کد مقاله | کد نشریه | سال انتشار | مقاله انگلیسی | نسخه تمام متن |
---|---|---|---|---|
1146257 | 957501 | 2012 | 23 صفحه PDF | دانلود رایگان |
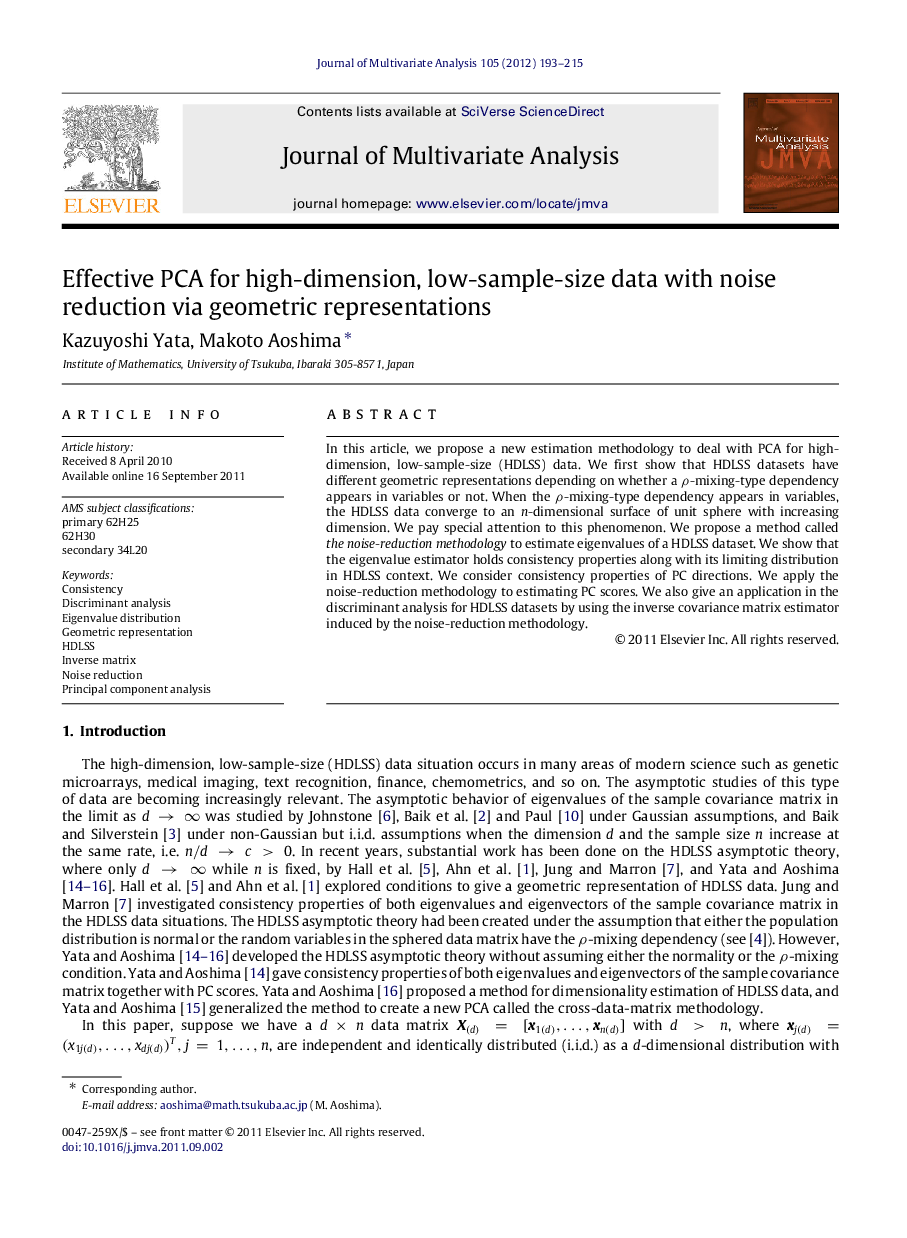
In this article, we propose a new estimation methodology to deal with PCA for high-dimension, low-sample-size (HDLSS) data. We first show that HDLSS datasets have different geometric representations depending on whether a ρρ-mixing-type dependency appears in variables or not. When the ρρ-mixing-type dependency appears in variables, the HDLSS data converge to an nn-dimensional surface of unit sphere with increasing dimension. We pay special attention to this phenomenon. We propose a method called the noise-reduction methodology to estimate eigenvalues of a HDLSS dataset. We show that the eigenvalue estimator holds consistency properties along with its limiting distribution in HDLSS context. We consider consistency properties of PC directions. We apply the noise-reduction methodology to estimating PC scores. We also give an application in the discriminant analysis for HDLSS datasets by using the inverse covariance matrix estimator induced by the noise-reduction methodology.
Journal: Journal of Multivariate Analysis - Volume 105, Issue 1, February 2012, Pages 193–215