کد مقاله | کد نشریه | سال انتشار | مقاله انگلیسی | نسخه تمام متن |
---|---|---|---|---|
1994674 | 1541277 | 2016 | 11 صفحه PDF | دانلود رایگان |
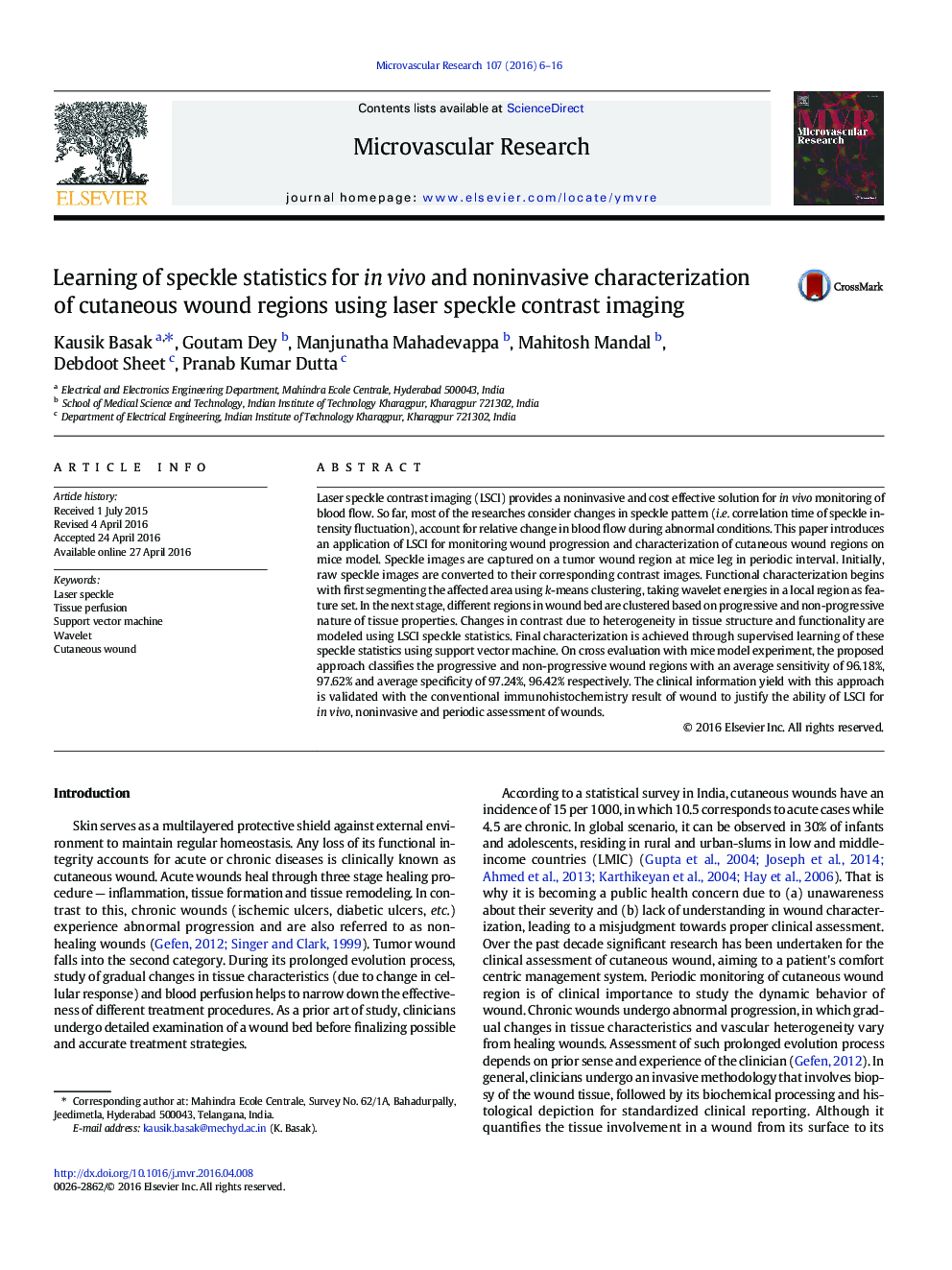
• Optical nature of different tissues results in characteristic response of LSCI signals.
• Supervised learning of speckle statistics for characterization of wound progression is proposed.
• Experiments are performed on cutaneous tumour wound of mice.
• The framework can accurately track changes in structural and functional attributes of tissue in tumour wound region.
• The clinical information is validated with immunohistochemistry result of the wound.
• Quantitative analysis shows significant results in terms of sensitivity, specificity and ROC.
Laser speckle contrast imaging (LSCI) provides a noninvasive and cost effective solution for in vivo monitoring of blood flow. So far, most of the researches consider changes in speckle pattern (i.e. correlation time of speckle intensity fluctuation), account for relative change in blood flow during abnormal conditions. This paper introduces an application of LSCI for monitoring wound progression and characterization of cutaneous wound regions on mice model. Speckle images are captured on a tumor wound region at mice leg in periodic interval. Initially, raw speckle images are converted to their corresponding contrast images. Functional characterization begins with first segmenting the affected area using k-means clustering, taking wavelet energies in a local region as feature set. In the next stage, different regions in wound bed are clustered based on progressive and non-progressive nature of tissue properties. Changes in contrast due to heterogeneity in tissue structure and functionality are modeled using LSCI speckle statistics. Final characterization is achieved through supervised learning of these speckle statistics using support vector machine. On cross evaluation with mice model experiment, the proposed approach classifies the progressive and non-progressive wound regions with an average sensitivity of 96.18%, 97.62% and average specificity of 97.24%, 96.42% respectively. The clinical information yield with this approach is validated with the conventional immunohistochemistry result of wound to justify the ability of LSCI for in vivo, noninvasive and periodic assessment of wounds.
Journal: Microvascular Research - Volume 107, September 2016, Pages 6–16