کد مقاله | کد نشریه | سال انتشار | مقاله انگلیسی | نسخه تمام متن |
---|---|---|---|---|
5787152 | 1641111 | 2017 | 9 صفحه PDF | دانلود رایگان |
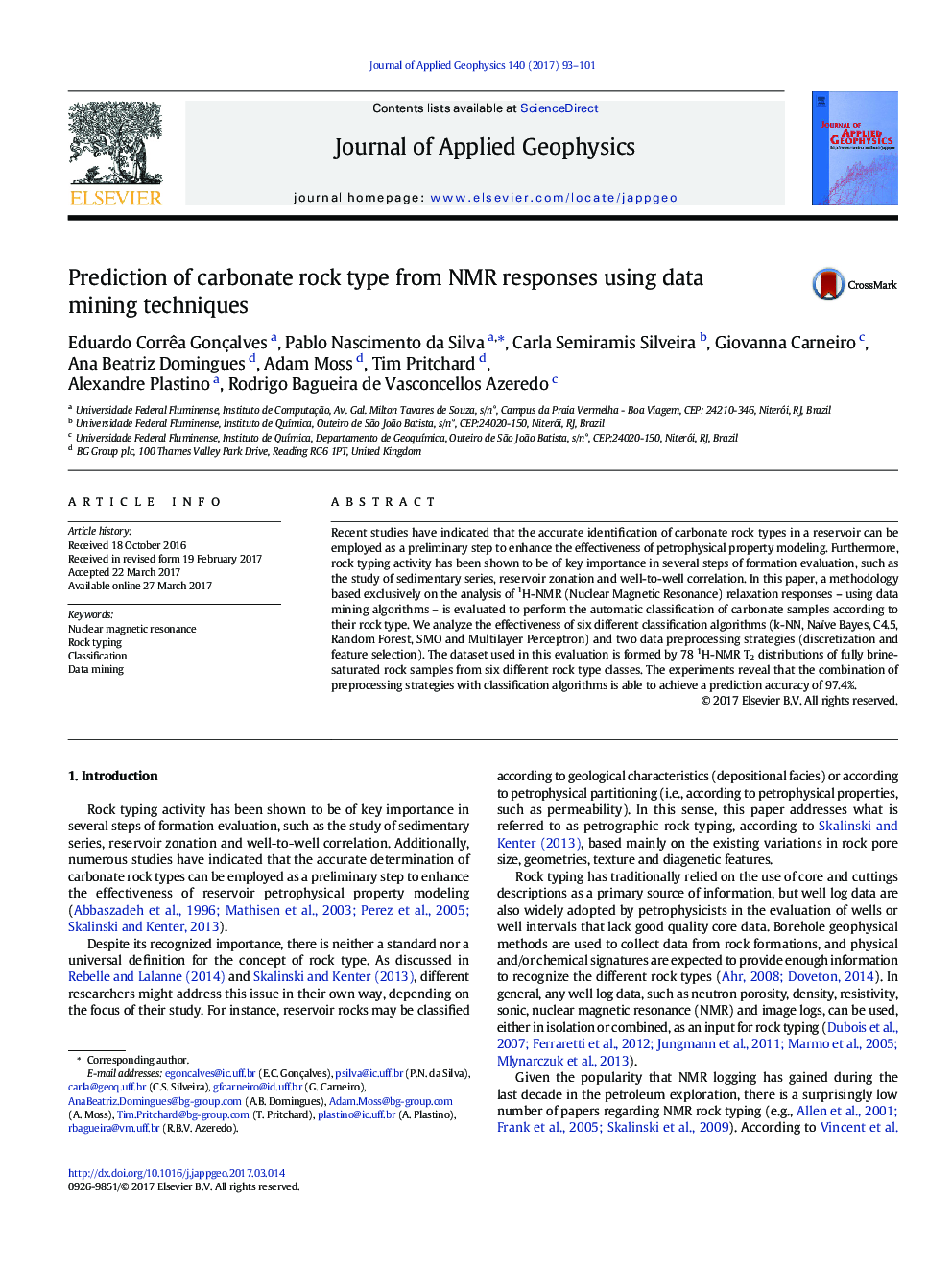
- We have proposed a data mining workflow to perform rock typing.
- The goal is to predict the rock type of carbonate samples using NMR relaxation data.
- We have evaluated the effect of six distinct classifiers in the rock type prediction.
- Discretization and feature selection techniques have improved the results.
- We have achieved an accuracy value of 97.4% for the rock type prediction problem.
Recent studies have indicated that the accurate identification of carbonate rock types in a reservoir can be employed as a preliminary step to enhance the effectiveness of petrophysical property modeling. Furthermore, rock typing activity has been shown to be of key importance in several steps of formation evaluation, such as the study of sedimentary series, reservoir zonation and well-to-well correlation. In this paper, a methodology based exclusively on the analysis of 1H-NMR (Nuclear Magnetic Resonance) relaxation responses - using data mining algorithms - is evaluated to perform the automatic classification of carbonate samples according to their rock type. We analyze the effectiveness of six different classification algorithms (k-NN, Naïve Bayes, C4.5, Random Forest, SMO and Multilayer Perceptron) and two data preprocessing strategies (discretization and feature selection). The dataset used in this evaluation is formed by 78 1H-NMR T2 distributions of fully brine-saturated rock samples from six different rock type classes. The experiments reveal that the combination of preprocessing strategies with classification algorithms is able to achieve a prediction accuracy of 97.4%.
Journal: Journal of Applied Geophysics - Volume 140, May 2017, Pages 93-101