کد مقاله | کد نشریه | سال انتشار | مقاله انگلیسی | نسخه تمام متن |
---|---|---|---|---|
480194 | 1446089 | 2012 | 14 صفحه PDF | دانلود رایگان |
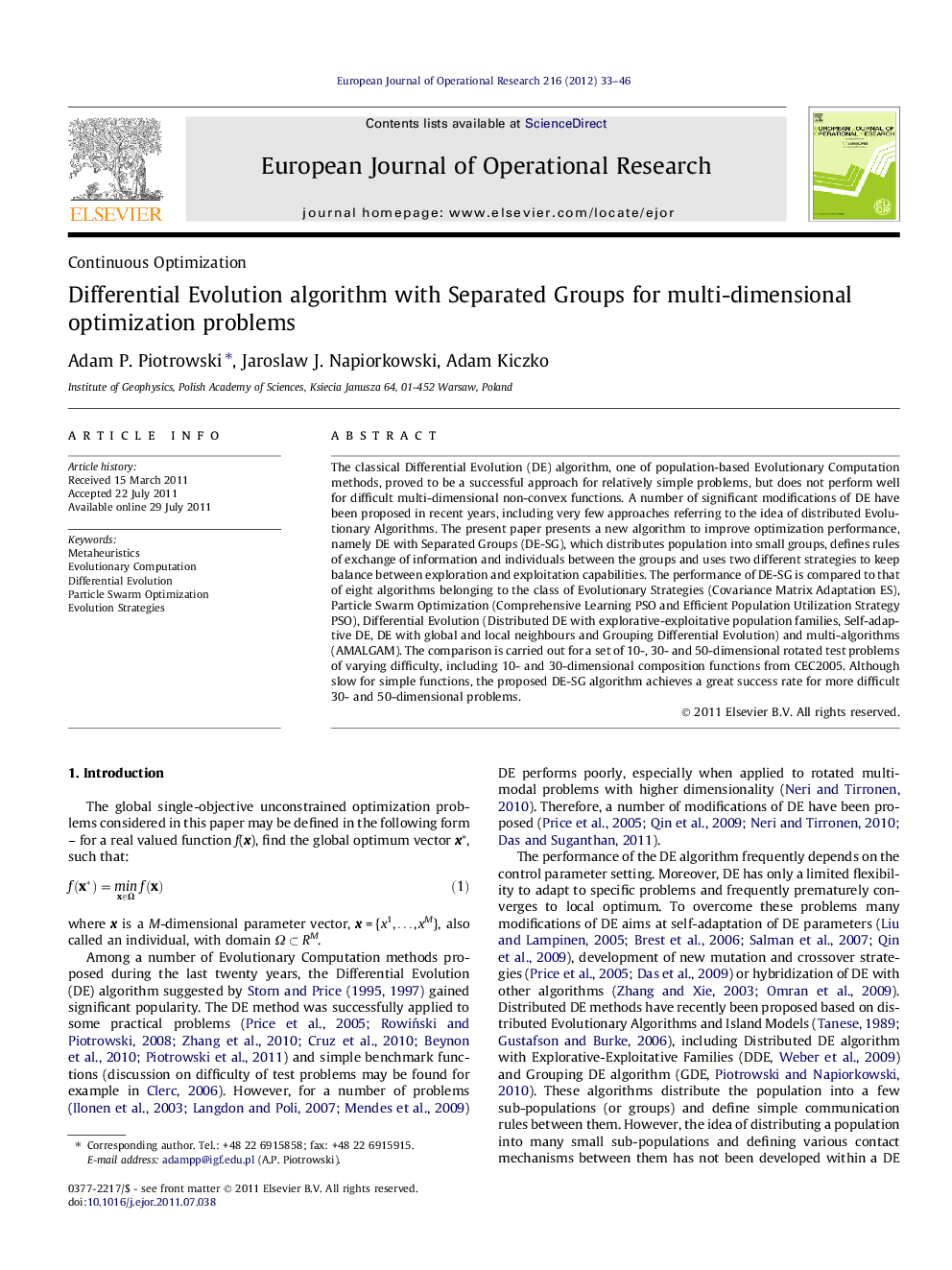
The classical Differential Evolution (DE) algorithm, one of population-based Evolutionary Computation methods, proved to be a successful approach for relatively simple problems, but does not perform well for difficult multi-dimensional non-convex functions. A number of significant modifications of DE have been proposed in recent years, including very few approaches referring to the idea of distributed Evolutionary Algorithms. The present paper presents a new algorithm to improve optimization performance, namely DE with Separated Groups (DE-SG), which distributes population into small groups, defines rules of exchange of information and individuals between the groups and uses two different strategies to keep balance between exploration and exploitation capabilities. The performance of DE-SG is compared to that of eight algorithms belonging to the class of Evolutionary Strategies (Covariance Matrix Adaptation ES), Particle Swarm Optimization (Comprehensive Learning PSO and Efficient Population Utilization Strategy PSO), Differential Evolution (Distributed DE with explorative-exploitative population families, Self-adaptive DE, DE with global and local neighbours and Grouping Differential Evolution) and multi-algorithms (AMALGAM). The comparison is carried out for a set of 10-, 30- and 50-dimensional rotated test problems of varying difficulty, including 10- and 30-dimensional composition functions from CEC2005. Although slow for simple functions, the proposed DE-SG algorithm achieves a great success rate for more difficult 30- and 50-dimensional problems.
► New algorithm based on Differential Evolution and an idea of Island Models is presented.
► New algorithm outperforms several state-of-the-art methods for difficult benchmark problems.
► Among state-of-the-art algorithms CMA-ES and AMALGAM outperform the other methods.
Journal: European Journal of Operational Research - Volume 216, Issue 1, 1 January 2012, Pages 33–46