کد مقاله | کد نشریه | سال انتشار | مقاله انگلیسی | نسخه تمام متن |
---|---|---|---|---|
393762 | 665684 | 2011 | 14 صفحه PDF | دانلود رایگان |
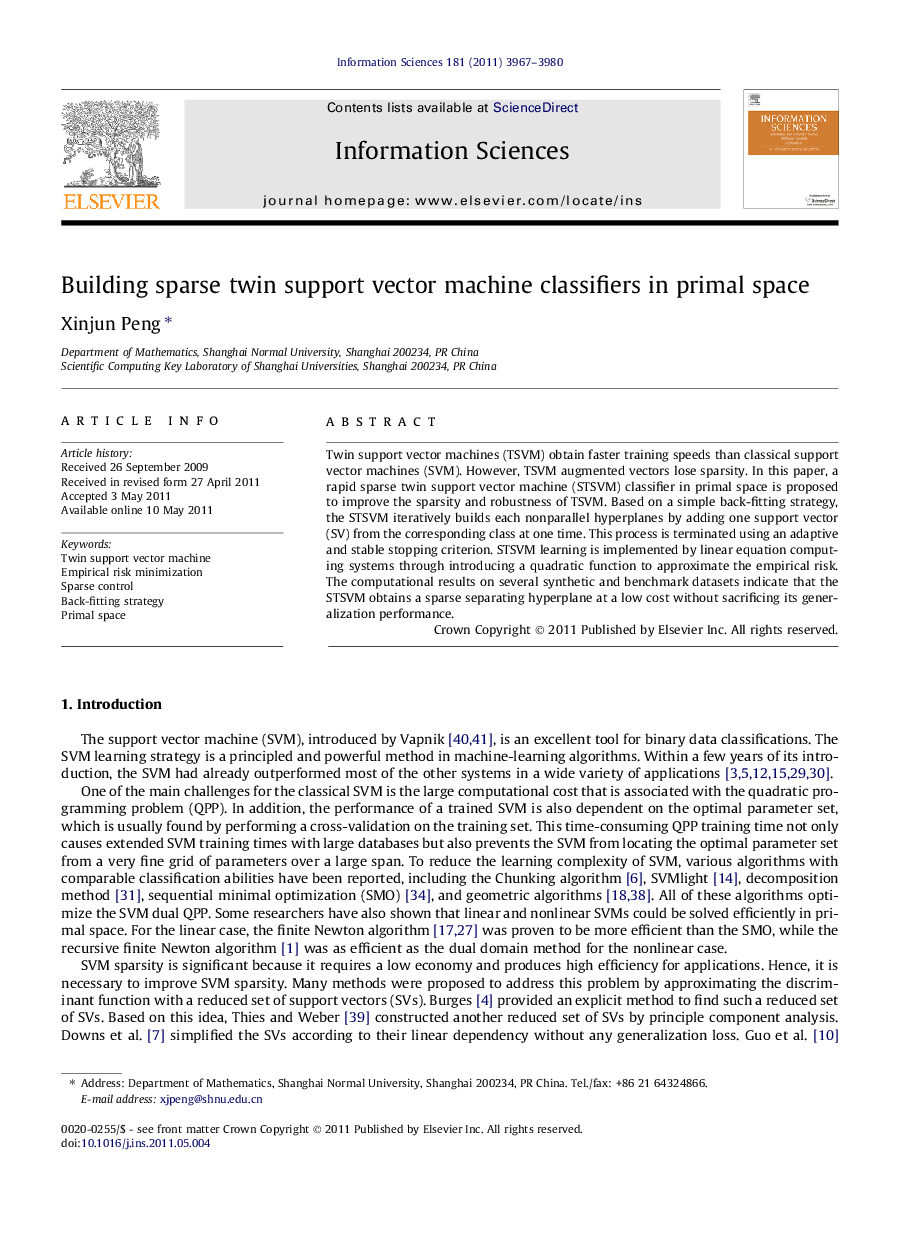
Twin support vector machines (TSVM) obtain faster training speeds than classical support vector machines (SVM). However, TSVM augmented vectors lose sparsity. In this paper, a rapid sparse twin support vector machine (STSVM) classifier in primal space is proposed to improve the sparsity and robustness of TSVM. Based on a simple back-fitting strategy, the STSVM iteratively builds each nonparallel hyperplanes by adding one support vector (SV) from the corresponding class at one time. This process is terminated using an adaptive and stable stopping criterion. STSVM learning is implemented by linear equation computing systems through introducing a quadratic function to approximate the empirical risk. The computational results on several synthetic and benchmark datasets indicate that the STSVM obtains a sparse separating hyperplane at a low cost without sacrificing its generalization performance.
Journal: Information Sciences - Volume 181, Issue 18, 15 September 2011, Pages 3967–3980