کد مقاله | کد نشریه | سال انتشار | مقاله انگلیسی | نسخه تمام متن |
---|---|---|---|---|
260938 | 503674 | 2008 | 9 صفحه PDF | دانلود رایگان |
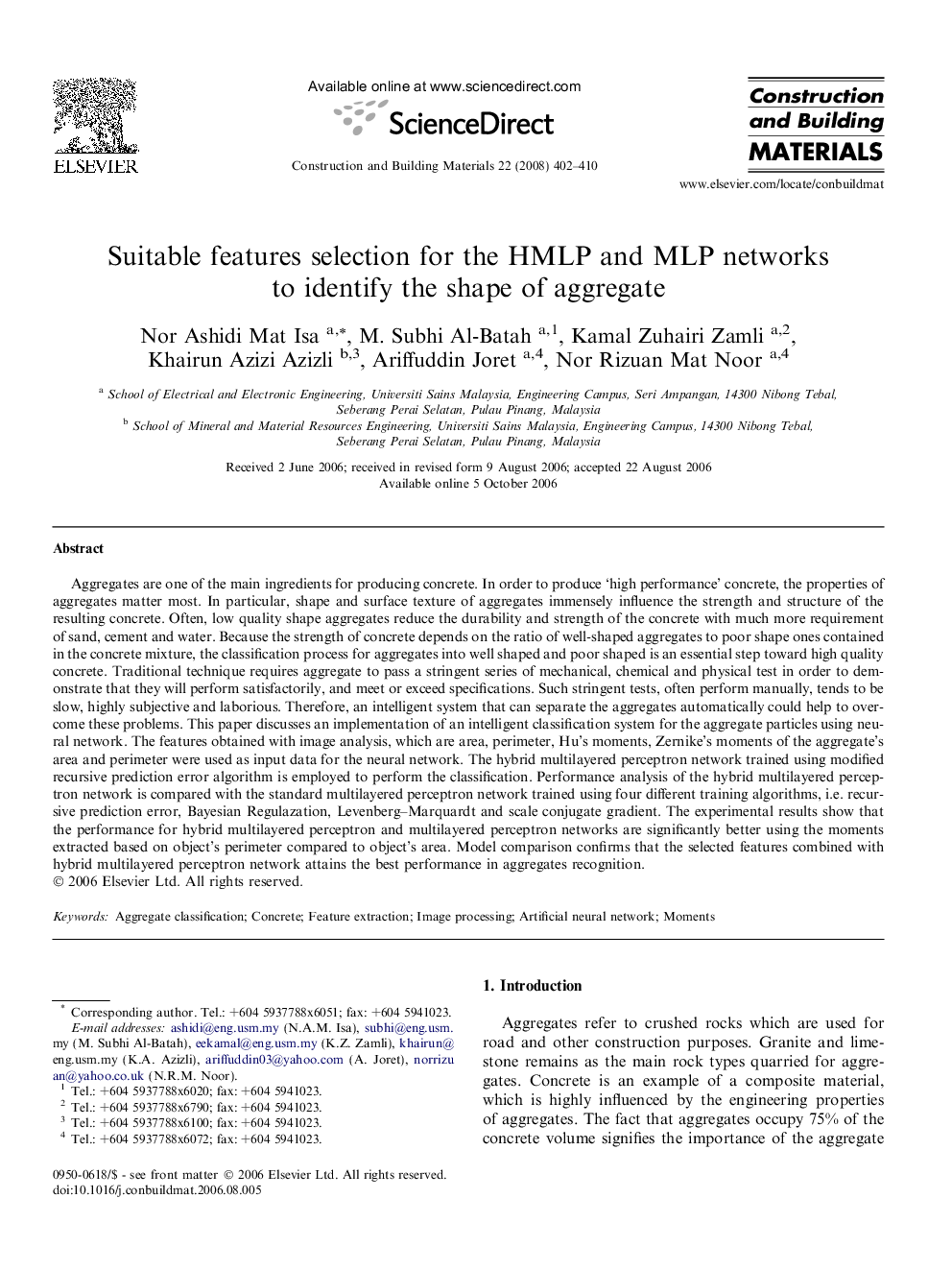
Aggregates are one of the main ingredients for producing concrete. In order to produce ‘high performance’ concrete, the properties of aggregates matter most. In particular, shape and surface texture of aggregates immensely influence the strength and structure of the resulting concrete. Often, low quality shape aggregates reduce the durability and strength of the concrete with much more requirement of sand, cement and water. Because the strength of concrete depends on the ratio of well-shaped aggregates to poor shape ones contained in the concrete mixture, the classification process for aggregates into well shaped and poor shaped is an essential step toward high quality concrete. Traditional technique requires aggregate to pass a stringent series of mechanical, chemical and physical test in order to demonstrate that they will perform satisfactorily, and meet or exceed specifications. Such stringent tests, often perform manually, tends to be slow, highly subjective and laborious. Therefore, an intelligent system that can separate the aggregates automatically could help to overcome these problems. This paper discusses an implementation of an intelligent classification system for the aggregate particles using neural network. The features obtained with image analysis, which are area, perimeter, Hu’s moments, Zernike’s moments of the aggregate’s area and perimeter were used as input data for the neural network. The hybrid multilayered perceptron network trained using modified recursive prediction error algorithm is employed to perform the classification. Performance analysis of the hybrid multilayered perceptron network is compared with the standard multilayered perceptron network trained using four different training algorithms, i.e. recursive prediction error, Bayesian Regulazation, Levenberg–Marquardt and scale conjugate gradient. The experimental results show that the performance for hybrid multilayered perceptron and multilayered perceptron networks are significantly better using the moments extracted based on object’s perimeter compared to object’s area. Model comparison confirms that the selected features combined with hybrid multilayered perceptron network attains the best performance in aggregates recognition.
Journal: Construction and Building Materials - Volume 22, Issue 3, March 2008, Pages 402–410