کد مقاله | کد نشریه | سال انتشار | مقاله انگلیسی | نسخه تمام متن |
---|---|---|---|---|
391795 | 662001 | 2014 | 11 صفحه PDF | دانلود رایگان |
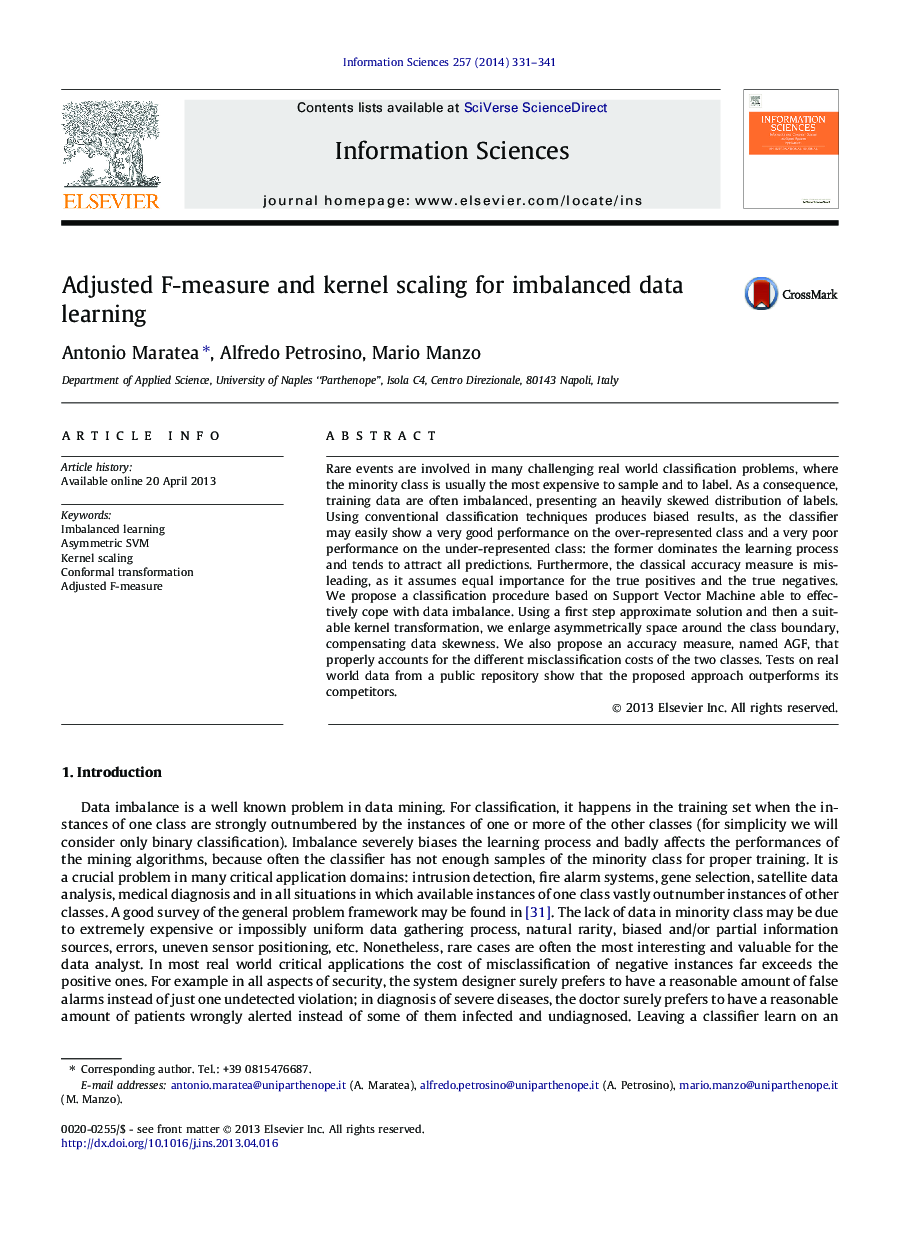
Rare events are involved in many challenging real world classification problems, where the minority class is usually the most expensive to sample and to label. As a consequence, training data are often imbalanced, presenting an heavily skewed distribution of labels. Using conventional classification techniques produces biased results, as the classifier may easily show a very good performance on the over-represented class and a very poor performance on the under-represented class: the former dominates the learning process and tends to attract all predictions. Furthermore, the classical accuracy measure is misleading, as it assumes equal importance for the true positives and the true negatives. We propose a classification procedure based on Support Vector Machine able to effectively cope with data imbalance. Using a first step approximate solution and then a suitable kernel transformation, we enlarge asymmetrically space around the class boundary, compensating data skewness. We also propose an accuracy measure, named AGF, that properly accounts for the different misclassification costs of the two classes. Tests on real world data from a public repository show that the proposed approach outperforms its competitors.
Journal: Information Sciences - Volume 257, 1 February 2014, Pages 331–341