کد مقاله | کد نشریه | سال انتشار | مقاله انگلیسی | نسخه تمام متن |
---|---|---|---|---|
6026965 | 1580908 | 2014 | 12 صفحه PDF | دانلود رایگان |
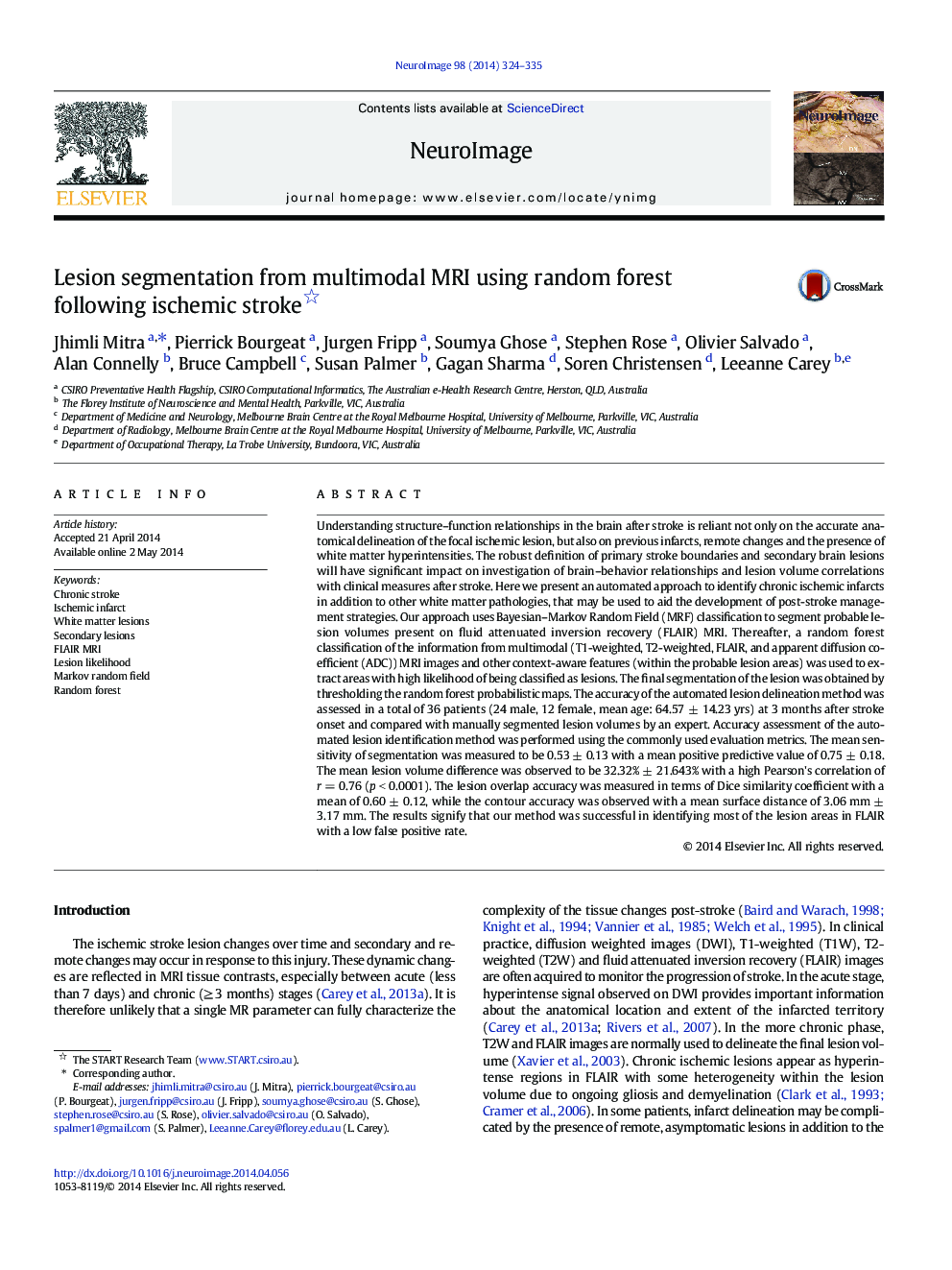
- Segmentation of chronic ischemic and other lesions impacting poststroke depression.
- Hierarchical segmentation of the probable lesion from FLAIR using Bayesian-MRF.
- Random-forest (RF) probabilistic classification on probable lesion class.
- Context-rich features used in RF include multimodal MRI and lesion likelihood.
Understanding structure-function relationships in the brain after stroke is reliant not only on the accurate anatomical delineation of the focal ischemic lesion, but also on previous infarcts, remote changes and the presence of white matter hyperintensities. The robust definition of primary stroke boundaries and secondary brain lesions will have significant impact on investigation of brain-behavior relationships and lesion volume correlations with clinical measures after stroke. Here we present an automated approach to identify chronic ischemic infarcts in addition to other white matter pathologies, that may be used to aid the development of post-stroke management strategies. Our approach uses Bayesian-Markov Random Field (MRF) classification to segment probable lesion volumes present on fluid attenuated inversion recovery (FLAIR) MRI. Thereafter, a random forest classification of the information from multimodal (T1-weighted, T2-weighted, FLAIR, and apparent diffusion coefficient (ADC)) MRI images and other context-aware features (within the probable lesion areas) was used to extract areas with high likelihood of being classified as lesions. The final segmentation of the lesion was obtained by thresholding the random forest probabilistic maps. The accuracy of the automated lesion delineation method was assessed in a total of 36 patients (24 male, 12 female, mean age: 64.57 ± 14.23 yrs) at 3 months after stroke onset and compared with manually segmented lesion volumes by an expert. Accuracy assessment of the automated lesion identification method was performed using the commonly used evaluation metrics. The mean sensitivity of segmentation was measured to be 0.53 ± 0.13 with a mean positive predictive value of 0.75 ± 0.18. The mean lesion volume difference was observed to be 32.32% ± 21.643% with a high Pearson's correlation of r = 0.76 (p < 0.0001). The lesion overlap accuracy was measured in terms of Dice similarity coefficient with a mean of 0.60 ± 0.12, while the contour accuracy was observed with a mean surface distance of 3.06 mm ± 3.17 mm. The results signify that our method was successful in identifying most of the lesion areas in FLAIR with a low false positive rate.
131
Journal: NeuroImage - Volume 98, September 2014, Pages 324-335