کد مقاله | کد نشریه | سال انتشار | مقاله انگلیسی | نسخه تمام متن |
---|---|---|---|---|
410262 | 679132 | 2013 | 13 صفحه PDF | دانلود رایگان |
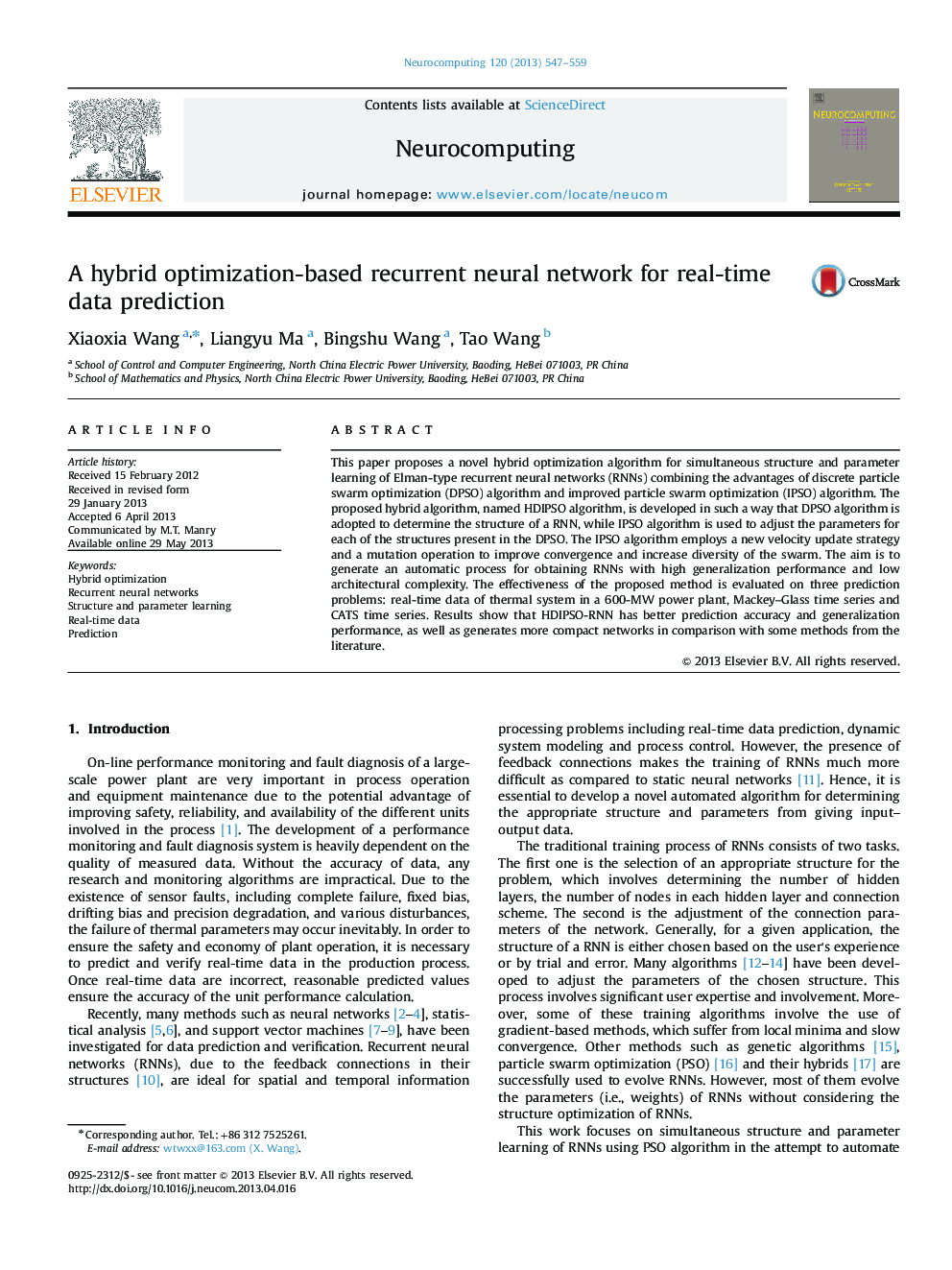
This paper proposes a novel hybrid optimization algorithm for simultaneous structure and parameter learning of Elman-type recurrent neural networks (RNNs) combining the advantages of discrete particle swarm optimization (DPSO) algorithm and improved particle swarm optimization (IPSO) algorithm. The proposed hybrid algorithm, named HDIPSO algorithm, is developed in such a way that DPSO algorithm is adopted to determine the structure of a RNN, while IPSO algorithm is used to adjust the parameters for each of the structures present in the DPSO. The IPSO algorithm employs a new velocity update strategy and a mutation operation to improve convergence and increase diversity of the swarm. The aim is to generate an automatic process for obtaining RNNs with high generalization performance and low architectural complexity. The effectiveness of the proposed method is evaluated on three prediction problems: real-time data of thermal system in a 600-MW power plant, Mackey–Glass time series and CATS time series. Results show that HDIPSO-RNN has better prediction accuracy and generalization performance, as well as generates more compact networks in comparison with some methods from the literature.
Journal: Neurocomputing - Volume 120, 23 November 2013, Pages 547–559