کد مقاله | کد نشریه | سال انتشار | مقاله انگلیسی | نسخه تمام متن |
---|---|---|---|---|
4460593 | 1621329 | 2008 | 10 صفحه PDF | دانلود رایگان |
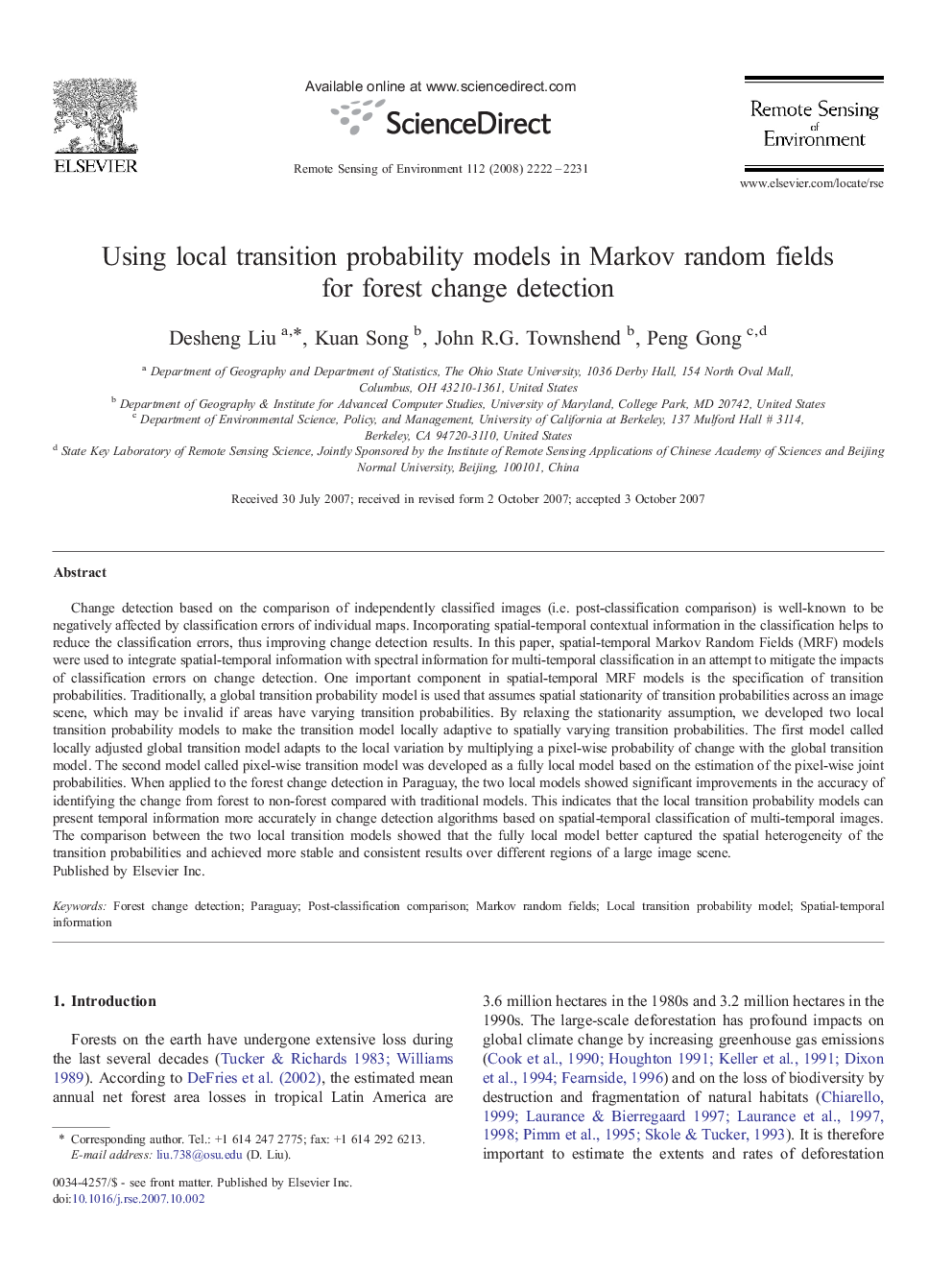
Change detection based on the comparison of independently classified images (i.e. post-classification comparison) is well-known to be negatively affected by classification errors of individual maps. Incorporating spatial-temporal contextual information in the classification helps to reduce the classification errors, thus improving change detection results. In this paper, spatial-temporal Markov Random Fields (MRF) models were used to integrate spatial-temporal information with spectral information for multi-temporal classification in an attempt to mitigate the impacts of classification errors on change detection. One important component in spatial-temporal MRF models is the specification of transition probabilities. Traditionally, a global transition probability model is used that assumes spatial stationarity of transition probabilities across an image scene, which may be invalid if areas have varying transition probabilities. By relaxing the stationarity assumption, we developed two local transition probability models to make the transition model locally adaptive to spatially varying transition probabilities. The first model called locally adjusted global transition model adapts to the local variation by multiplying a pixel-wise probability of change with the global transition model. The second model called pixel-wise transition model was developed as a fully local model based on the estimation of the pixel-wise joint probabilities. When applied to the forest change detection in Paraguay, the two local models showed significant improvements in the accuracy of identifying the change from forest to non-forest compared with traditional models. This indicates that the local transition probability models can present temporal information more accurately in change detection algorithms based on spatial-temporal classification of multi-temporal images. The comparison between the two local transition models showed that the fully local model better captured the spatial heterogeneity of the transition probabilities and achieved more stable and consistent results over different regions of a large image scene.
Journal: Remote Sensing of Environment - Volume 112, Issue 5, 15 May 2008, Pages 2222–2231