کد مقاله | کد نشریه | سال انتشار | مقاله انگلیسی | نسخه تمام متن |
---|---|---|---|---|
460288 | 696322 | 2011 | 9 صفحه PDF | دانلود رایگان |
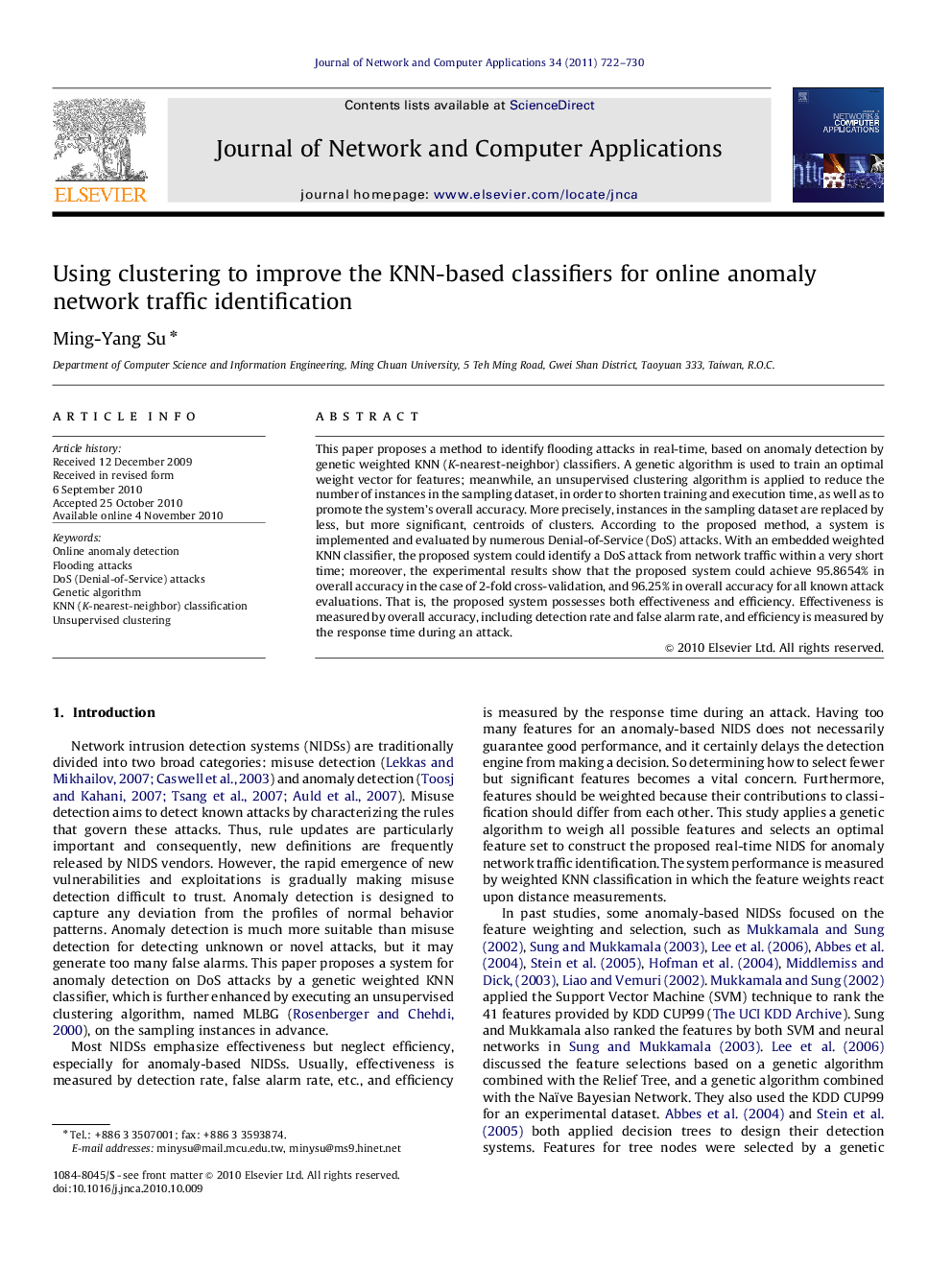
This paper proposes a method to identify flooding attacks in real-time, based on anomaly detection by genetic weighted KNN (K-nearest-neighbor) classifiers. A genetic algorithm is used to train an optimal weight vector for features; meanwhile, an unsupervised clustering algorithm is applied to reduce the number of instances in the sampling dataset, in order to shorten training and execution time, as well as to promote the system’s overall accuracy. More precisely, instances in the sampling dataset are replaced by less, but more significant, centroids of clusters. According to the proposed method, a system is implemented and evaluated by numerous Denial-of-Service (DoS) attacks. With an embedded weighted KNN classifier, the proposed system could identify a DoS attack from network traffic within a very short time; moreover, the experimental results show that the proposed system could achieve 95.8654% in overall accuracy in the case of 2-fold cross-validation, and 96.25% in overall accuracy for all known attack evaluations. That is, the proposed system possesses both effectiveness and efficiency. Effectiveness is measured by overall accuracy, including detection rate and false alarm rate, and efficiency is measured by the response time during an attack.
Journal: Journal of Network and Computer Applications - Volume 34, Issue 2, March 2011, Pages 722–730