کد مقاله | کد نشریه | سال انتشار | مقاله انگلیسی | نسخه تمام متن |
---|---|---|---|---|
393113 | 665571 | 2013 | 15 صفحه PDF | دانلود رایگان |
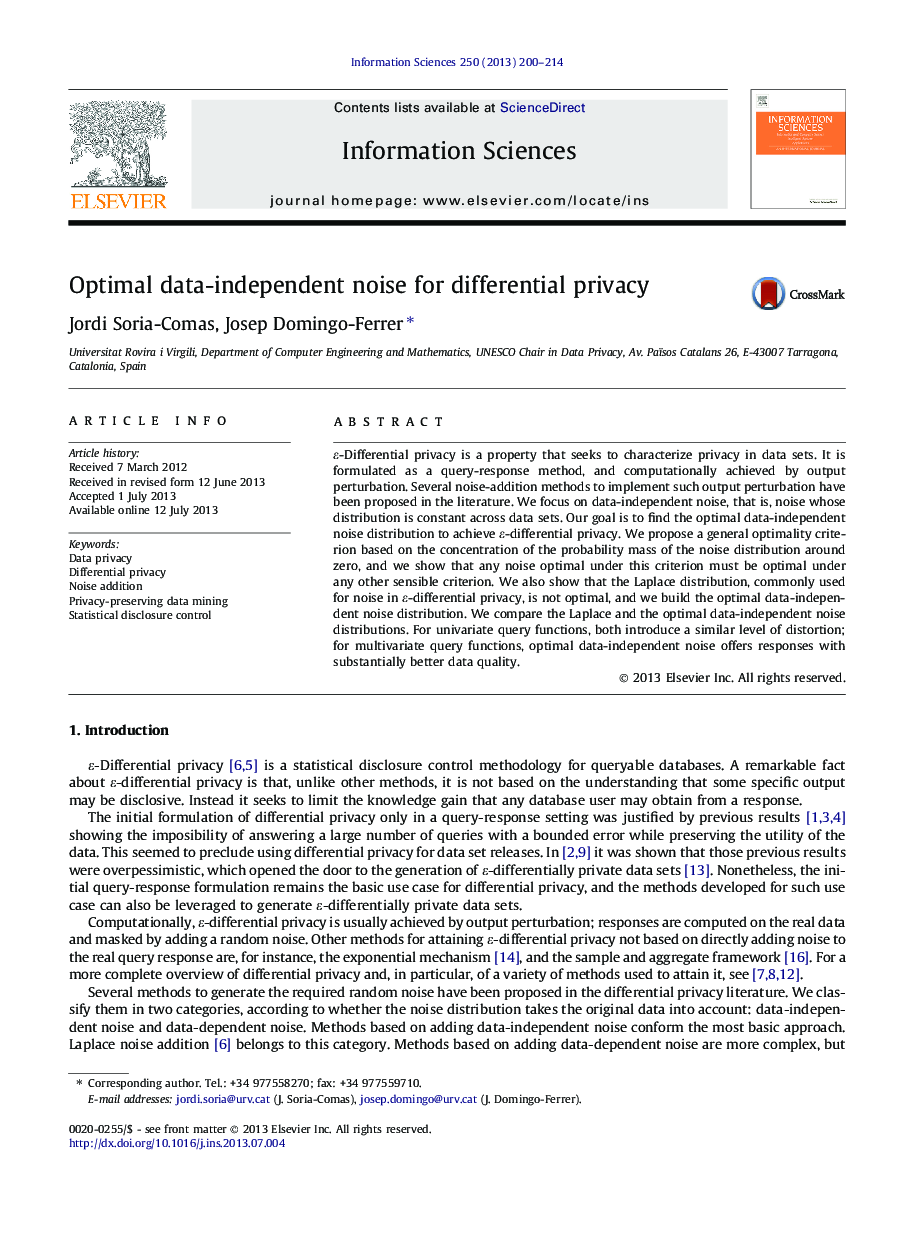
ε-Differential privacy is a property that seeks to characterize privacy in data sets. It is formulated as a query-response method, and computationally achieved by output perturbation. Several noise-addition methods to implement such output perturbation have been proposed in the literature. We focus on data-independent noise, that is, noise whose distribution is constant across data sets. Our goal is to find the optimal data-independent noise distribution to achieve ε-differential privacy. We propose a general optimality criterion based on the concentration of the probability mass of the noise distribution around zero, and we show that any noise optimal under this criterion must be optimal under any other sensible criterion. We also show that the Laplace distribution, commonly used for noise in ε-differential privacy, is not optimal, and we build the optimal data-independent noise distribution. We compare the Laplace and the optimal data-independent noise distributions. For univariate query functions, both introduce a similar level of distortion; for multivariate query functions, optimal data-independent noise offers responses with substantially better data quality.
Journal: Information Sciences - Volume 250, 20 November 2013, Pages 200–214