کد مقاله | کد نشریه | سال انتشار | مقاله انگلیسی | نسخه تمام متن |
---|---|---|---|---|
5103176 | 1480096 | 2017 | 17 صفحه PDF | دانلود رایگان |
عنوان انگلیسی مقاله ISI
Multidimensional k-nearest neighbor model based on EEMD for financial time series forecasting
دانلود مقاله + سفارش ترجمه
دانلود مقاله ISI انگلیسی
رایگان برای ایرانیان
کلمات کلیدی
موضوعات مرتبط
مهندسی و علوم پایه
ریاضیات
فیزیک ریاضی
پیش نمایش صفحه اول مقاله
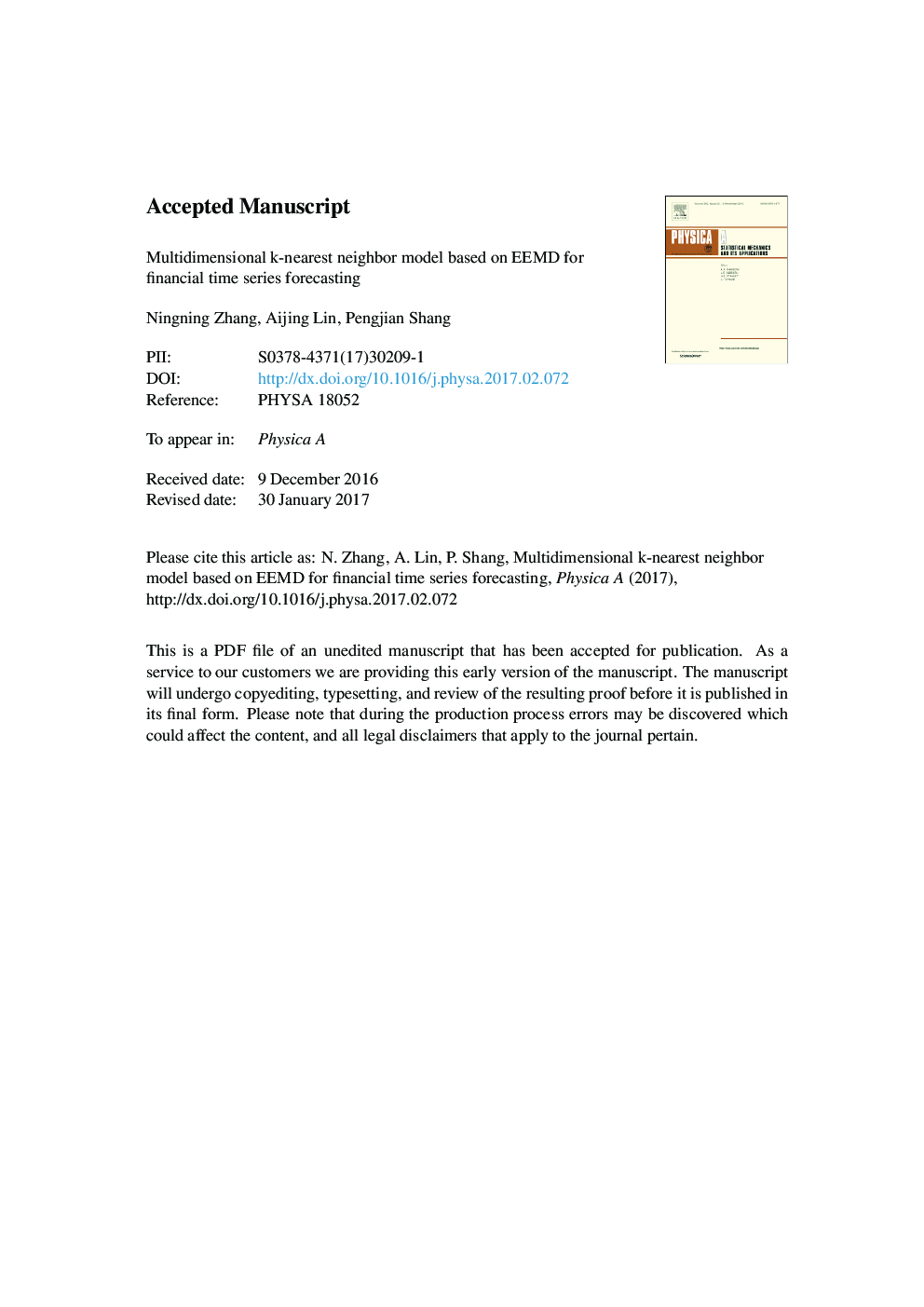
چکیده انگلیسی
In this paper, we propose a new two-stage methodology that combines the ensemble empirical mode decomposition (EEMD) with multidimensional k-nearest neighbor model (MKNN) in order to forecast the closing price and high price of the stocks simultaneously. The modified algorithm of k-nearest neighbors (KNN) has an increasingly wide application in the prediction of all fields. Empirical mode decomposition (EMD) decomposes a nonlinear and non-stationary signal into a series of intrinsic mode functions (IMFs), however, it cannot reveal characteristic information of the signal with much accuracy as a result of mode mixing. So ensemble empirical mode decomposition (EEMD), an improved method of EMD, is presented to resolve the weaknesses of EMD by adding white noise to the original data. With EEMD, the components with true physical meaning can be extracted from the time series. Utilizing the advantage of EEMD and MKNN, the new proposed ensemble empirical mode decomposition combined with multidimensional k-nearest neighbor model (EEMD-MKNN) has high predictive precision for short-term forecasting. Moreover, we extend this methodology to the case of two-dimensions to forecast the closing price and high price of the four stocks (NAS, S&P500, DJI and STI stock indices) at the same time. The results indicate that the proposed EEMD-MKNN model has a higher forecast precision than EMD-KNN, KNN method and ARIMA.
ناشر
Database: Elsevier - ScienceDirect (ساینس دایرکت)
Journal: Physica A: Statistical Mechanics and its Applications - Volume 477, 1 July 2017, Pages 161-173
Journal: Physica A: Statistical Mechanics and its Applications - Volume 477, 1 July 2017, Pages 161-173
نویسندگان
Ningning Zhang, Aijing Lin, Pengjian Shang,