کد مقاله | کد نشریه | سال انتشار | مقاله انگلیسی | نسخه تمام متن |
---|---|---|---|---|
526114 | 869063 | 2011 | 22 صفحه PDF | دانلود رایگان |
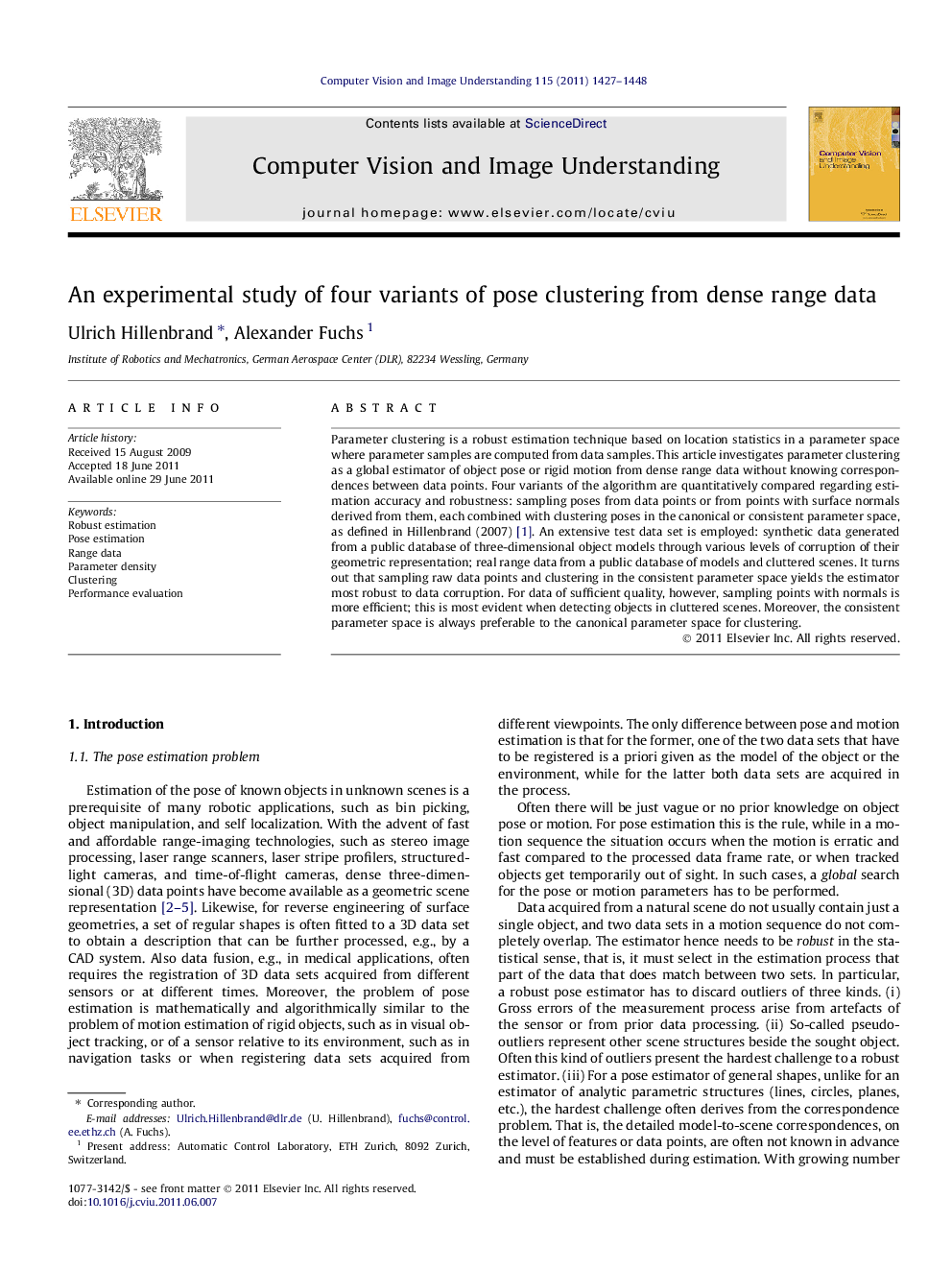
Parameter clustering is a robust estimation technique based on location statistics in a parameter space where parameter samples are computed from data samples. This article investigates parameter clustering as a global estimator of object pose or rigid motion from dense range data without knowing correspondences between data points. Four variants of the algorithm are quantitatively compared regarding estimation accuracy and robustness: sampling poses from data points or from points with surface normals derived from them, each combined with clustering poses in the canonical or consistent parameter space, as defined in Hillenbrand (2007) [1]. An extensive test data set is employed: synthetic data generated from a public database of three-dimensional object models through various levels of corruption of their geometric representation; real range data from a public database of models and cluttered scenes. It turns out that sampling raw data points and clustering in the consistent parameter space yields the estimator most robust to data corruption. For data of sufficient quality, however, sampling points with normals is more efficient; this is most evident when detecting objects in cluttered scenes. Moreover, the consistent parameter space is always preferable to the canonical parameter space for clustering.
► Pose clustering from dense range data without correspondences is studied.
► Variants with sampling point triples and point-with-normal pairs from the data.
► Variants with clustering in canonical parameter space and consistent parameter space.
► At sufficient data quality, sampling point-with-normal pairs is preferable.
► Clustering in consistent parameter space is always preferable.
Journal: Computer Vision and Image Understanding - Volume 115, Issue 10, October 2011, Pages 1427–1448