کد مقاله | کد نشریه | سال انتشار | مقاله انگلیسی | نسخه تمام متن |
---|---|---|---|---|
5768755 | 1628519 | 2017 | 9 صفحه PDF | دانلود رایگان |
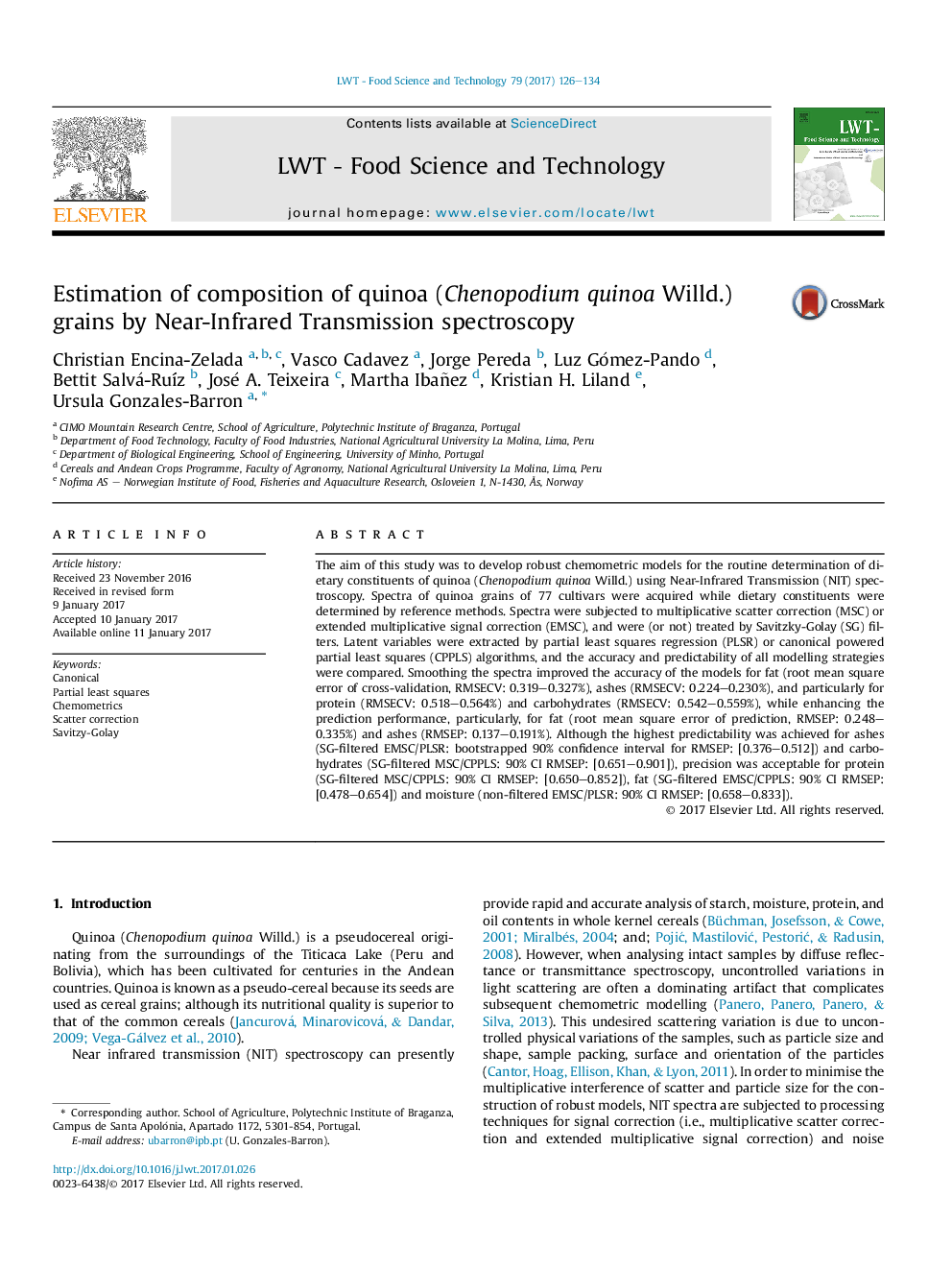
- Near-Infrared Transmission spectra of quinoa grains cultivars were acquired.
- Two methods for multiplicative scatter correction were compared.
- Two multivariate algorithms based on PLS were compared.
- Savitzy-Golay filters improved prediction of dietary constituents.
- NIT spectroscopy can be used for routine analysis of quinoa grains.
The aim of this study was to develop robust chemometric models for the routine determination of dietary constituents of quinoa (Chenopodium quinoa Willd.) using Near-Infrared Transmission (NIT) spectroscopy. Spectra of quinoa grains of 77 cultivars were acquired while dietary constituents were determined by reference methods. Spectra were subjected to multiplicative scatter correction (MSC) or extended multiplicative signal correction (EMSC), and were (or not) treated by Savitzky-Golay (SG) filters. Latent variables were extracted by partial least squares regression (PLSR) or canonical powered partial least squares (CPPLS) algorithms, and the accuracy and predictability of all modelling strategies were compared. Smoothing the spectra improved the accuracy of the models for fat (root mean square error of cross-validation, RMSECV: 0.319-0.327%), ashes (RMSECV: 0.224-0.230%), and particularly for protein (RMSECV: 0.518-0.564%) and carbohydrates (RMSECV: 0.542-0.559%), while enhancing the prediction performance, particularly, for fat (root mean square error of prediction, RMSEP: 0.248-0.335%) and ashes (RMSEP: 0.137-0.191%). Although the highest predictability was achieved for ashes (SG-filtered EMSC/PLSR: bootstrapped 90% confidence interval for RMSEP: [0.376-0.512]) and carbohydrates (SG-filtered MSC/CPPLS: 90% CI RMSEP: [0.651-0.901]), precision was acceptable for protein (SG-filtered MSC/CPPLS: 90% CI RMSEP: [0.650-0.852]), fat (SG-filtered EMSC/CPPLS: 90% CI RMSEP: [0.478-0.654]) and moisture (non-filtered EMSC/PLSR: 90% CI RMSEP: [0.658-0.833]).
Journal: LWT - Food Science and Technology - Volume 79, June 2017, Pages 126-134