کد مقاله | کد نشریه | سال انتشار | مقاله انگلیسی | نسخه تمام متن |
---|---|---|---|---|
10322819 | 660871 | 2015 | 36 صفحه PDF | دانلود رایگان |
عنوان انگلیسی مقاله ISI
MARK-ELM: Application of a novel Multiple Kernel Learning framework for improving the robustness of Network Intrusion Detection
دانلود مقاله + سفارش ترجمه
دانلود مقاله ISI انگلیسی
رایگان برای ایرانیان
کلمات کلیدی
cyber security - امنیت سایبریKernel selection - انتخاب کرنلNetwork intrusion detection - تشخیص نفوذ شبکهAdaptive boosting - تقویت سازگاریExtreme learning machine - دستگاه یادگیری شدیدMulticlass classification - طبقه بندی چند طبقهMachine learning - یادگیری ماشینMultiple kernel learning - یادگیری چند هسته ایEnsemble learning - یادگیری گروهی
موضوعات مرتبط
مهندسی و علوم پایه
مهندسی کامپیوتر
هوش مصنوعی
پیش نمایش صفحه اول مقاله
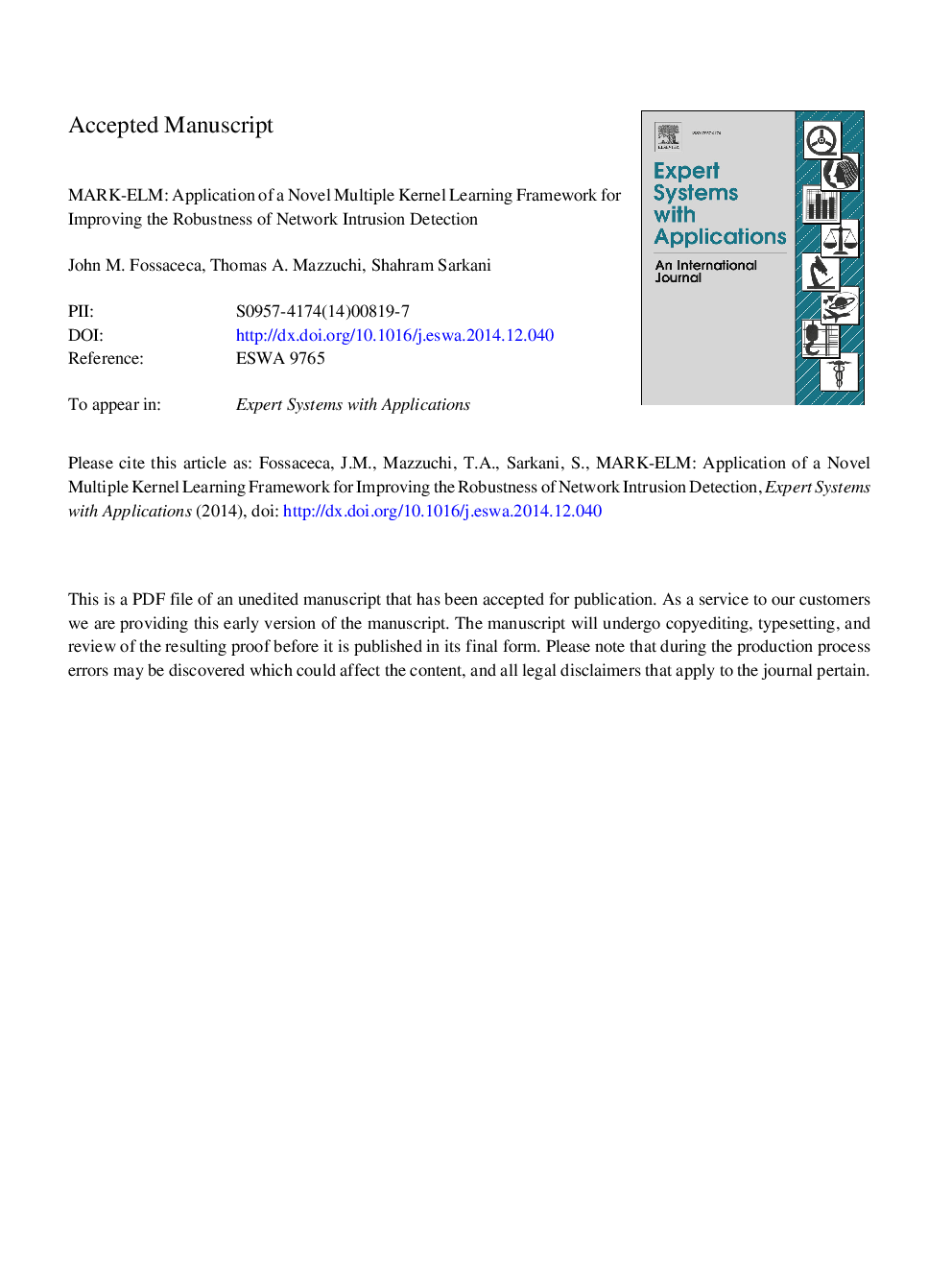
چکیده انگلیسی
Detection of cyber-based attacks on computer networks continues to be a relevant and challenging area of research. Daily reports of incidents appear in public media including major ex-filtrations of data for the purposes of stealing identities, credit card numbers, and intellectual property as well as to take control of network resources. Methods used by attackers constantly change in order to defeat techniques employed by information technology (IT) teams intended to discover or block intrusions. “Zero Day” attacks whose “signatures” are not yet in IT databases are continually being uncovered. Machine learning approaches have been widely used to increase the effectiveness of intrusion detection platforms. While some machine learning techniques are effective at detecting certain types of attacks, there are no known methods that can be applied universally and achieve consistent results for multiple attack types. The focus of our research is the development of a framework that combines the outputs of multiple learners in order to improve the efficacy of network intrusion on data that contains instances of multiple classes of attacks. We have chosen the Extreme Learning Machine (ELM) as the core learning algorithm due to recent research that suggests that ELMs are straightforward to implement, computationally efficient and have excellent learning performance characteristics on par with the Support Vector Machine (SVM), one of the most widely used and best performing machine learning platforms (Liu, Gao, & Li, 2012). We introduce the novel Multiple Adaptive Reduced Kernel Extreme Learning Machine (MARK-ELM) which combines Multiple Kernel Boosting (Xia & Hoi, 2013) with the Multiple Classification Reduced Kernel ELM (Deng, Zheng, & Zhang, 2013). We tested this approach on several machine learning datasets as well as the KDD Cup 99 (Hettich & Bay, 1999) intrusion detection dataset. Our results indicate that MARK-ELM works well for the majority of University of California, Irvine (UCI) Machine Learning Repository small datasets and is scalable for larger datasets. For UCI datasets we achieved performance similar to the MKBoost Support Vector Machine (SVM) approach. In our experiments we demonstrate that MARK-ELM achieves superior detection rates and much lower false alarm rates than other approaches on intrusion detection data.
ناشر
Database: Elsevier - ScienceDirect (ساینس دایرکت)
Journal: Expert Systems with Applications - Volume 42, Issue 8, 15 May 2015, Pages 4062-4080
Journal: Expert Systems with Applications - Volume 42, Issue 8, 15 May 2015, Pages 4062-4080
نویسندگان
John M. Fossaceca, Thomas A. Mazzuchi, Shahram Sarkani,