کد مقاله | کد نشریه | سال انتشار | مقاله انگلیسی | نسخه تمام متن |
---|---|---|---|---|
394023 | 665715 | 2013 | 18 صفحه PDF | دانلود رایگان |
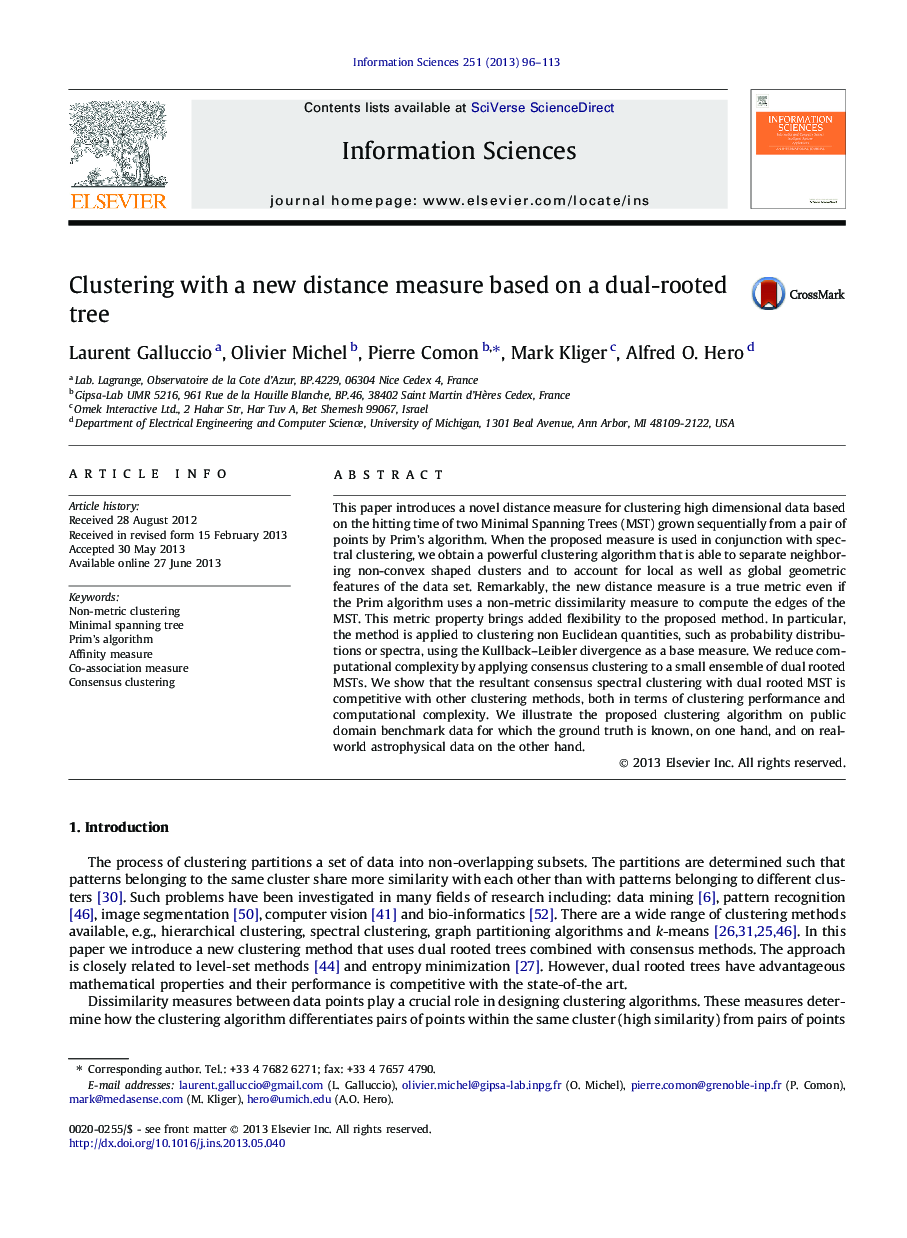
• New dual rooted tree based metric is introduced.
• Shown to be a true distance, whatever the metrics used to grow the MST.
• Emphasis put on pseudo metrics like information divergences, improving clustering.
• Co-association measures allow to keep computional load acceptable.
• Illustrated on various datasets, including real astrophysical data.
This paper introduces a novel distance measure for clustering high dimensional data based on the hitting time of two Minimal Spanning Trees (MST) grown sequentially from a pair of points by Prim’s algorithm. When the proposed measure is used in conjunction with spectral clustering, we obtain a powerful clustering algorithm that is able to separate neighboring non-convex shaped clusters and to account for local as well as global geometric features of the data set. Remarkably, the new distance measure is a true metric even if the Prim algorithm uses a non-metric dissimilarity measure to compute the edges of the MST. This metric property brings added flexibility to the proposed method. In particular, the method is applied to clustering non Euclidean quantities, such as probability distributions or spectra, using the Kullback–Leibler divergence as a base measure. We reduce computational complexity by applying consensus clustering to a small ensemble of dual rooted MSTs. We show that the resultant consensus spectral clustering with dual rooted MST is competitive with other clustering methods, both in terms of clustering performance and computational complexity. We illustrate the proposed clustering algorithm on public domain benchmark data for which the ground truth is known, on one hand, and on real-world astrophysical data on the other hand.
Journal: Information Sciences - Volume 251, 1 December 2013, Pages 96–113