کد مقاله | کد نشریه | سال انتشار | مقاله انگلیسی | نسخه تمام متن |
---|---|---|---|---|
4571688 | 1629248 | 2013 | 9 صفحه PDF | دانلود رایگان |
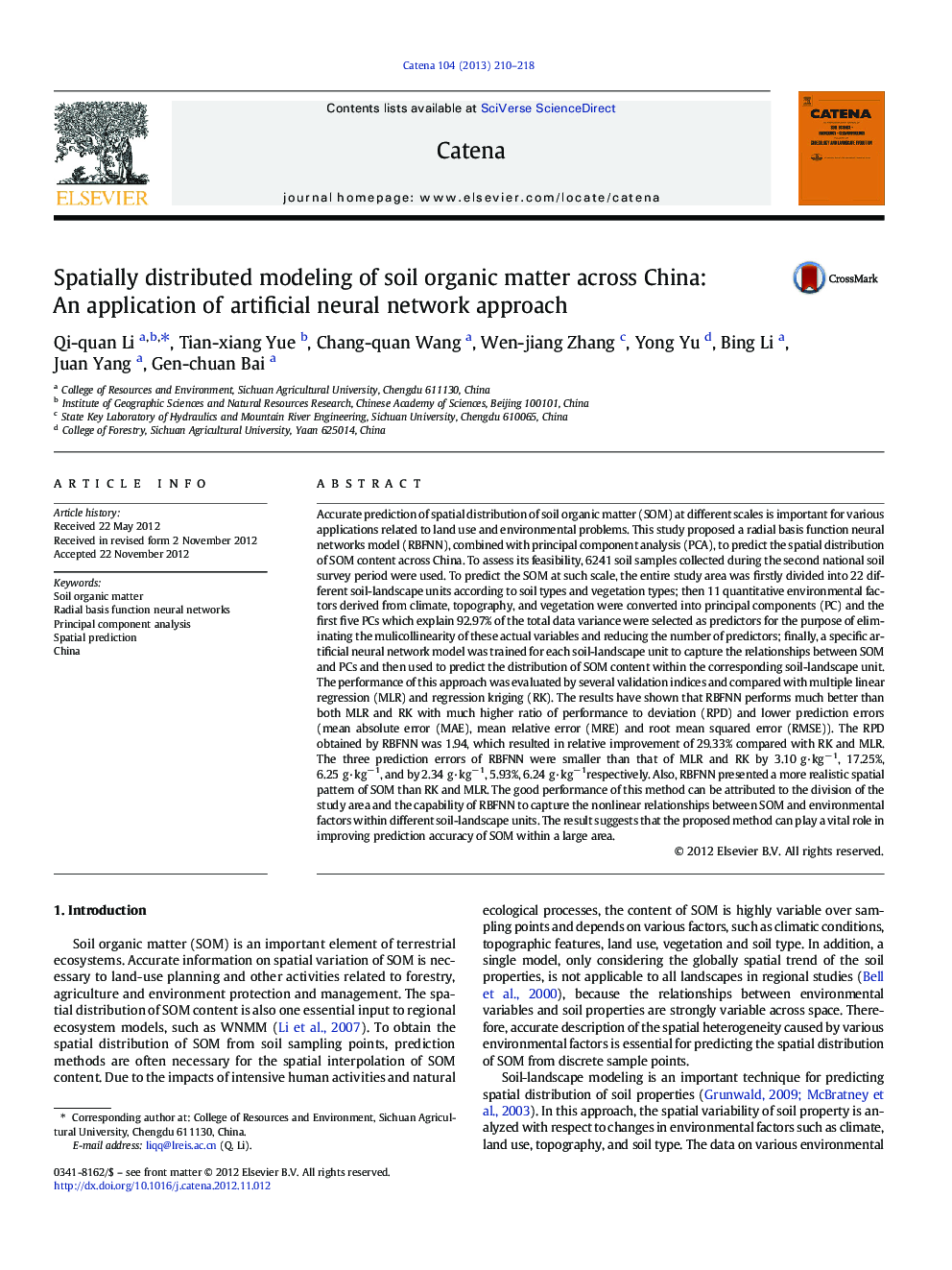
Accurate prediction of spatial distribution of soil organic matter (SOM) at different scales is important for various applications related to land use and environmental problems. This study proposed a radial basis function neural networks model (RBFNN), combined with principal component analysis (PCA), to predict the spatial distribution of SOM content across China. To assess its feasibility, 6241 soil samples collected during the second national soil survey period were used. To predict the SOM at such scale, the entire study area was firstly divided into 22 different soil-landscape units according to soil types and vegetation types; then 11 quantitative environmental factors derived from climate, topography, and vegetation were converted into principal components (PC) and the first five PCs which explain 92.97% of the total data variance were selected as predictors for the purpose of eliminating the mulicollinearity of these actual variables and reducing the number of predictors; finally, a specific artificial neural network model was trained for each soil-landscape unit to capture the relationships between SOM and PCs and then used to predict the distribution of SOM content within the corresponding soil-landscape unit. The performance of this approach was evaluated by several validation indices and compared with multiple linear regression (MLR) and regression kriging (RK). The results have shown that RBFNN performs much better than both MLR and RK with much higher ratio of performance to deviation (RPD) and lower prediction errors (mean absolute error (MAE), mean relative error (MRE) and root mean squared error (RMSE)). The RPD obtained by RBFNN was 1.94, which resulted in relative improvement of 29.33% compared with RK and MLR. The three prediction errors of RBFNN were smaller than that of MLR and RK by 3.10 g·kg− 1, 17.25%, 6.25 g·kg− 1, and by 2.34 g·kg− 1, 5.93%, 6.24 g·kg− 1respectively. Also, RBFNN presented a more realistic spatial pattern of SOM than RK and MLR. The good performance of this method can be attributed to the division of the study area and the capability of RBFNN to capture the nonlinear relationships between SOM and environmental factors within different soil-landscape units. The result suggests that the proposed method can play a vital role in improving prediction accuracy of SOM within a large area.
► An artificial neural network based approach was proposed for predicting soil organic matter across China.
► This approach obtained lower prediction errors than multiple linear regression and regression kriging.
► This approach also produced a more realistic spatial pattern of soil organic matter.
► The proposed method could be used for predicting soil properties over large area.
Journal: CATENA - Volume 104, May 2013, Pages 210–218