کد مقاله | کد نشریه | سال انتشار | مقاله انگلیسی | نسخه تمام متن |
---|---|---|---|---|
412206 | 679619 | 2014 | 9 صفحه PDF | دانلود رایگان |
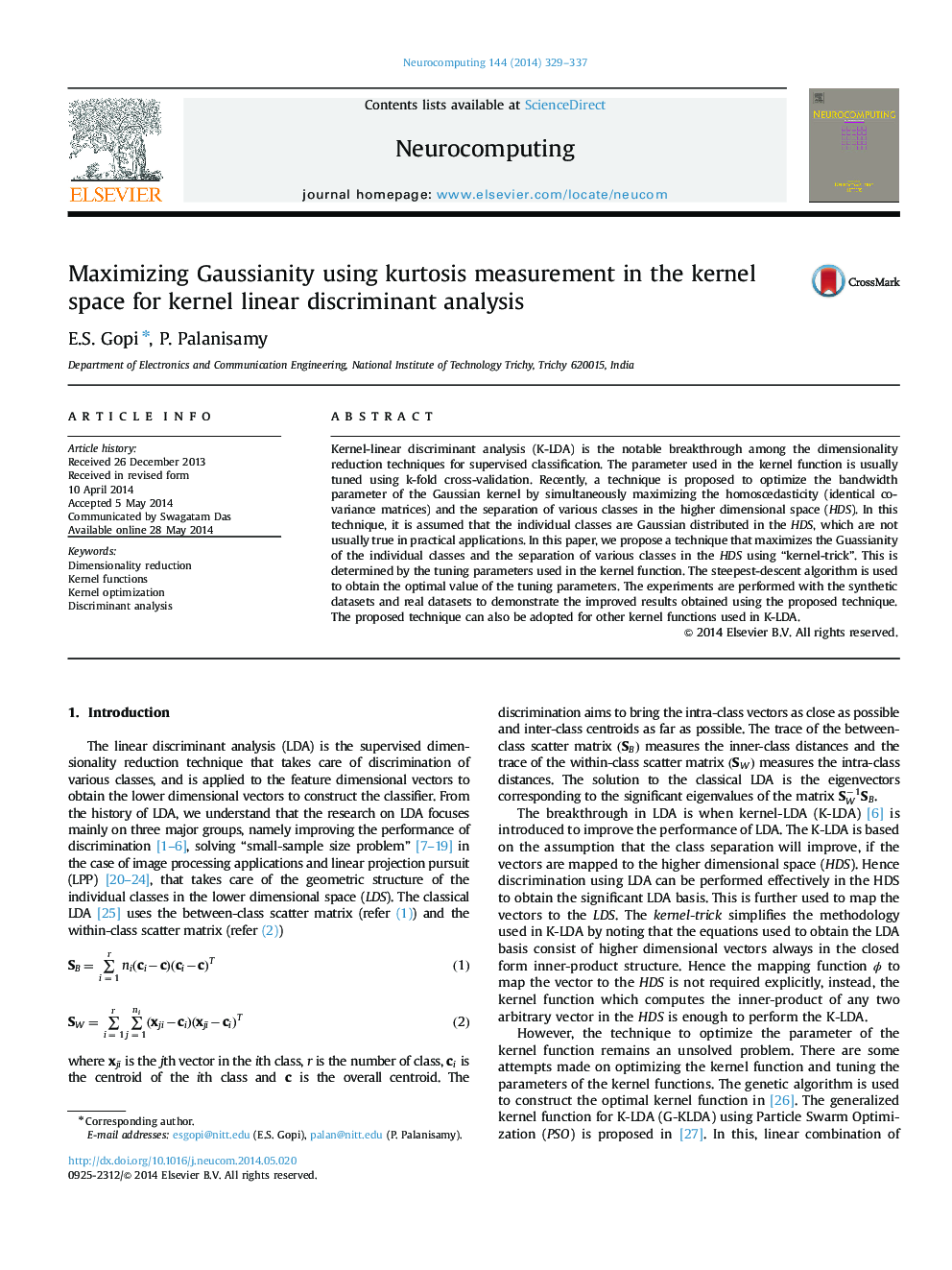
Kernel-linear discriminant analysis (K-LDA) is the notable breakthrough among the dimensionality reduction techniques for supervised classification. The parameter used in the kernel function is usually tuned using k-fold cross-validation. Recently, a technique is proposed to optimize the bandwidth parameter of the Gaussian kernel by simultaneously maximizing the homoscedasticity (identical co-variance matrices) and the separation of various classes in the higher dimensional space (HDS). In this technique, it is assumed that the individual classes are Gaussian distributed in the HDS, which are not usually true in practical applications. In this paper, we propose a technique that maximizes the Guassianity of the individual classes and the separation of various classes in the HDS using “kernel-trick”. This is determined by the tuning parameters used in the kernel function. The steepest-descent algorithm is used to obtain the optimal value of the tuning parameters. The experiments are performed with the synthetic datasets and real datasets to demonstrate the improved results obtained using the proposed technique. The proposed technique can also be adopted for other kernel functions used in K-LDA.
Journal: Neurocomputing - Volume 144, 20 November 2014, Pages 329–337