کد مقاله | کد نشریه | سال انتشار | مقاله انگلیسی | نسخه تمام متن |
---|---|---|---|---|
396036 | 666107 | 2007 | 19 صفحه PDF | دانلود رایگان |
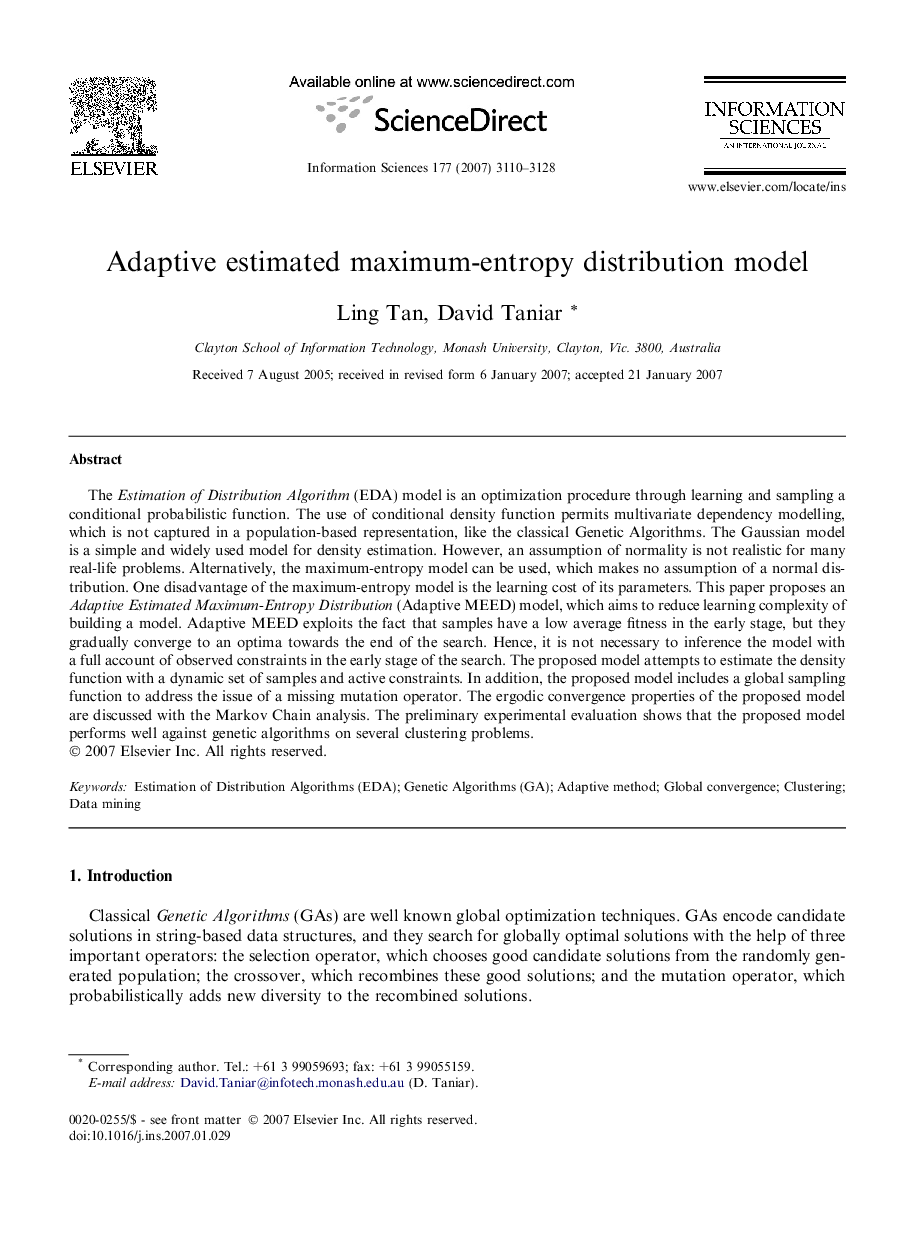
The Estimation of Distribution Algorithm (EDA) model is an optimization procedure through learning and sampling a conditional probabilistic function. The use of conditional density function permits multivariate dependency modelling, which is not captured in a population-based representation, like the classical Genetic Algorithms. The Gaussian model is a simple and widely used model for density estimation. However, an assumption of normality is not realistic for many real-life problems. Alternatively, the maximum-entropy model can be used, which makes no assumption of a normal distribution. One disadvantage of the maximum-entropy model is the learning cost of its parameters. This paper proposes an Adaptive Estimated Maximum-Entropy Distribution (Adaptive MEED) model, which aims to reduce learning complexity of building a model. Adaptive MEED exploits the fact that samples have a low average fitness in the early stage, but they gradually converge to an optima towards the end of the search. Hence, it is not necessary to inference the model with a full account of observed constraints in the early stage of the search. The proposed model attempts to estimate the density function with a dynamic set of samples and active constraints. In addition, the proposed model includes a global sampling function to address the issue of a missing mutation operator. The ergodic convergence properties of the proposed model are discussed with the Markov Chain analysis. The preliminary experimental evaluation shows that the proposed model performs well against genetic algorithms on several clustering problems.
Journal: Information Sciences - Volume 177, Issue 15, 1 August 2007, Pages 3110–3128