کد مقاله | کد نشریه | سال انتشار | مقاله انگلیسی | نسخه تمام متن |
---|---|---|---|---|
526198 | 869078 | 2011 | 13 صفحه PDF | دانلود رایگان |
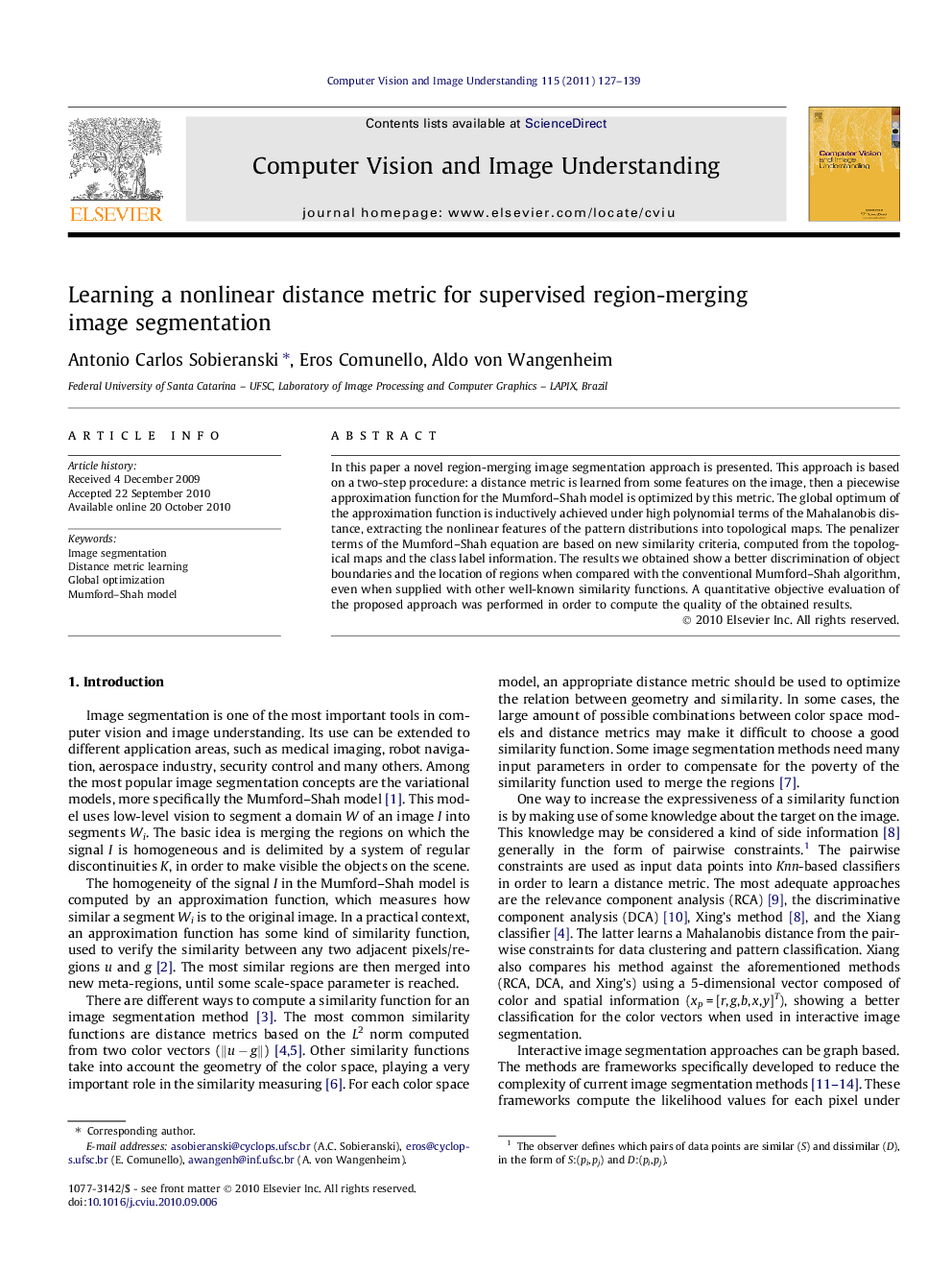
In this paper a novel region-merging image segmentation approach is presented. This approach is based on a two-step procedure: a distance metric is learned from some features on the image, then a piecewise approximation function for the Mumford–Shah model is optimized by this metric. The global optimum of the approximation function is inductively achieved under high polynomial terms of the Mahalanobis distance, extracting the nonlinear features of the pattern distributions into topological maps. The penalizer terms of the Mumford–Shah equation are based on new similarity criteria, computed from the topological maps and the class label information. The results we obtained show a better discrimination of object boundaries and the location of regions when compared with the conventional Mumford–Shah algorithm, even when supplied with other well-known similarity functions. A quantitative objective evaluation of the proposed approach was performed in order to compute the quality of the obtained results.
Research highlights
► Supervised Mumford–Shah approach guides the merging process.
► The observer is able to orient the segmentation result.
► Supervised objective evaluation using ground-truths images.
► Polynomial Mahalanobis distance as clustering method.
Journal: Computer Vision and Image Understanding - Volume 115, Issue 2, February 2011, Pages 127–139