کد مقاله | کد نشریه | سال انتشار | مقاله انگلیسی | نسخه تمام متن |
---|---|---|---|---|
4549208 | 1627353 | 2007 | 18 صفحه PDF | دانلود رایگان |
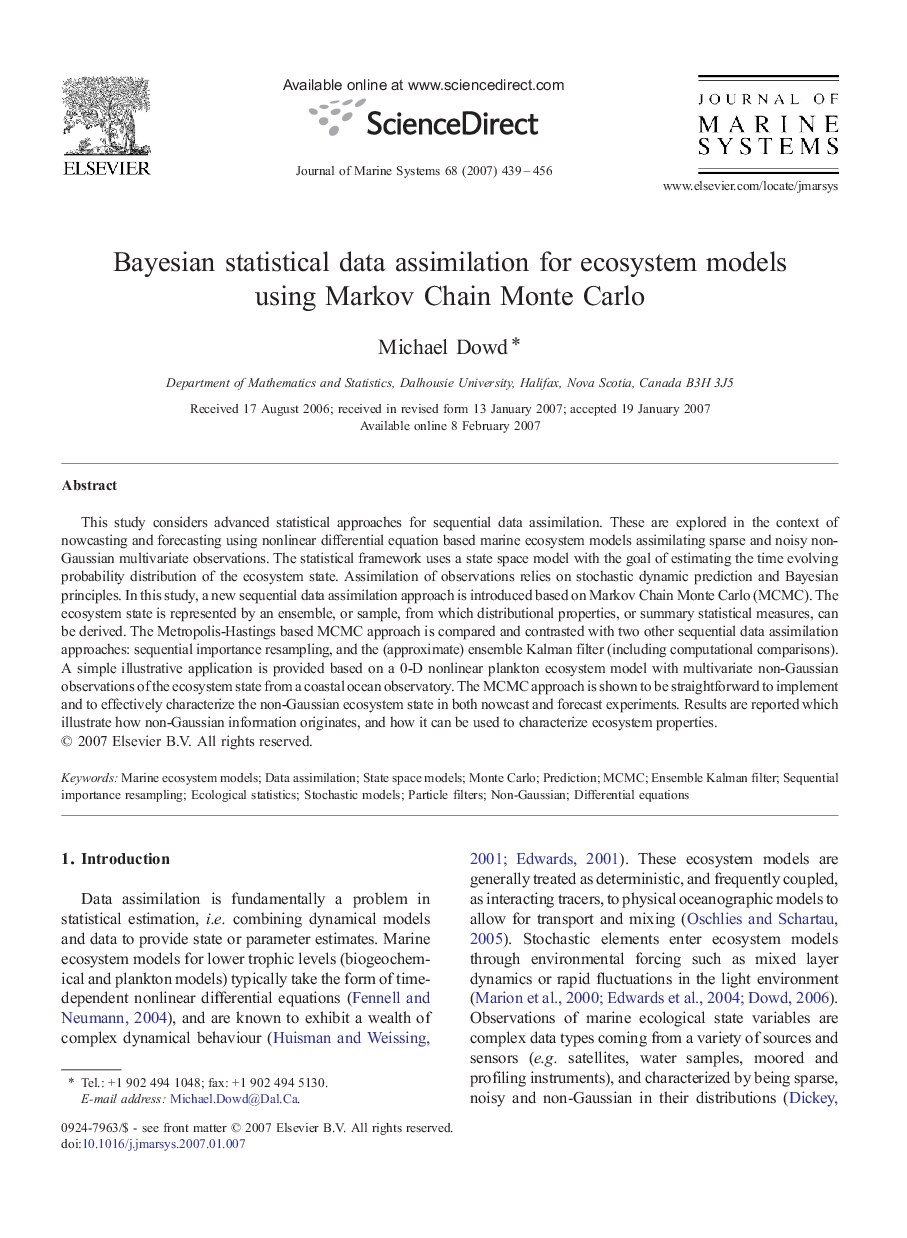
This study considers advanced statistical approaches for sequential data assimilation. These are explored in the context of nowcasting and forecasting using nonlinear differential equation based marine ecosystem models assimilating sparse and noisy non-Gaussian multivariate observations. The statistical framework uses a state space model with the goal of estimating the time evolving probability distribution of the ecosystem state. Assimilation of observations relies on stochastic dynamic prediction and Bayesian principles. In this study, a new sequential data assimilation approach is introduced based on Markov Chain Monte Carlo (MCMC). The ecosystem state is represented by an ensemble, or sample, from which distributional properties, or summary statistical measures, can be derived. The Metropolis-Hastings based MCMC approach is compared and contrasted with two other sequential data assimilation approaches: sequential importance resampling, and the (approximate) ensemble Kalman filter (including computational comparisons). A simple illustrative application is provided based on a 0-D nonlinear plankton ecosystem model with multivariate non-Gaussian observations of the ecosystem state from a coastal ocean observatory. The MCMC approach is shown to be straightforward to implement and to effectively characterize the non-Gaussian ecosystem state in both nowcast and forecast experiments. Results are reported which illustrate how non-Gaussian information originates, and how it can be used to characterize ecosystem properties.
Journal: Journal of Marine Systems - Volume 68, Issues 3–4, December 2007, Pages 439–456