کد مقاله | کد نشریه | سال انتشار | مقاله انگلیسی | نسخه تمام متن |
---|---|---|---|---|
1758083 | 1523025 | 2013 | 11 صفحه PDF | دانلود رایگان |
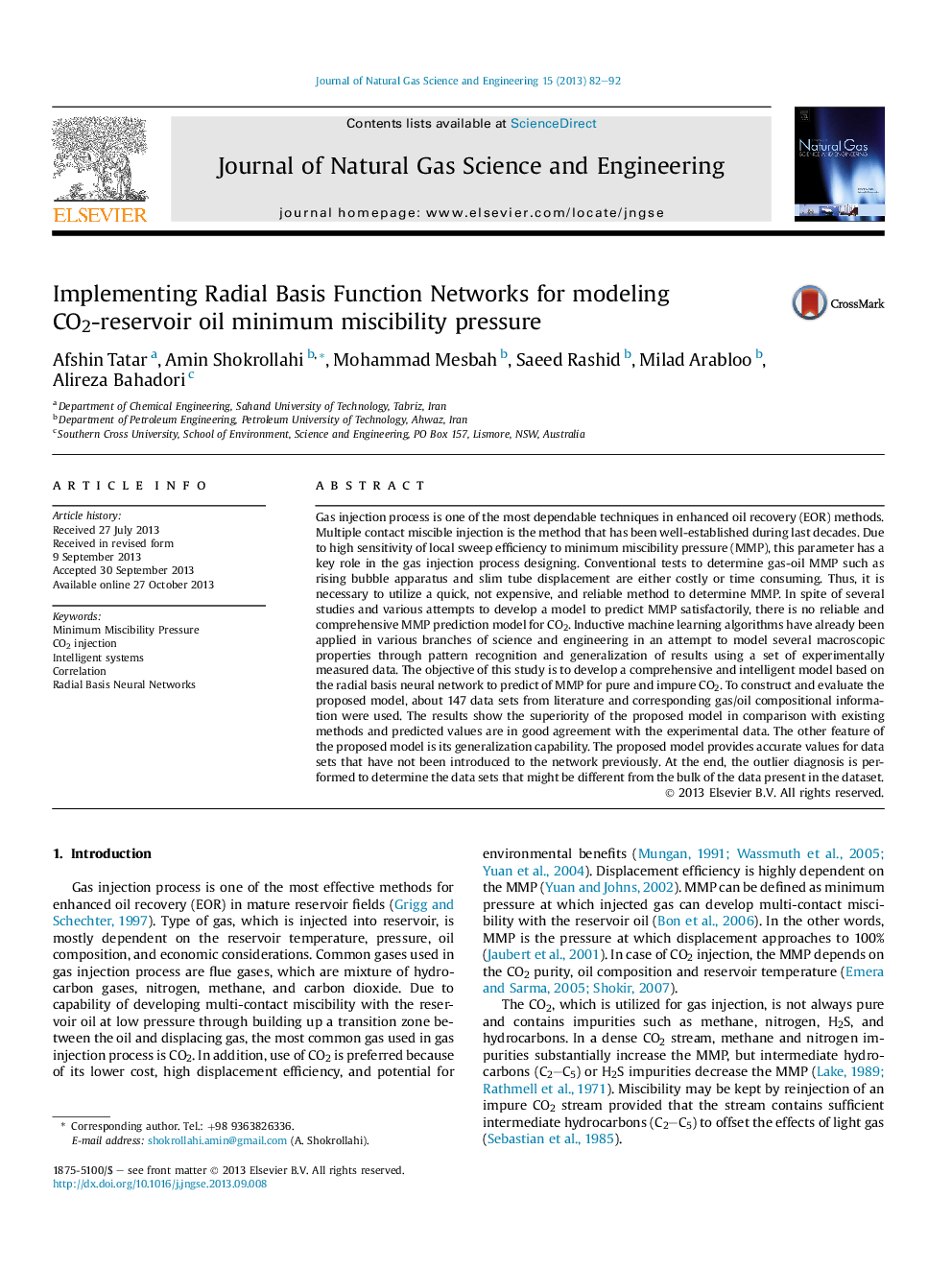
• Radial basis functions used for compositional modeling of CO2-reservoir oil MMP.
• Comparative studies were carried out between proposed model and the other empirical correlations.
• The validity of the model to the experimental data was successfully verified.
• Outlier diagnosis was performed for detection of the probable doubtful data.
Gas injection process is one of the most dependable techniques in enhanced oil recovery (EOR) methods. Multiple contact miscible injection is the method that has been well-established during last decades. Due to high sensitivity of local sweep efficiency to minimum miscibility pressure (MMP), this parameter has a key role in the gas injection process designing. Conventional tests to determine gas-oil MMP such as rising bubble apparatus and slim tube displacement are either costly or time consuming. Thus, it is necessary to utilize a quick, not expensive, and reliable method to determine MMP. In spite of several studies and various attempts to develop a model to predict MMP satisfactorily, there is no reliable and comprehensive MMP prediction model for CO2. Inductive machine learning algorithms have already been applied in various branches of science and engineering in an attempt to model several macroscopic properties through pattern recognition and generalization of results using a set of experimentally measured data. The objective of this study is to develop a comprehensive and intelligent model based on the radial basis neural network to predict of MMP for pure and impure CO2. To construct and evaluate the proposed model, about 147 data sets from literature and corresponding gas/oil compositional information were used. The results show the superiority of the proposed model in comparison with existing methods and predicted values are in good agreement with the experimental data. The other feature of the proposed model is its generalization capability. The proposed model provides accurate values for data sets that have not been introduced to the network previously. At the end, the outlier diagnosis is performed to determine the data sets that might be different from the bulk of the data present in the dataset.
Figure optionsDownload high-quality image (102 K)Download as PowerPoint slide
Journal: Journal of Natural Gas Science and Engineering - Volume 15, November 2013, Pages 82–92