کد مقاله | کد نشریه | سال انتشار | مقاله انگلیسی | نسخه تمام متن |
---|---|---|---|---|
569782 | 876690 | 2011 | 12 صفحه PDF | دانلود رایگان |
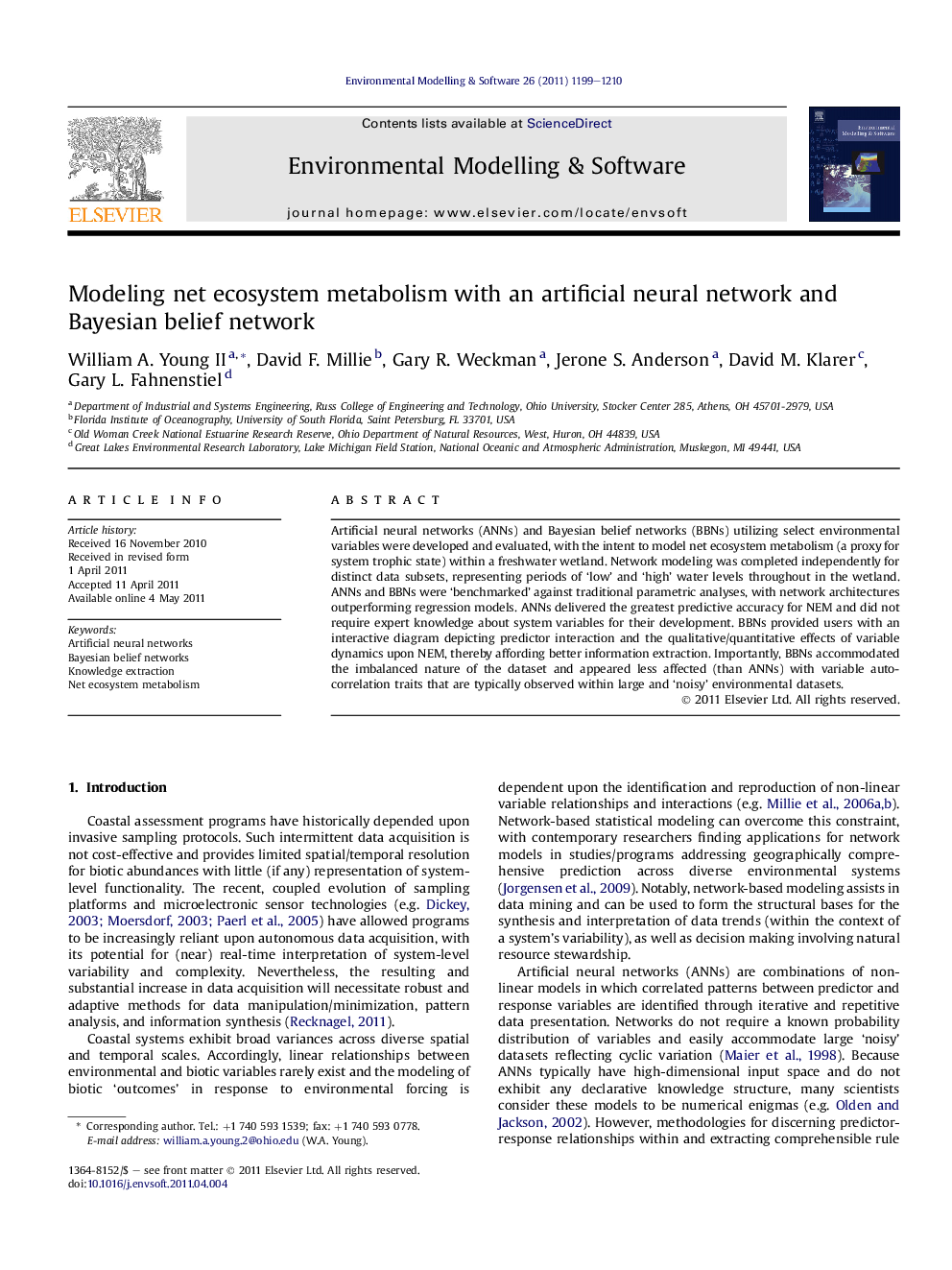
Artificial neural networks (ANNs) and Bayesian belief networks (BBNs) utilizing select environmental variables were developed and evaluated, with the intent to model net ecosystem metabolism (a proxy for system trophic state) within a freshwater wetland. Network modeling was completed independently for distinct data subsets, representing periods of ‘low’ and ‘high’ water levels throughout in the wetland. ANNs and BBNs were ‘benchmarked’ against traditional parametric analyses, with network architectures outperforming regression models. ANNs delivered the greatest predictive accuracy for NEM and did not require expert knowledge about system variables for their development. BBNs provided users with an interactive diagram depicting predictor interaction and the qualitative/quantitative effects of variable dynamics upon NEM, thereby affording better information extraction. Importantly, BBNs accommodated the imbalanced nature of the dataset and appeared less affected (than ANNs) with variable auto-correlation traits that are typically observed within large and ‘noisy’ environmental datasets.
► Mathematical models can be used to analyze an estuary's net ecosystem metabolism (NEM).
► ANNs achieved the highest predictive accuracy and do not require expert knowledge.
► BBNs provide better knowledge extraction solutions but require expert knowledge.
► Results show that it is difficult for a low-water-level estuary to produce oxygen.
► Water flow is a affects NEM significantly and can be controlled by design or land-use policies.
Journal: Environmental Modelling & Software - Volume 26, Issue 10, October 2011, Pages 1199–1210