کد مقاله | کد نشریه | سال انتشار | مقاله انگلیسی | نسخه تمام متن |
---|---|---|---|---|
1731174 | 1521454 | 2016 | 10 صفحه PDF | دانلود رایگان |
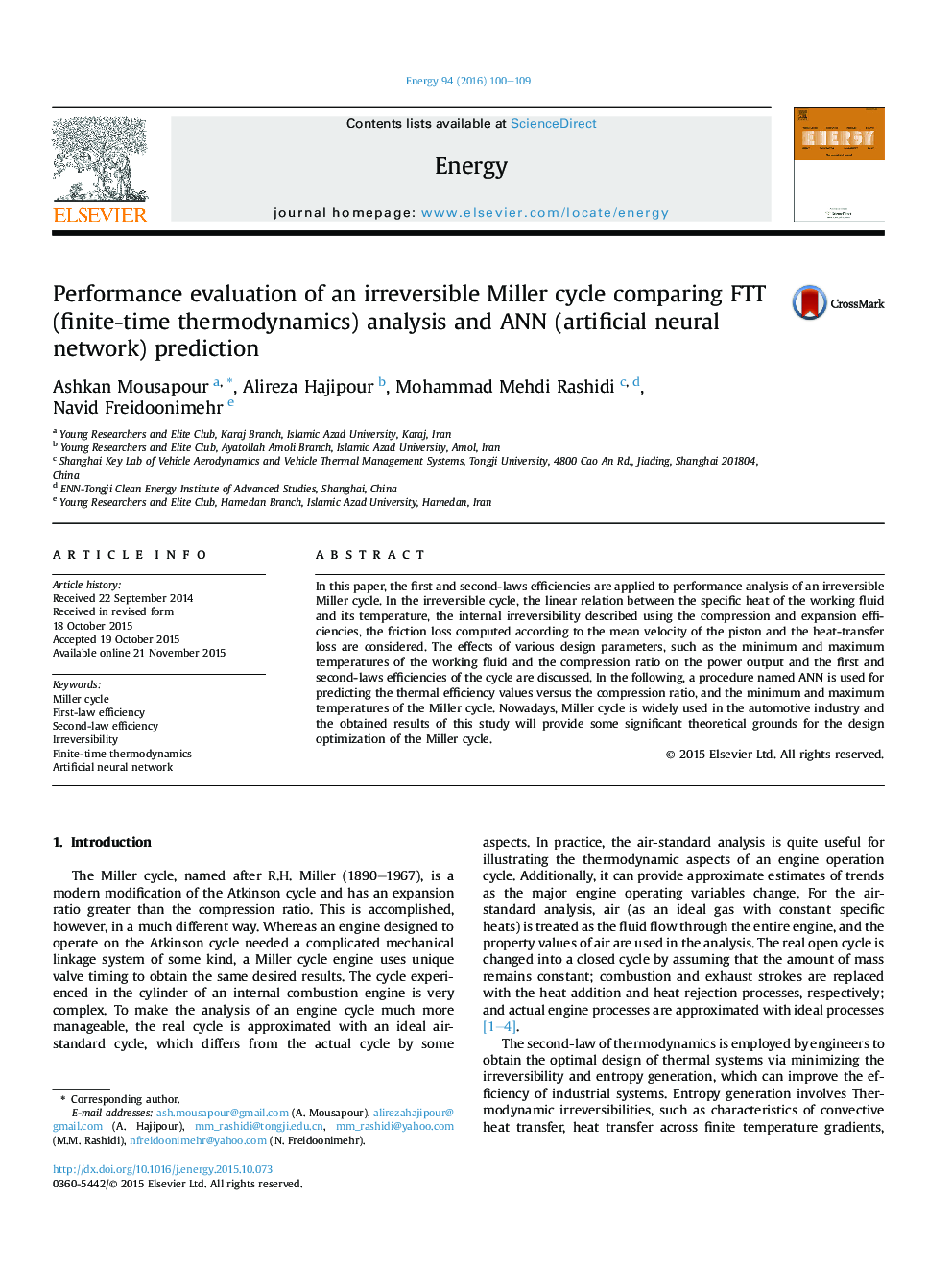
• The performance of an irreversible Miller cycle is investigated using FFT.
• The effects of design parameters on the performance of the cycle are investigated.
• ANN is applied to predict the thermal efficiency and the power output values.
• There is an excellent correlation between FTT and ANN data.
• ANN can be applied to predict data where FTT analysis has not been performed.
In this paper, the first and second-laws efficiencies are applied to performance analysis of an irreversible Miller cycle. In the irreversible cycle, the linear relation between the specific heat of the working fluid and its temperature, the internal irreversibility described using the compression and expansion efficiencies, the friction loss computed according to the mean velocity of the piston and the heat-transfer loss are considered. The effects of various design parameters, such as the minimum and maximum temperatures of the working fluid and the compression ratio on the power output and the first and second-laws efficiencies of the cycle are discussed. In the following, a procedure named ANN is used for predicting the thermal efficiency values versus the compression ratio, and the minimum and maximum temperatures of the Miller cycle. Nowadays, Miller cycle is widely used in the automotive industry and the obtained results of this study will provide some significant theoretical grounds for the design optimization of the Miller cycle.
Journal: Energy - Volume 94, 1 January 2016, Pages 100–109