کد مقاله | کد نشریه | سال انتشار | مقاله انگلیسی | نسخه تمام متن |
---|---|---|---|---|
1757478 | 1019127 | 2015 | 10 صفحه PDF | دانلود رایگان |
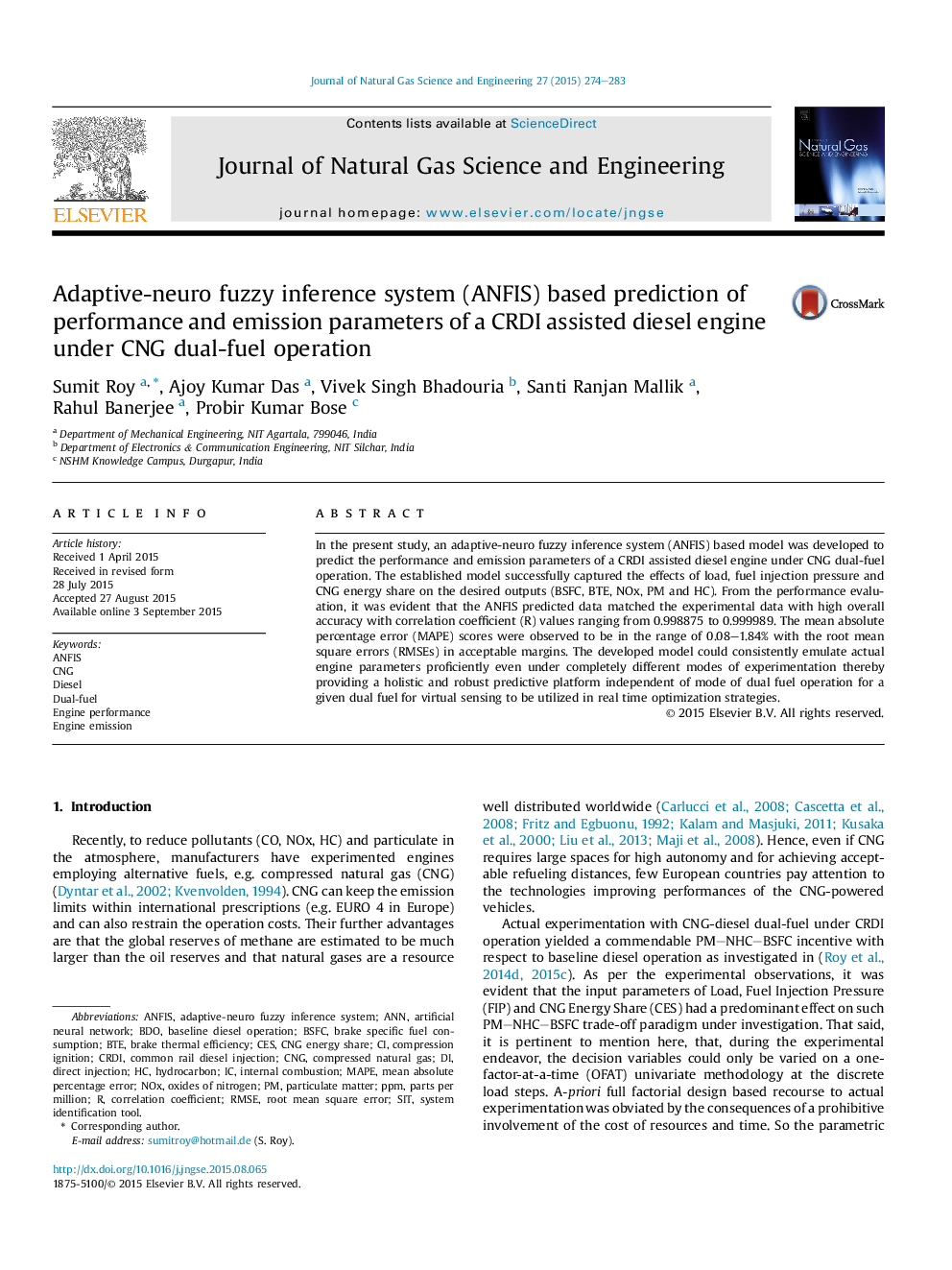
• CRDI-CNG dual-fuel operation for better performance and emission.
• ANFIS as a system identification tool.
• ANFIS based modeling of BSFC, BTE, NOx, PM and HC.
• ANFIS is capable in predicting performance and emission parameters of the experimental engine with commendable accuracy.
In the present study, an adaptive-neuro fuzzy inference system (ANFIS) based model was developed to predict the performance and emission parameters of a CRDI assisted diesel engine under CNG dual-fuel operation. The established model successfully captured the effects of load, fuel injection pressure and CNG energy share on the desired outputs (BSFC, BTE, NOx, PM and HC). From the performance evaluation, it was evident that the ANFIS predicted data matched the experimental data with high overall accuracy with correlation coefficient (R) values ranging from 0.998875 to 0.999989. The mean absolute percentage error (MAPE) scores were observed to be in the range of 0.08–1.84% with the root mean square errors (RMSEs) in acceptable margins. The developed model could consistently emulate actual engine parameters proficiently even under completely different modes of experimentation thereby providing a holistic and robust predictive platform independent of mode of dual fuel operation for a given dual fuel for virtual sensing to be utilized in real time optimization strategies.
Journal: Journal of Natural Gas Science and Engineering - Volume 27, Part 1, November 2015, Pages 274–283