کد مقاله | کد نشریه | سال انتشار | مقاله انگلیسی | نسخه تمام متن |
---|---|---|---|---|
4947762 | 1439590 | 2017 | 24 صفحه PDF | دانلود رایگان |
عنوان انگلیسی مقاله ISI
Hypergraph regularized sparse feature learning
دانلود مقاله + سفارش ترجمه
دانلود مقاله ISI انگلیسی
رایگان برای ایرانیان
کلمات کلیدی
موضوعات مرتبط
مهندسی و علوم پایه
مهندسی کامپیوتر
هوش مصنوعی
پیش نمایش صفحه اول مقاله
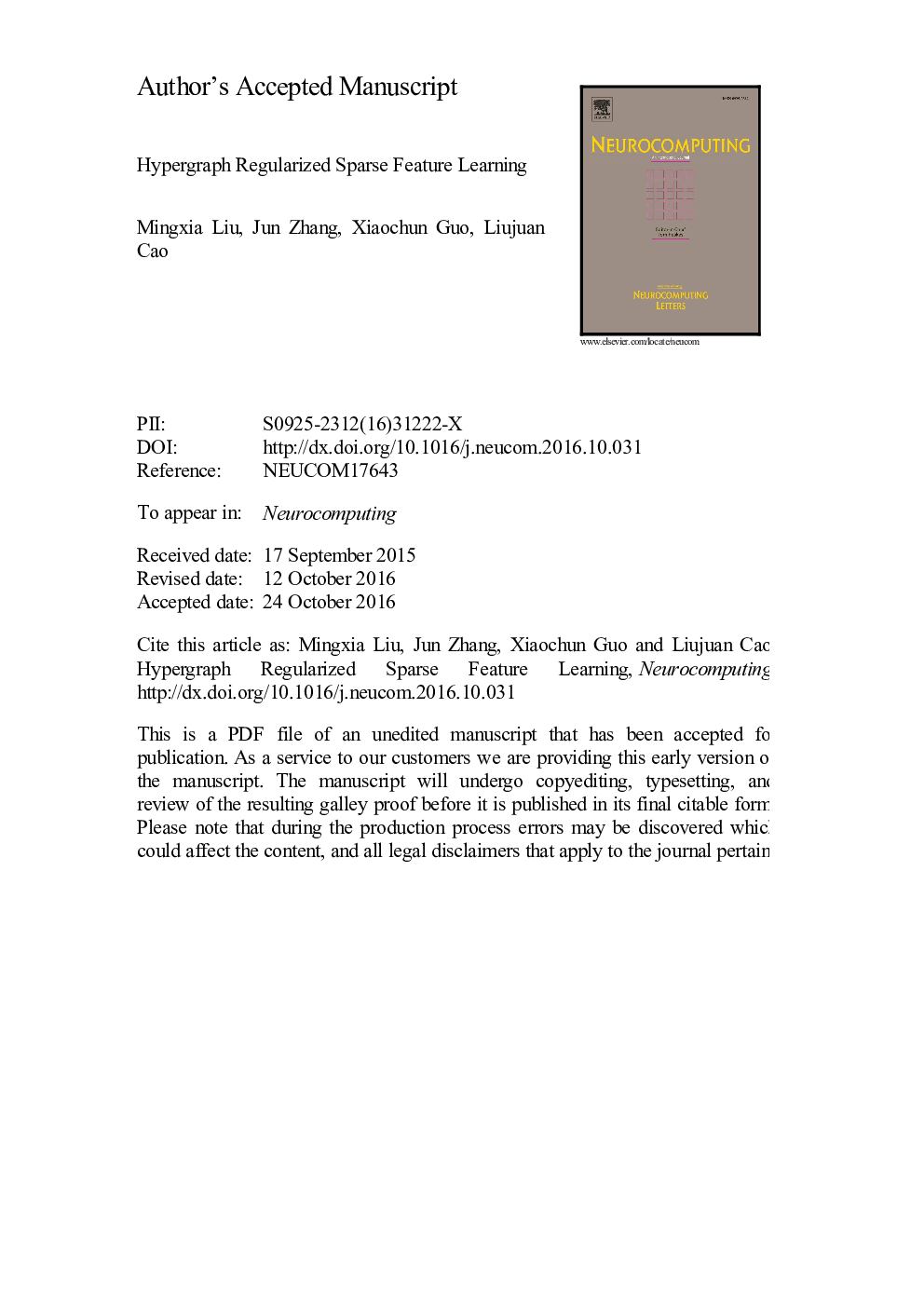
چکیده انگلیسی
As an important pre-processing stage in many machine learning and pattern recognition domains, feature selection deems to identify the most discriminate features for a compact data representation. As typical feature selection methods, Lasso and its variants using the l1-norm based regularization have received much attention in recent years. However, most of existing l1-norm based sparse feature selection methods ignore the structure information of data or only consider the pairwise relationships among samples. In this paper, we propose a hypergraph regularized sparse feature learning method, where the high-order relationships among samples are modeled and incorporated into the learning process. Specifically, we first construct a hypergraph with multiple hyperedges to capture the high-order relationships among samples, followed by the computation of a hypergraph Laplacian matrix. Then, we propose a hypergraph regularization term, and a hypergraph regularized Lasso model. We conduct a series of experiments on a number of data sets from UCI machine learning repository, and two real-world neuroimaging based classification tasks. Experimental results demonstrate that the proposed method achieves promising classification results, compared with several well known feature selection approaches.
ناشر
Database: Elsevier - ScienceDirect (ساینس دایرکت)
Journal: Neurocomputing - Volume 237, 10 May 2017, Pages 185-192
Journal: Neurocomputing - Volume 237, 10 May 2017, Pages 185-192
نویسندگان
Mingxia Liu, Jun Zhang, Xiaochun Guo, Liujuan Cao,