کد مقاله | کد نشریه | سال انتشار | مقاله انگلیسی | نسخه تمام متن |
---|---|---|---|---|
6338403 | 1620364 | 2015 | 11 صفحه PDF | دانلود رایگان |
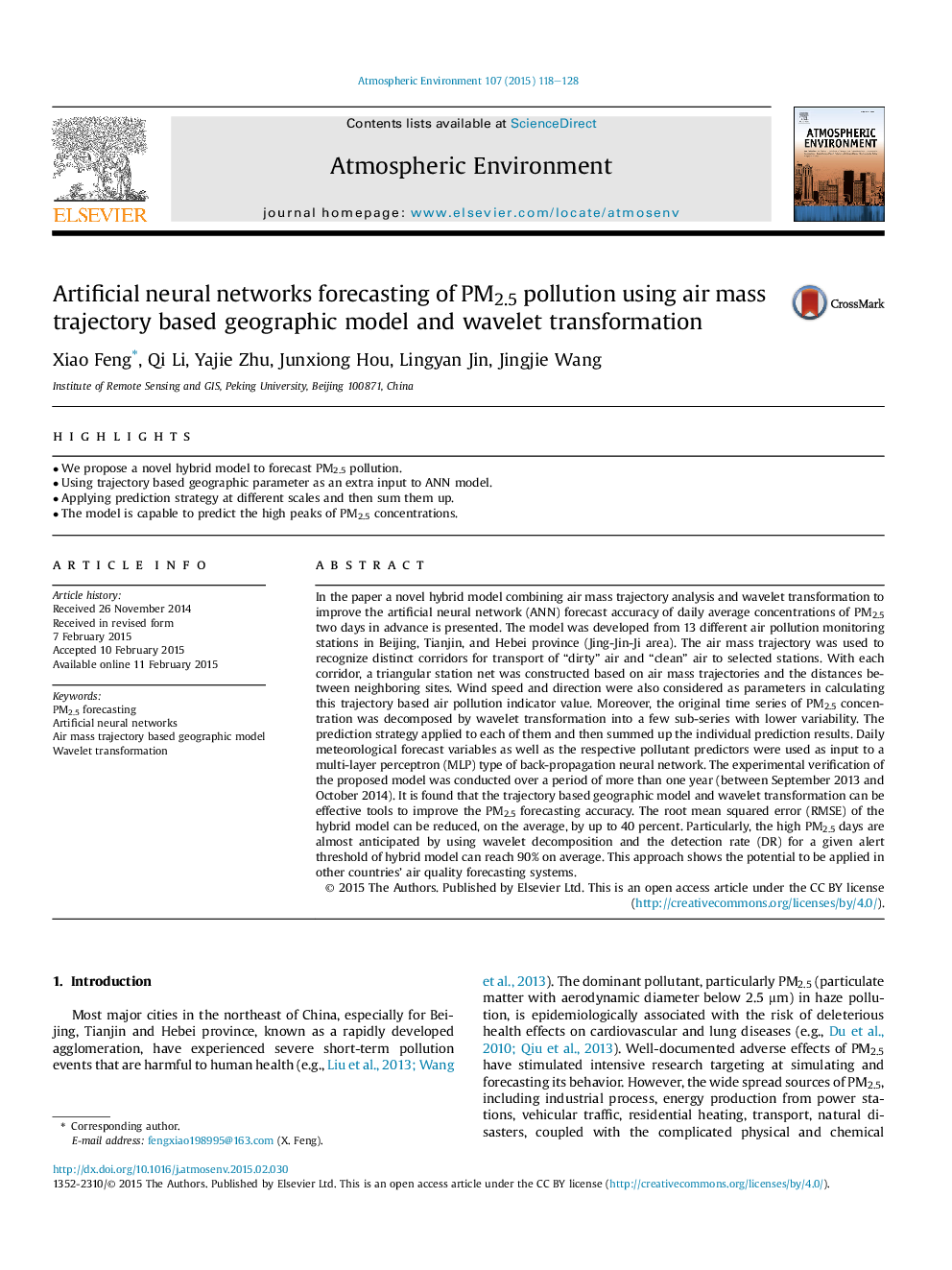
- We propose a novel hybrid model to forecast PM2.5 pollution.
- Using trajectory based geographic parameter as an extra input to ANN model.
- Applying prediction strategy at different scales and then sum them up.
- The model is capable to predict the high peaks of PM2.5 concentrations.
In the paper a novel hybrid model combining air mass trajectory analysis and wavelet transformation to improve the artificial neural network (ANN) forecast accuracy of daily average concentrations of PM2.5 two days in advance is presented. The model was developed from 13 different air pollution monitoring stations in Beijing, Tianjin, and Hebei province (Jing-Jin-Ji area). The air mass trajectory was used to recognize distinct corridors for transport of “dirty” air and “clean” air to selected stations. With each corridor, a triangular station net was constructed based on air mass trajectories and the distances between neighboring sites. Wind speed and direction were also considered as parameters in calculating this trajectory based air pollution indicator value. Moreover, the original time series of PM2.5 concentration was decomposed by wavelet transformation into a few sub-series with lower variability. The prediction strategy applied to each of them and then summed up the individual prediction results. Daily meteorological forecast variables as well as the respective pollutant predictors were used as input to a multi-layer perceptron (MLP) type of back-propagation neural network. The experimental verification of the proposed model was conducted over a period of more than one year (between September 2013 and October 2014). It is found that the trajectory based geographic model and wavelet transformation can be effective tools to improve the PM2.5 forecasting accuracy. The root mean squared error (RMSE) of the hybrid model can be reduced, on the average, by up to 40 percent. Particularly, the high PM2.5 days are almost anticipated by using wavelet decomposition and the detection rate (DR) for a given alert threshold of hybrid model can reach 90% on average. This approach shows the potential to be applied in other countries' air quality forecasting systems.
Journal: Atmospheric Environment - Volume 107, April 2015, Pages 118-128