کد مقاله | کد نشریه | سال انتشار | مقاله انگلیسی | نسخه تمام متن |
---|---|---|---|---|
528026 | 869486 | 2016 | 11 صفحه PDF | دانلود رایگان |
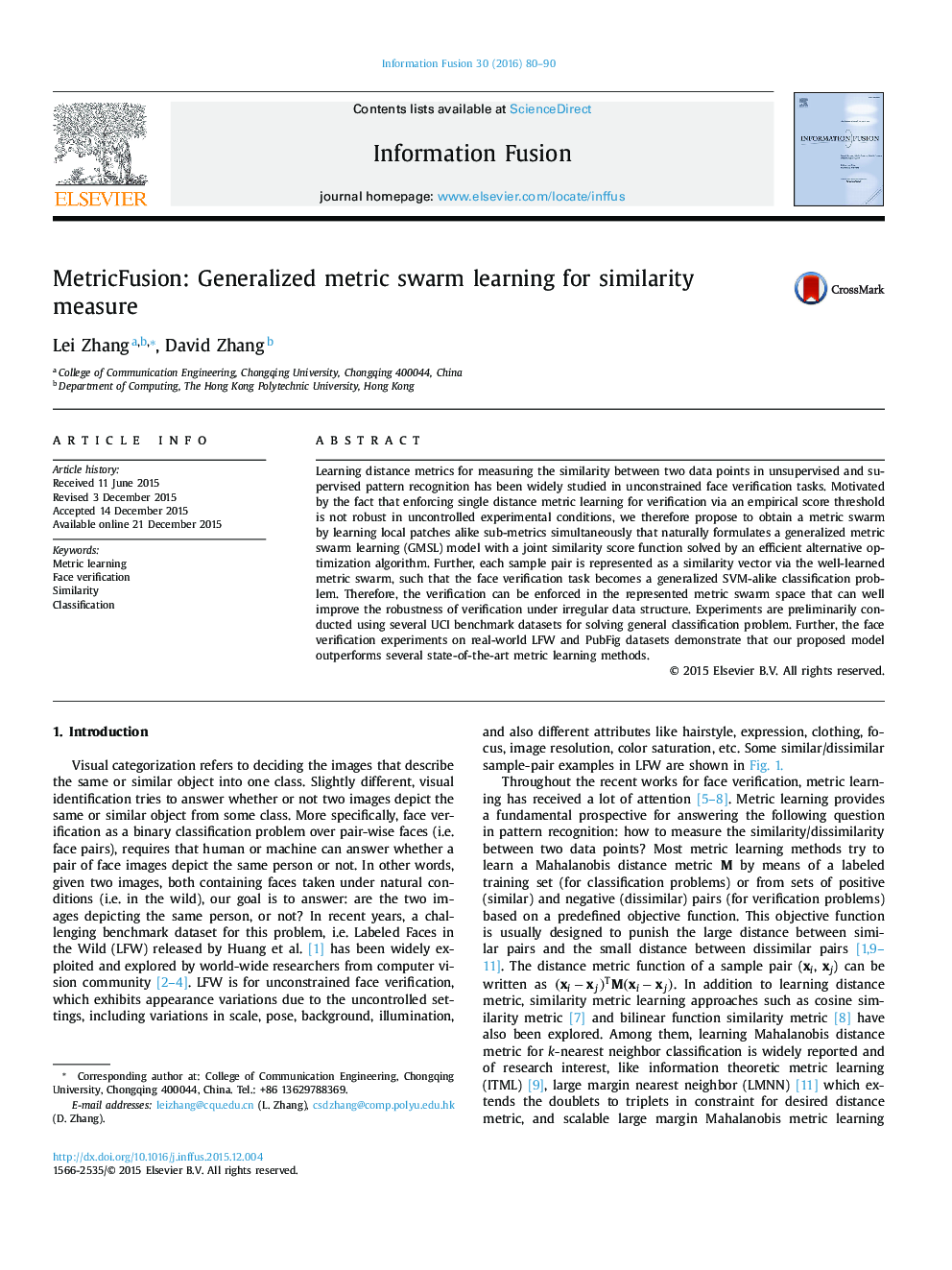
• This paper proposes a similarity measure based on metric fusion and joint similarity score function.
• A generalized metric swarm learning that learns local sub-metrics simultaneously is formulated.
• An efficient alternative optimization algorithm is given for solving the proposed GMSL in dual.
Learning distance metrics for measuring the similarity between two data points in unsupervised and supervised pattern recognition has been widely studied in unconstrained face verification tasks. Motivated by the fact that enforcing single distance metric learning for verification via an empirical score threshold is not robust in uncontrolled experimental conditions, we therefore propose to obtain a metric swarm by learning local patches alike sub-metrics simultaneously that naturally formulates a generalized metric swarm learning (GMSL) model with a joint similarity score function solved by an efficient alternative optimization algorithm. Further, each sample pair is represented as a similarity vector via the well-learned metric swarm, such that the face verification task becomes a generalized SVM-alike classification problem. Therefore, the verification can be enforced in the represented metric swarm space that can well improve the robustness of verification under irregular data structure. Experiments are preliminarily conducted using several UCI benchmark datasets for solving general classification problem. Further, the face verification experiments on real-world LFW and PubFig datasets demonstrate that our proposed model outperforms several state-of-the-art metric learning methods.
Journal: Information Fusion - Volume 30, July 2016, Pages 80–90