کد مقاله | کد نشریه | سال انتشار | مقاله انگلیسی | نسخه تمام متن |
---|---|---|---|---|
409659 | 679083 | 2013 | 14 صفحه PDF | دانلود رایگان |
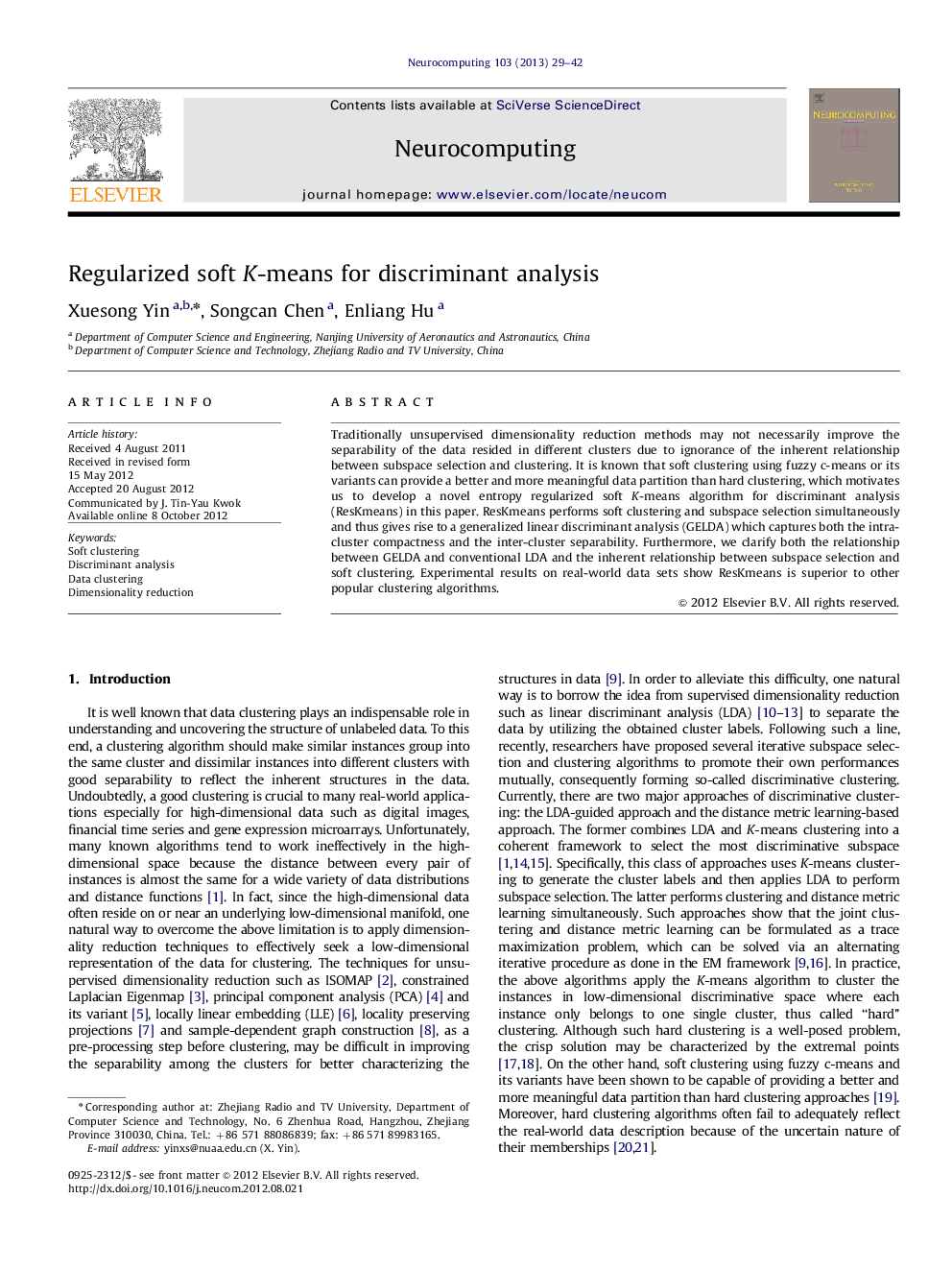
Traditionally unsupervised dimensionality reduction methods may not necessarily improve the separability of the data resided in different clusters due to ignorance of the inherent relationship between subspace selection and clustering. It is known that soft clustering using fuzzy c-means or its variants can provide a better and more meaningful data partition than hard clustering, which motivates us to develop a novel entropy regularized soft K-means algorithm for discriminant analysis (ResKmeans) in this paper. ResKmeans performs soft clustering and subspace selection simultaneously and thus gives rise to a generalized linear discriminant analysis (GELDA) which captures both the intra-cluster compactness and the inter-cluster separability. Furthermore, we clarify both the relationship between GELDA and conventional LDA and the inherent relationship between subspace selection and soft clustering. Experimental results on real-world data sets show ResKmeans is superior to other popular clustering algorithms.
Journal: Neurocomputing - Volume 103, 1 March 2013, Pages 29–42