کد مقاله | کد نشریه | سال انتشار | مقاله انگلیسی | نسخه تمام متن |
---|---|---|---|---|
526146 | 869067 | 2011 | 14 صفحه PDF | دانلود رایگان |
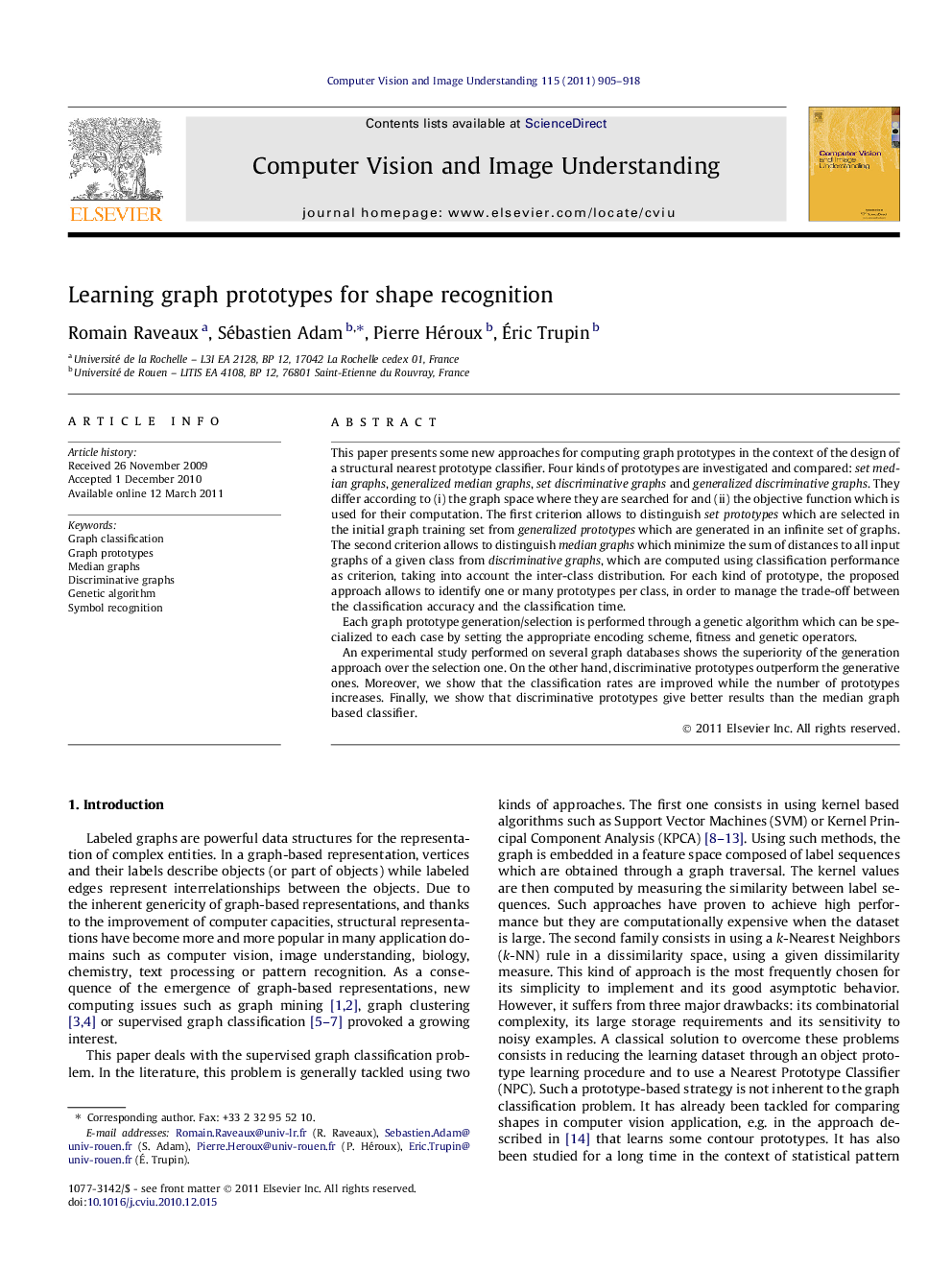
This paper presents some new approaches for computing graph prototypes in the context of the design of a structural nearest prototype classifier. Four kinds of prototypes are investigated and compared: set median graphs, generalized median graphs, set discriminative graphs and generalized discriminative graphs. They differ according to (i) the graph space where they are searched for and (ii) the objective function which is used for their computation. The first criterion allows to distinguish set prototypes which are selected in the initial graph training set from generalized prototypes which are generated in an infinite set of graphs. The second criterion allows to distinguish median graphs which minimize the sum of distances to all input graphs of a given class from discriminative graphs, which are computed using classification performance as criterion, taking into account the inter-class distribution. For each kind of prototype, the proposed approach allows to identify one or many prototypes per class, in order to manage the trade-off between the classification accuracy and the classification time.Each graph prototype generation/selection is performed through a genetic algorithm which can be specialized to each case by setting the appropriate encoding scheme, fitness and genetic operators.An experimental study performed on several graph databases shows the superiority of the generation approach over the selection one. On the other hand, discriminative prototypes outperform the generative ones. Moreover, we show that the classification rates are improved while the number of prototypes increases. Finally, we show that discriminative prototypes give better results than the median graph based classifier.
► The computation of graph prototypes reduces the complexity of graph classification.
► Discriminative prototypes have a better classification power than median graphs.
► Generalized prototypes provide better classification results than set prototypes.
► Dedicated genetic algorithm are efficient for different prototype computation.
► Using many prototypes per class enable to improve classification accuracy
Journal: Computer Vision and Image Understanding - Volume 115, Issue 7, July 2011, Pages 905–918