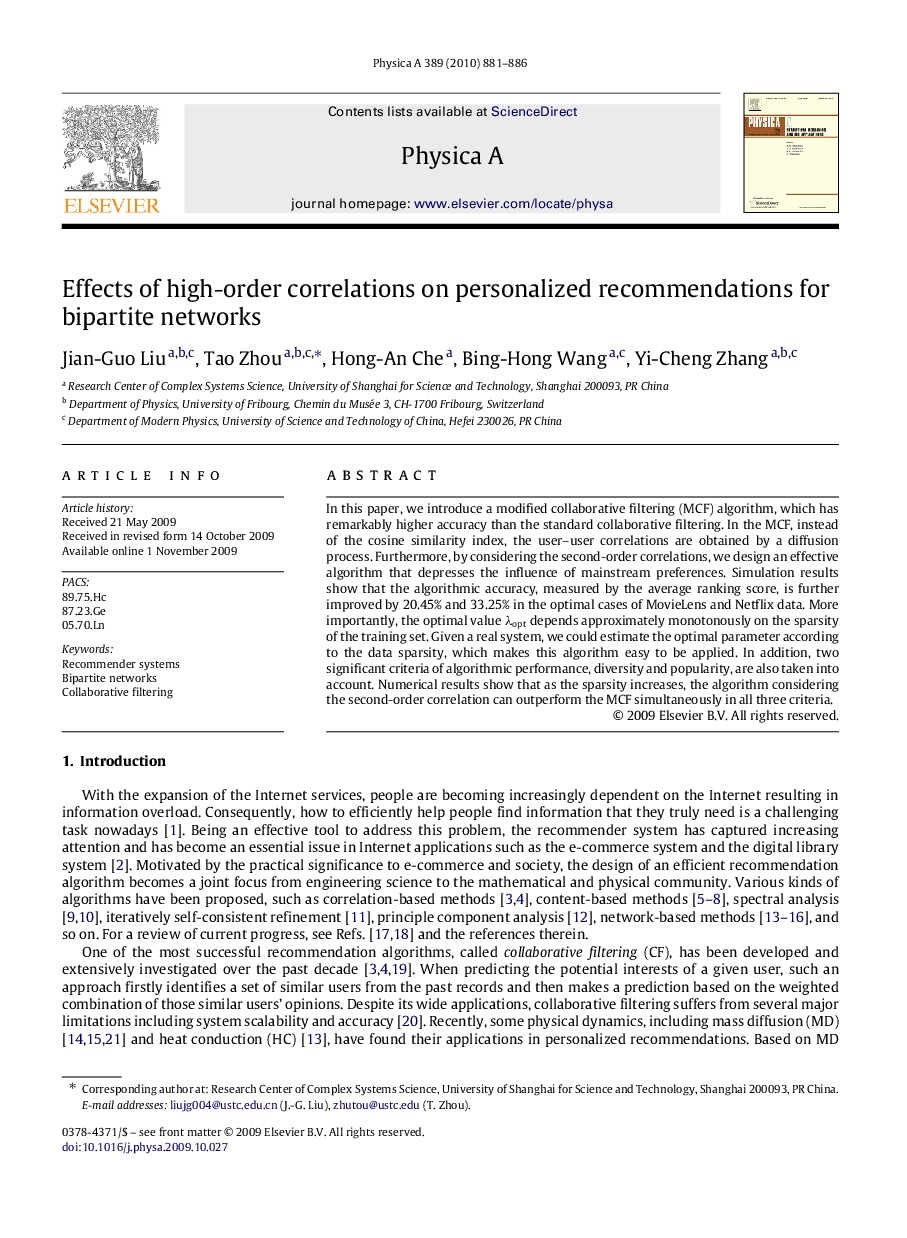
In this paper, we introduce a modified collaborative filtering (MCF) algorithm, which has remarkably higher accuracy than the standard collaborative filtering. In the MCF, instead of the cosine similarity index, the user–user correlations are obtained by a diffusion process. Furthermore, by considering the second-order correlations, we design an effective algorithm that depresses the influence of mainstream preferences. Simulation results show that the algorithmic accuracy, measured by the average ranking score, is further improved by 20.45% and 33.25% in the optimal cases of MovieLens and Netflix data. More importantly, the optimal value λopt depends approximately monotonously on the sparsity of the training set. Given a real system, we could estimate the optimal parameter according to the data sparsity, which makes this algorithm easy to be applied. In addition, two significant criteria of algorithmic performance, diversity and popularity, are also taken into account. Numerical results show that as the sparsity increases, the algorithm considering the second-order correlation can outperform the MCF simultaneously in all three criteria.
Journal: Physica A: Statistical Mechanics and its Applications - Volume 389, Issue 4, 15 February 2010, Pages 881–886