کد مقاله | کد نشریه | سال انتشار | مقاله انگلیسی | نسخه تمام متن |
---|---|---|---|---|
380359 | 1437437 | 2015 | 15 صفحه PDF | دانلود رایگان |
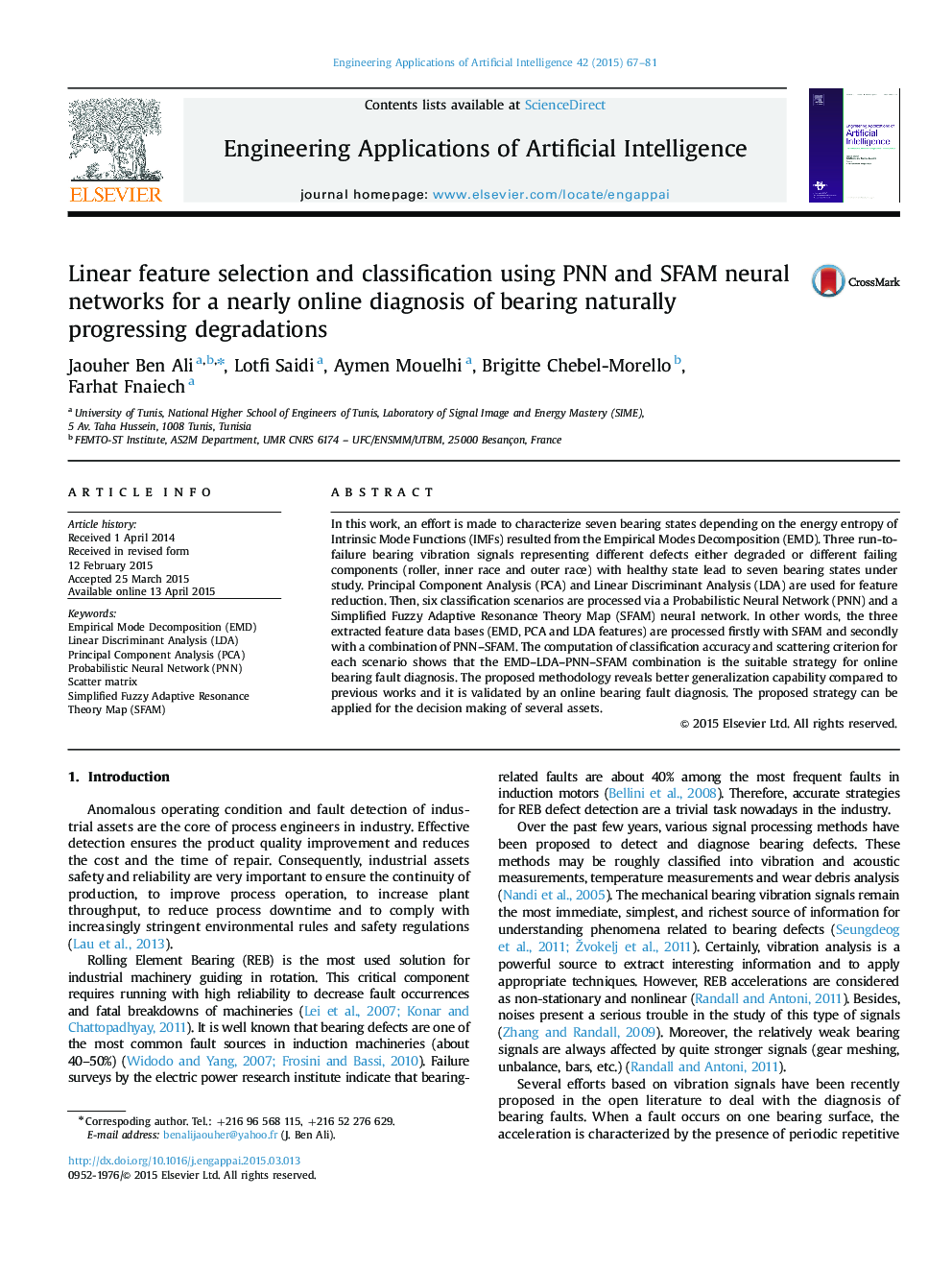
• A new methodology proposed for a nearly online damage stage detection.
• The proposed strategy is based on neural networks and EMD method.
• The nearly online detection of bearing health status is done thanks to various damage stages.
• Experimental results show that this methodology is very effective.
• Unlike previous works, the proposed method is tested for the diagnosis of naturally progressing bearing degradations.
In this work, an effort is made to characterize seven bearing states depending on the energy entropy of Intrinsic Mode Functions (IMFs) resulted from the Empirical Modes Decomposition (EMD). Three run-to-failure bearing vibration signals representing different defects either degraded or different failing components (roller, inner race and outer race) with healthy state lead to seven bearing states under study. Principal Component Analysis (PCA) and Linear Discriminant Analysis (LDA) are used for feature reduction. Then, six classification scenarios are processed via a Probabilistic Neural Network (PNN) and a Simplified Fuzzy Adaptive Resonance Theory Map (SFAM) neural network. In other words, the three extracted feature data bases (EMD, PCA and LDA features) are processed firstly with SFAM and secondly with a combination of PNN–SFAM. The computation of classification accuracy and scattering criterion for each scenario shows that the EMD–LDA–PNN–SFAM combination is the suitable strategy for online bearing fault diagnosis. The proposed methodology reveals better generalization capability compared to previous works and it is validated by an online bearing fault diagnosis. The proposed strategy can be applied for the decision making of several assets.
Figure optionsDownload as PowerPoint slide
Journal: Engineering Applications of Artificial Intelligence - Volume 42, June 2015, Pages 67–81