کد مقاله | کد نشریه | سال انتشار | مقاله انگلیسی | نسخه تمام متن |
---|---|---|---|---|
569590 | 1452135 | 2015 | 12 صفحه PDF | دانلود رایگان |
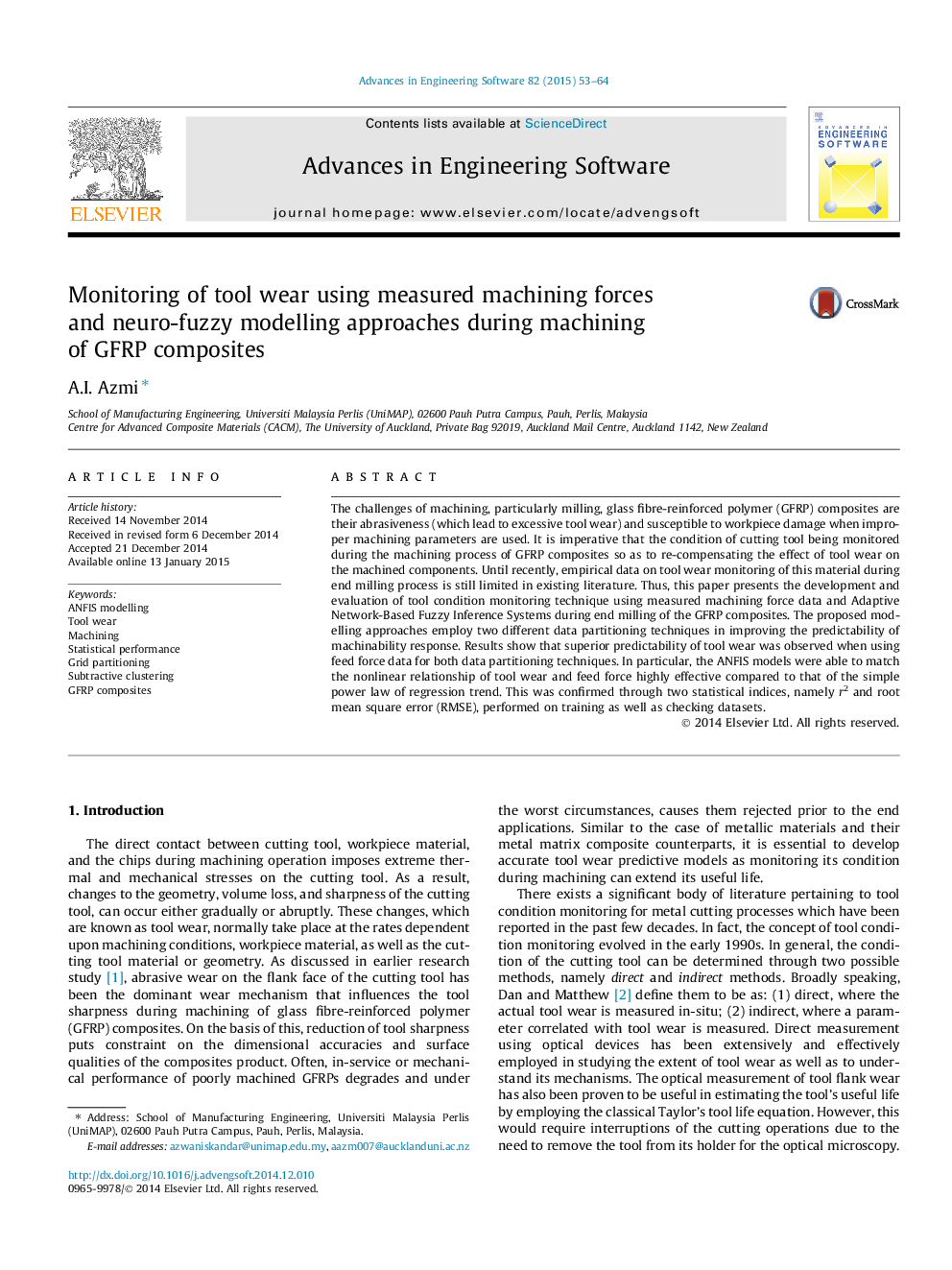
• ANFIS based tool wear/condition monitoring is demonstrated for end milling GFRP composites.
• Grid portioning and subtractive clustering are used to partition the experimental data.
• Using feed force produces better prediction capability compared to cutting force.
• Statistical analyses are used to evaluate the predictive capability.
The challenges of machining, particularly milling, glass fibre-reinforced polymer (GFRP) composites are their abrasiveness (which lead to excessive tool wear) and susceptible to workpiece damage when improper machining parameters are used. It is imperative that the condition of cutting tool being monitored during the machining process of GFRP composites so as to re-compensating the effect of tool wear on the machined components. Until recently, empirical data on tool wear monitoring of this material during end milling process is still limited in existing literature. Thus, this paper presents the development and evaluation of tool condition monitoring technique using measured machining force data and Adaptive Network-Based Fuzzy Inference Systems during end milling of the GFRP composites. The proposed modelling approaches employ two different data partitioning techniques in improving the predictability of machinability response. Results show that superior predictability of tool wear was observed when using feed force data for both data partitioning techniques. In particular, the ANFIS models were able to match the nonlinear relationship of tool wear and feed force highly effective compared to that of the simple power law of regression trend. This was confirmed through two statistical indices, namely r2 and root mean square error (RMSE), performed on training as well as checking datasets.
Journal: Advances in Engineering Software - Volume 82, April 2015, Pages 53–64