کد مقاله | کد نشریه | سال انتشار | مقاله انگلیسی | نسخه تمام متن |
---|---|---|---|---|
395291 | 665945 | 2010 | 17 صفحه PDF | دانلود رایگان |
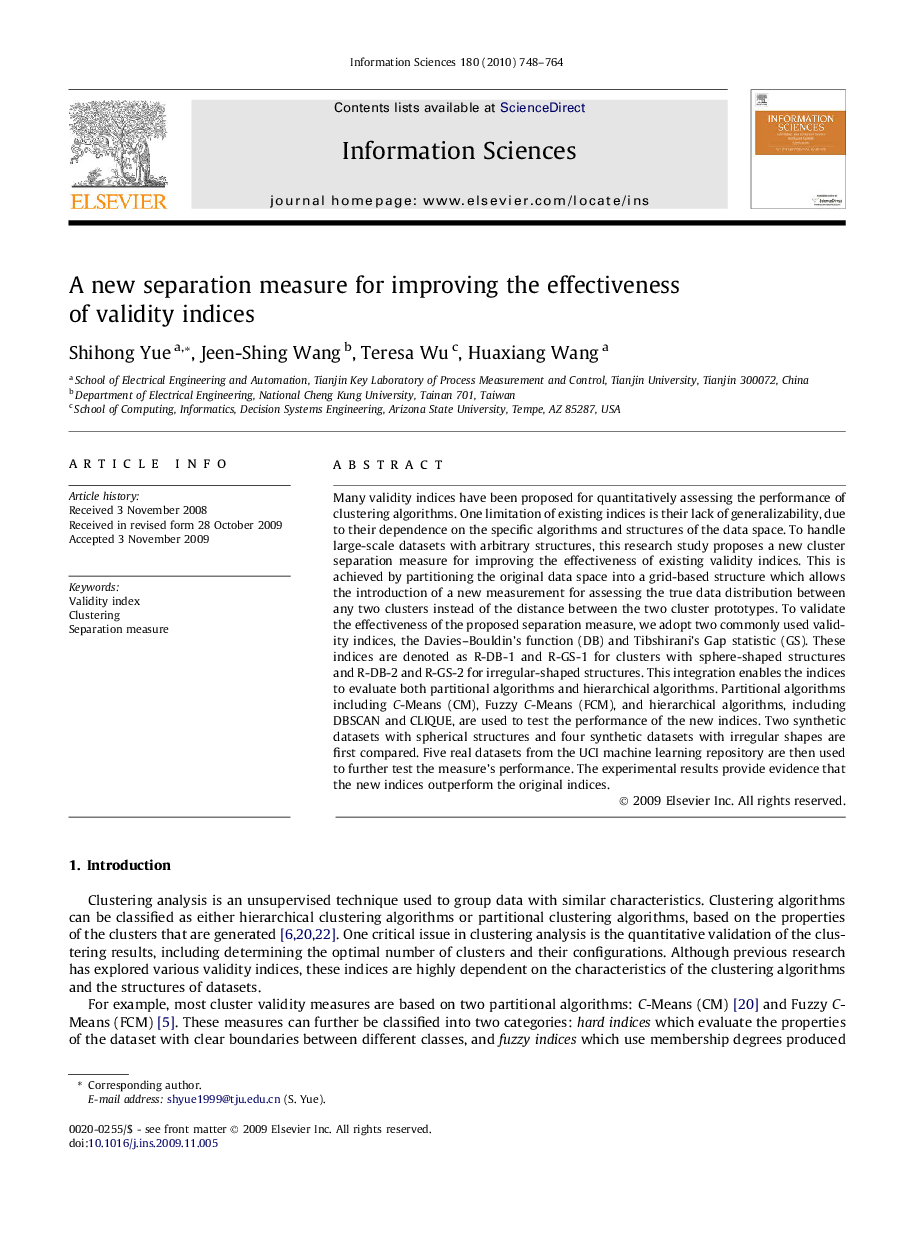
Many validity indices have been proposed for quantitatively assessing the performance of clustering algorithms. One limitation of existing indices is their lack of generalizability, due to their dependence on the specific algorithms and structures of the data space. To handle large-scale datasets with arbitrary structures, this research study proposes a new cluster separation measure for improving the effectiveness of existing validity indices. This is achieved by partitioning the original data space into a grid-based structure which allows the introduction of a new measurement for assessing the true data distribution between any two clusters instead of the distance between the two cluster prototypes. To validate the effectiveness of the proposed separation measure, we adopt two commonly used validity indices, the Davies–Bouldin’s function (DB) and Tibshirani’s Gap statistic (GS). These indices are denoted as R-DB-1 and R-GS-1 for clusters with sphere-shaped structures and R-DB-2 and R-GS-2 for irregular-shaped structures. This integration enables the indices to evaluate both partitional algorithms and hierarchical algorithms. Partitional algorithms including C-Means (CM), Fuzzy C-Means (FCM), and hierarchical algorithms, including DBSCAN and CLIQUE, are used to test the performance of the new indices. Two synthetic datasets with spherical structures and four synthetic datasets with irregular shapes are first compared. Five real datasets from the UCI machine learning repository are then used to further test the measure’s performance. The experimental results provide evidence that the new indices outperform the original indices.
Journal: Information Sciences - Volume 180, Issue 5, 1 March 2010, Pages 748–764