کد مقاله | کد نشریه | سال انتشار | مقاله انگلیسی | نسخه تمام متن |
---|---|---|---|---|
4464635 | 1621810 | 2016 | 15 صفحه PDF | دانلود رایگان |
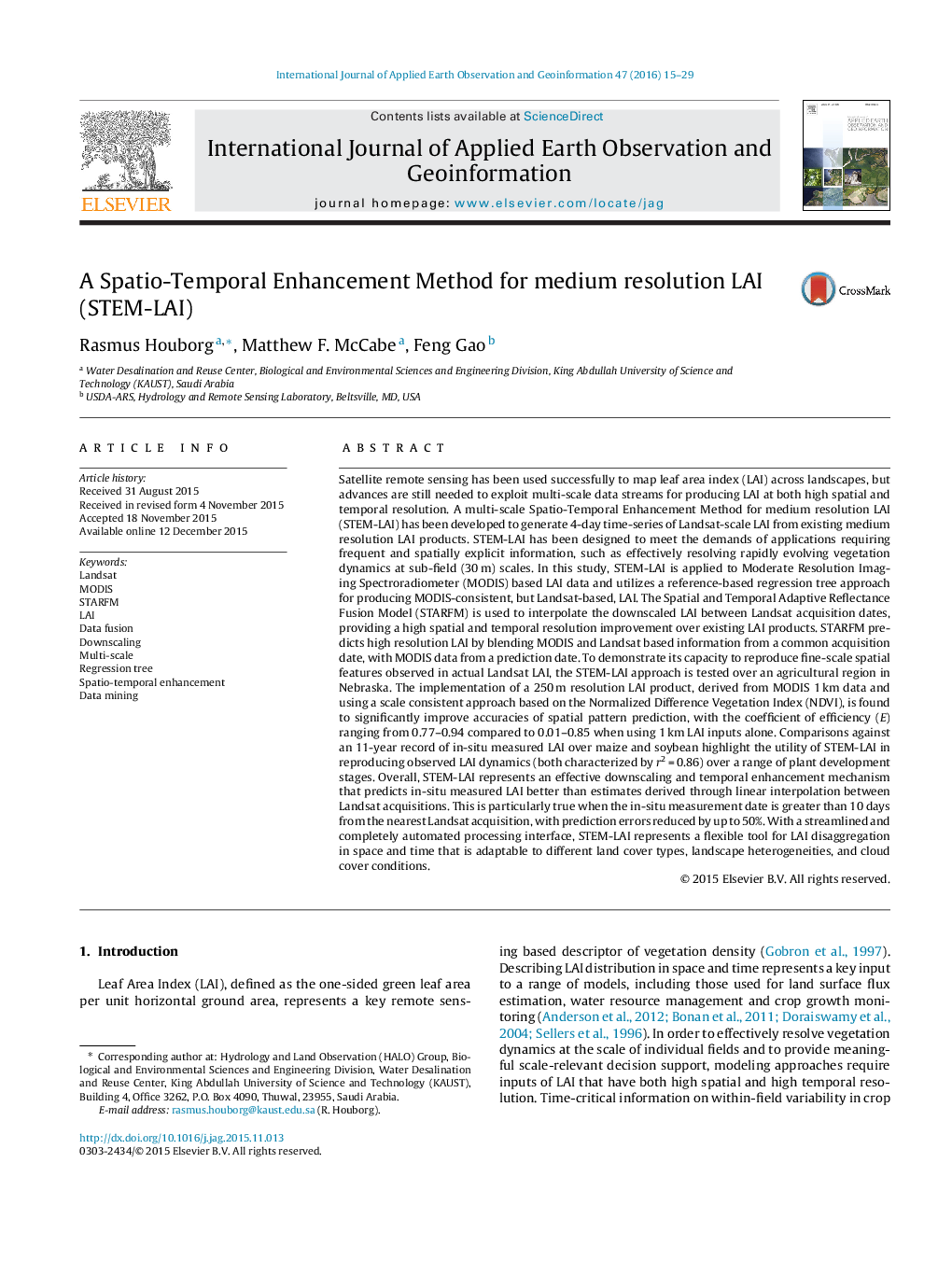
• STEM-LAI combines MODIS and Landsat based information.
• Data fusion enables high frequency (4-day) mapping of LAI at 30 m resolution.
• STEM-LAI is packaged within a fully automated processing infrastructure.
• STEM-LAI effectively downscales LAI over an agricultural region.
• STEM-LAI is adaptable to variations in cloud environment and landscape heterogeneity.
Satellite remote sensing has been used successfully to map leaf area index (LAI) across landscapes, but advances are still needed to exploit multi-scale data streams for producing LAI at both high spatial and temporal resolution. A multi-scale Spatio-Temporal Enhancement Method for medium resolution LAI (STEM-LAI) has been developed to generate 4-day time-series of Landsat-scale LAI from existing medium resolution LAI products. STEM-LAI has been designed to meet the demands of applications requiring frequent and spatially explicit information, such as effectively resolving rapidly evolving vegetation dynamics at sub-field (30 m) scales. In this study, STEM-LAI is applied to Moderate Resolution Imaging Spectroradiometer (MODIS) based LAI data and utilizes a reference-based regression tree approach for producing MODIS-consistent, but Landsat-based, LAI. The Spatial and Temporal Adaptive Reflectance Fusion Model (STARFM) is used to interpolate the downscaled LAI between Landsat acquisition dates, providing a high spatial and temporal resolution improvement over existing LAI products. STARFM predicts high resolution LAI by blending MODIS and Landsat based information from a common acquisition date, with MODIS data from a prediction date. To demonstrate its capacity to reproduce fine-scale spatial features observed in actual Landsat LAI, the STEM-LAI approach is tested over an agricultural region in Nebraska. The implementation of a 250 m resolution LAI product, derived from MODIS 1 km data and using a scale consistent approach based on the Normalized Difference Vegetation Index (NDVI), is found to significantly improve accuracies of spatial pattern prediction, with the coefficient of efficiency (E) ranging from 0.77–0.94 compared to 0.01–0.85 when using 1 km LAI inputs alone. Comparisons against an 11-year record of in-situ measured LAI over maize and soybean highlight the utility of STEM-LAI in reproducing observed LAI dynamics (both characterized by r2 = 0.86) over a range of plant development stages. Overall, STEM-LAI represents an effective downscaling and temporal enhancement mechanism that predicts in-situ measured LAI better than estimates derived through linear interpolation between Landsat acquisitions. This is particularly true when the in-situ measurement date is greater than 10 days from the nearest Landsat acquisition, with prediction errors reduced by up to 50%. With a streamlined and completely automated processing interface, STEM-LAI represents a flexible tool for LAI disaggregation in space and time that is adaptable to different land cover types, landscape heterogeneities, and cloud cover conditions.
Journal: International Journal of Applied Earth Observation and Geoinformation - Volume 47, May 2016, Pages 15–29