کد مقاله | کد نشریه | سال انتشار | مقاله انگلیسی | نسخه تمام متن |
---|---|---|---|---|
386205 | 660880 | 2010 | 7 صفحه PDF | دانلود رایگان |
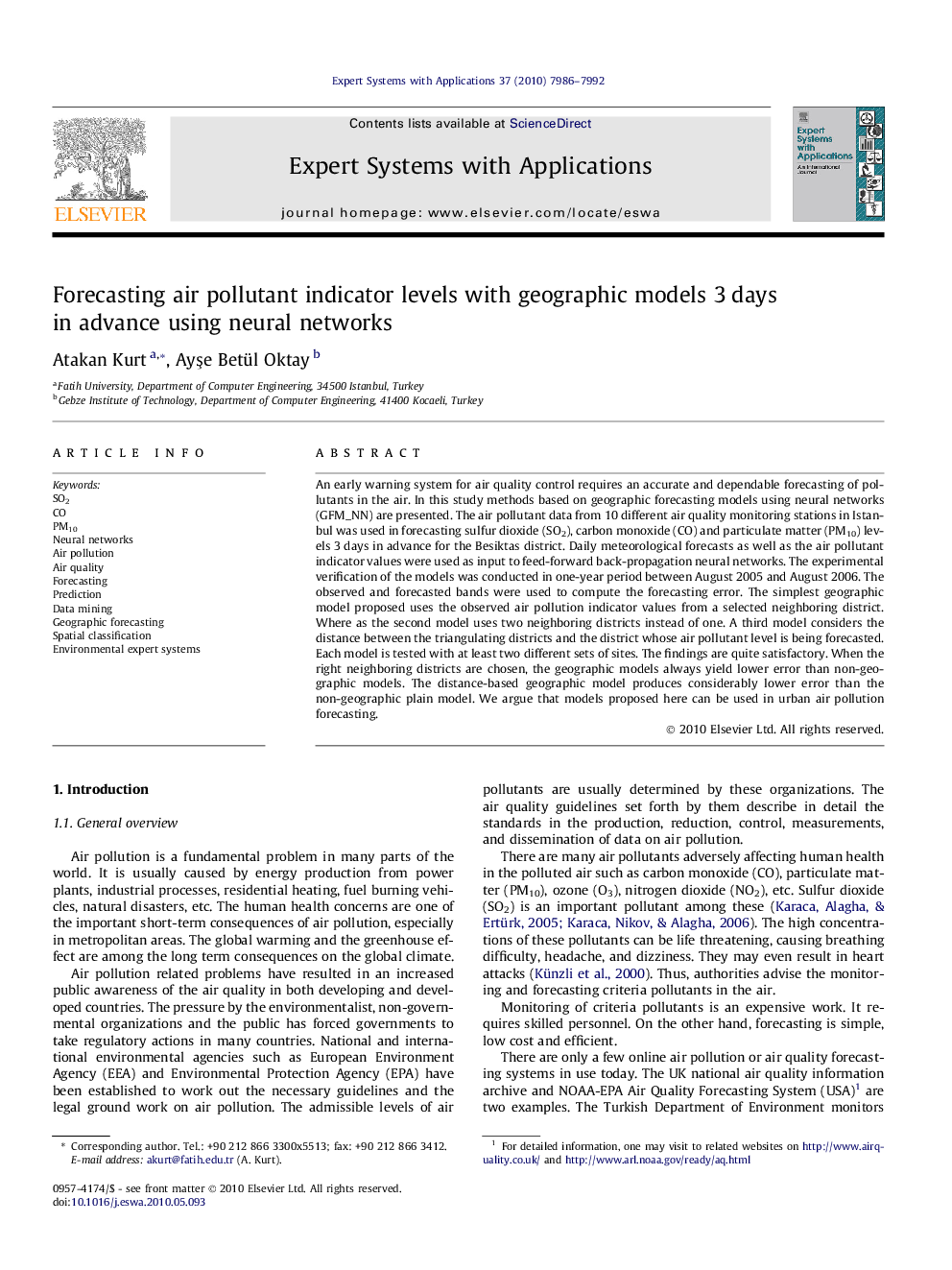
An early warning system for air quality control requires an accurate and dependable forecasting of pollutants in the air. In this study methods based on geographic forecasting models using neural networks (GFM_NN) are presented. The air pollutant data from 10 different air quality monitoring stations in Istanbul was used in forecasting sulfur dioxide (SO2), carbon monoxide (CO) and particulate matter (PM10) levels 3 days in advance for the Besiktas district. Daily meteorological forecasts as well as the air pollutant indicator values were used as input to feed-forward back-propagation neural networks. The experimental verification of the models was conducted in one-year period between August 2005 and August 2006. The observed and forecasted bands were used to compute the forecasting error. The simplest geographic model proposed uses the observed air pollution indicator values from a selected neighboring district. Where as the second model uses two neighboring districts instead of one. A third model considers the distance between the triangulating districts and the district whose air pollutant level is being forecasted. Each model is tested with at least two different sets of sites. The findings are quite satisfactory. When the right neighboring districts are chosen, the geographic models always yield lower error than non-geographic models. The distance-based geographic model produces considerably lower error than the non-geographic plain model. We argue that models proposed here can be used in urban air pollution forecasting.
Journal: Expert Systems with Applications - Volume 37, Issue 12, December 2010, Pages 7986–7992