کد مقاله | کد نشریه | سال انتشار | مقاله انگلیسی | نسخه تمام متن |
---|---|---|---|---|
527061 | 869279 | 2006 | 14 صفحه PDF | دانلود رایگان |
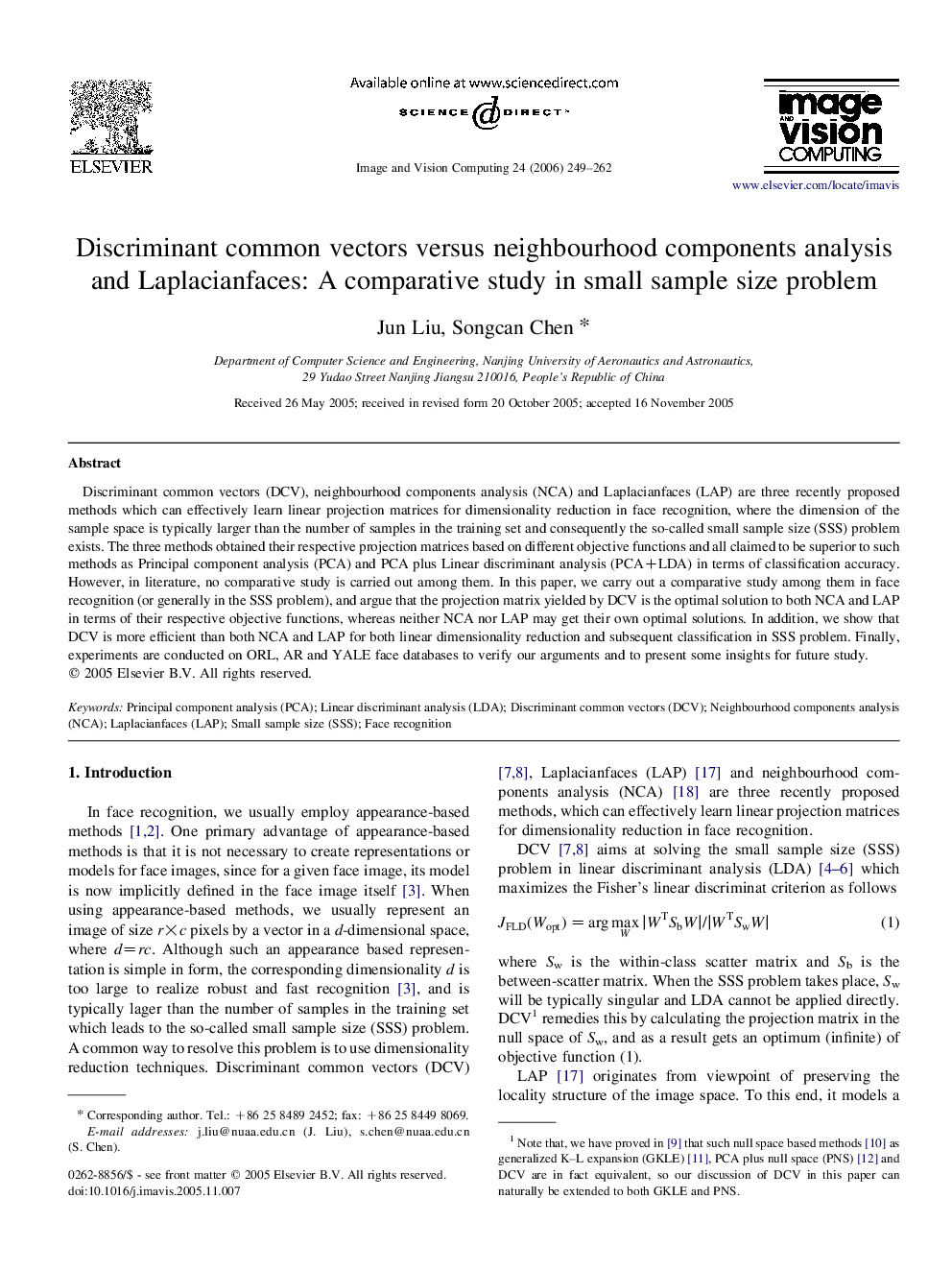
Discriminant common vectors (DCV), neighbourhood components analysis (NCA) and Laplacianfaces (LAP) are three recently proposed methods which can effectively learn linear projection matrices for dimensionality reduction in face recognition, where the dimension of the sample space is typically larger than the number of samples in the training set and consequently the so-called small sample size (SSS) problem exists. The three methods obtained their respective projection matrices based on different objective functions and all claimed to be superior to such methods as Principal component analysis (PCA) and PCA plus Linear discriminant analysis (PCA+LDA) in terms of classification accuracy. However, in literature, no comparative study is carried out among them. In this paper, we carry out a comparative study among them in face recognition (or generally in the SSS problem), and argue that the projection matrix yielded by DCV is the optimal solution to both NCA and LAP in terms of their respective objective functions, whereas neither NCA nor LAP may get their own optimal solutions. In addition, we show that DCV is more efficient than both NCA and LAP for both linear dimensionality reduction and subsequent classification in SSS problem. Finally, experiments are conducted on ORL, AR and YALE face databases to verify our arguments and to present some insights for future study.
Journal: Image and Vision Computing - Volume 24, Issue 3, 1 March 2006, Pages 249–262