کد مقاله | کد نشریه | سال انتشار | مقاله انگلیسی | نسخه تمام متن |
---|---|---|---|---|
393183 | 665576 | 2013 | 14 صفحه PDF | دانلود رایگان |
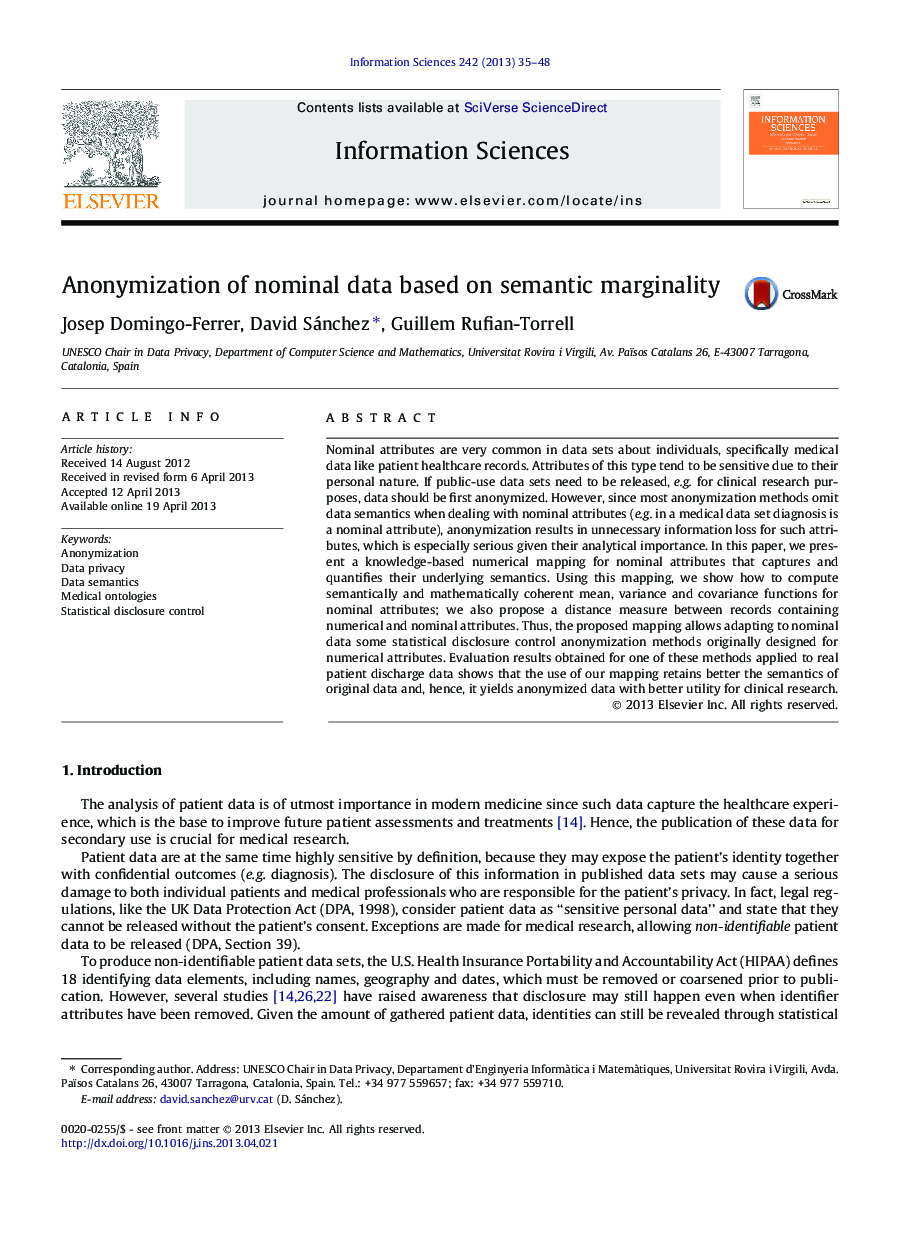
• A knowledge-based numerical mapping for nominal attributes is presented.
• Using this mapping, semantically-coherent means, variances, covariance, and distance functions are proposed.
• Using these functions, anonymization methods can be coherently applied to nominal data.
• As a result, anonymization of patient record data retains their semantics better.
• Hence, our proposal yields anonymized results with better utility for clinical research.
Nominal attributes are very common in data sets about individuals, specifically medical data like patient healthcare records. Attributes of this type tend to be sensitive due to their personal nature. If public-use data sets need to be released, e.g. for clinical research purposes, data should be first anonymized. However, since most anonymization methods omit data semantics when dealing with nominal attributes (e.g. in a medical data set diagnosis is a nominal attribute), anonymization results in unnecessary information loss for such attributes, which is especially serious given their analytical importance. In this paper, we present a knowledge-based numerical mapping for nominal attributes that captures and quantifies their underlying semantics. Using this mapping, we show how to compute semantically and mathematically coherent mean, variance and covariance functions for nominal attributes; we also propose a distance measure between records containing numerical and nominal attributes. Thus, the proposed mapping allows adapting to nominal data some statistical disclosure control anonymization methods originally designed for numerical attributes. Evaluation results obtained for one of these methods applied to real patient discharge data shows that the use of our mapping retains better the semantics of original data and, hence, it yields anonymized data with better utility for clinical research.
Journal: Information Sciences - Volume 242, 1 September 2013, Pages 35–48