کد مقاله | کد نشریه | سال انتشار | مقاله انگلیسی | نسخه تمام متن |
---|---|---|---|---|
383648 | 660828 | 2014 | 13 صفحه PDF | دانلود رایگان |
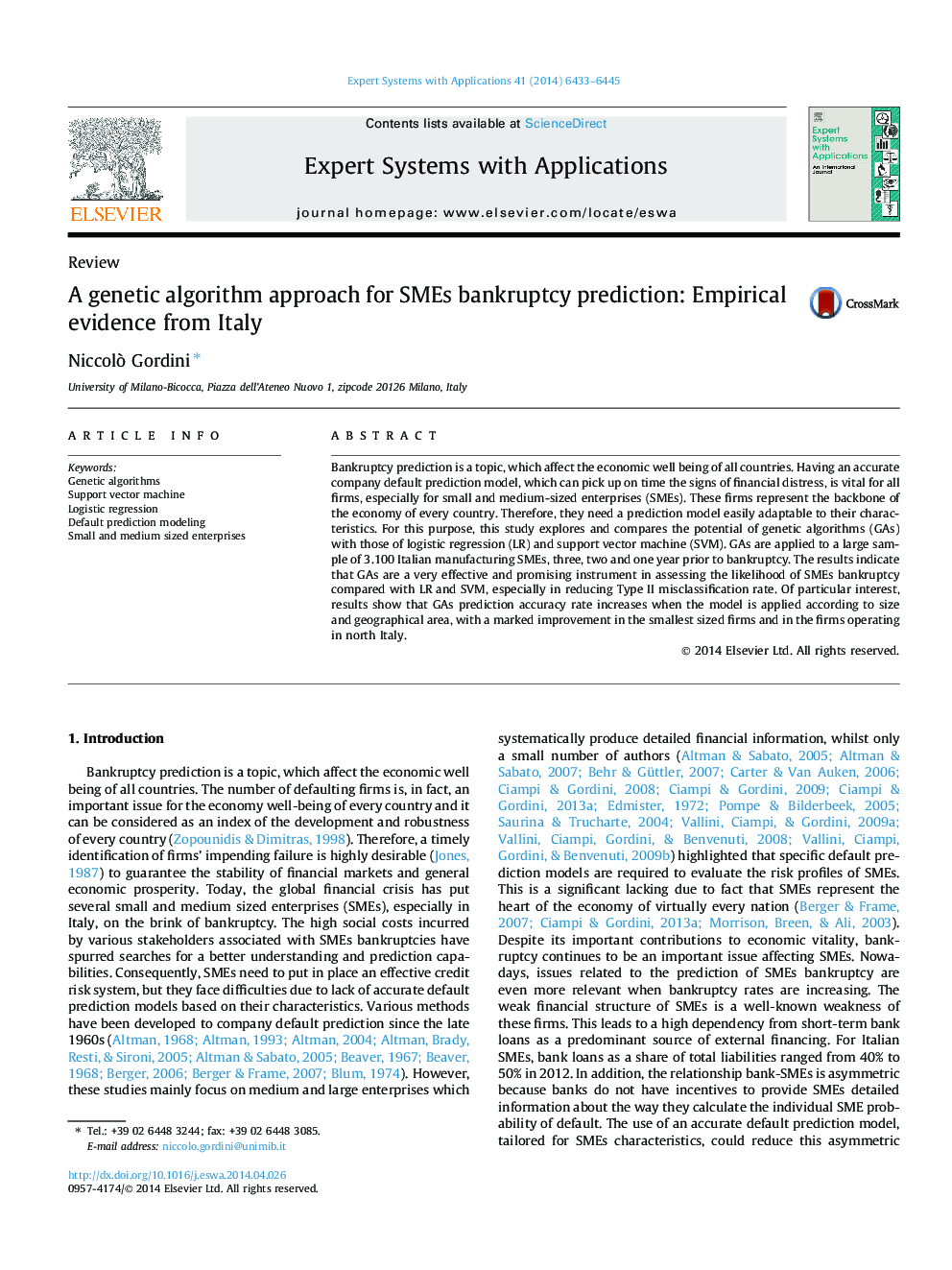
• GAs are a very promising method in SMEs default prediction analysis.
• GAs are capable of extracting rules that are easy to understand for users.
• GAs give a better SMEs default prediction accuracy rate compared with SVM and LR.
• GAs significantly reduce misclassification costs compared to SVM and LR.
• The prediction accuracy rate of GAs is markedly higher for the smallest sized firms and in the firms operating in the north.
Bankruptcy prediction is a topic, which affect the economic well being of all countries. Having an accurate company default prediction model, which can pick up on time the signs of financial distress, is vital for all firms, especially for small and medium-sized enterprises (SMEs). These firms represent the backbone of the economy of every country. Therefore, they need a prediction model easily adaptable to their characteristics. For this purpose, this study explores and compares the potential of genetic algorithms (GAs) with those of logistic regression (LR) and support vector machine (SVM). GAs are applied to a large sample of 3.100 Italian manufacturing SMEs, three, two and one year prior to bankruptcy. The results indicate that GAs are a very effective and promising instrument in assessing the likelihood of SMEs bankruptcy compared with LR and SVM, especially in reducing Type II misclassification rate. Of particular interest, results show that GAs prediction accuracy rate increases when the model is applied according to size and geographical area, with a marked improvement in the smallest sized firms and in the firms operating in north Italy.
Journal: Expert Systems with Applications - Volume 41, Issue 14, 15 October 2014, Pages 6433–6445