کد مقاله | کد نشریه | سال انتشار | مقاله انگلیسی | نسخه تمام متن |
---|---|---|---|---|
493951 | 723162 | 2015 | 15 صفحه PDF | دانلود رایگان |
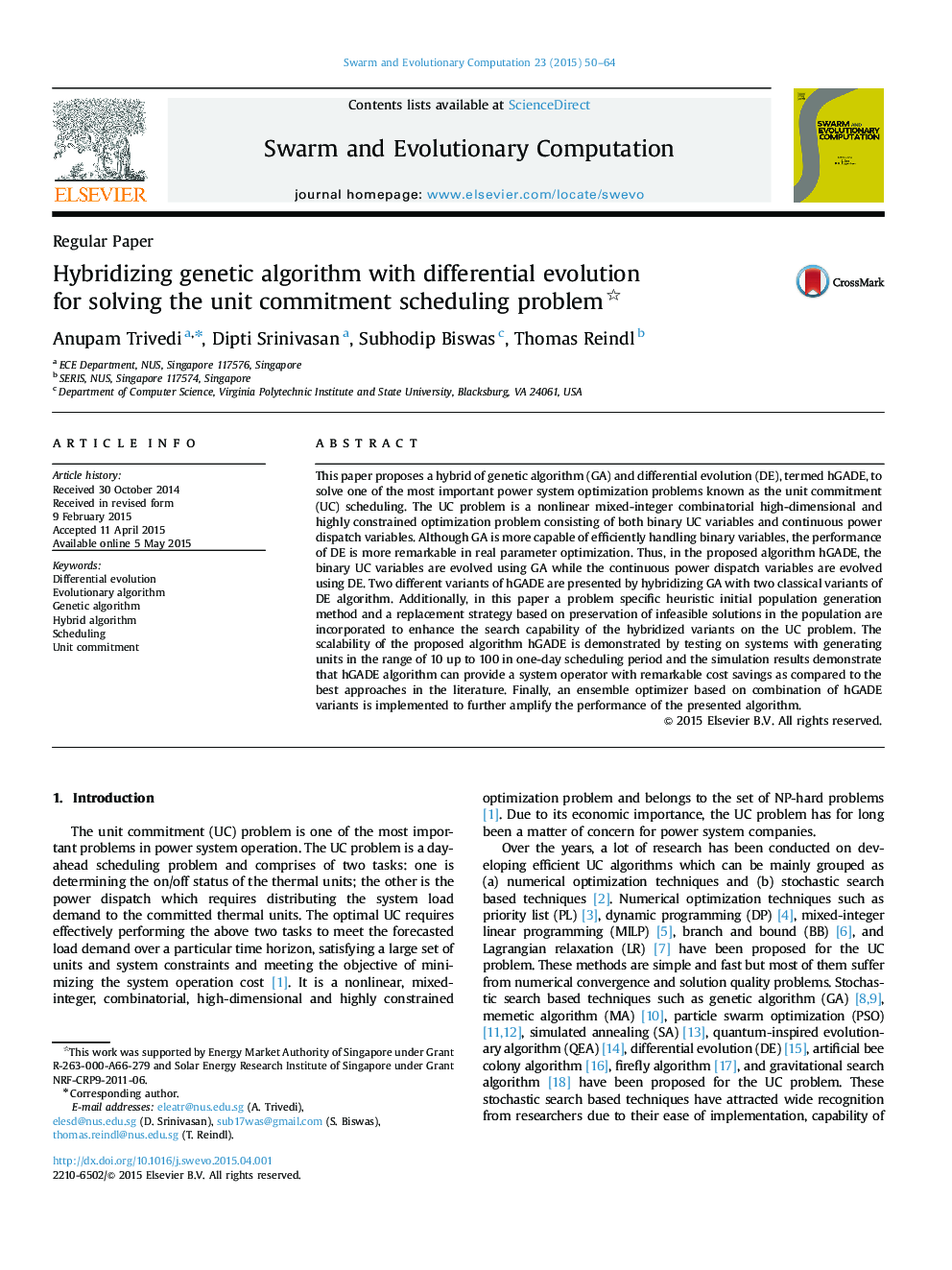
This paper proposes a hybrid of genetic algorithm (GA) and differential evolution (DE), termed hGADE, to solve one of the most important power system optimization problems known as the unit commitment (UC) scheduling. The UC problem is a nonlinear mixed-integer combinatorial high-dimensional and highly constrained optimization problem consisting of both binary UC variables and continuous power dispatch variables. Although GA is more capable of efficiently handling binary variables, the performance of DE is more remarkable in real parameter optimization. Thus, in the proposed algorithm hGADE, the binary UC variables are evolved using GA while the continuous power dispatch variables are evolved using DE. Two different variants of hGADE are presented by hybridizing GA with two classical variants of DE algorithm. Additionally, in this paper a problem specific heuristic initial population generation method and a replacement strategy based on preservation of infeasible solutions in the population are incorporated to enhance the search capability of the hybridized variants on the UC problem. The scalability of the proposed algorithm hGADE is demonstrated by testing on systems with generating units in the range of 10 up to 100 in one-day scheduling period and the simulation results demonstrate that hGADE algorithm can provide a system operator with remarkable cost savings as compared to the best approaches in the literature. Finally, an ensemble optimizer based on combination of hGADE variants is implemented to further amplify the performance of the presented algorithm.
Journal: Swarm and Evolutionary Computation - Volume 23, August 2015, Pages 50–64